Risk-Averse Control of Markov Systems with Value Function Learning
arxiv(2023)
摘要
We consider a control problem for a finite-state Markov system whose performance is evaluated by a coherent Markov risk measure. For each policy, the risk of a state is approximated by a function of its features, thus leading to a lower-dimensional policy evaluation problem, which involves non-differentiable stochastic operators. We introduce mini-batch transition risk mappings, which are particularly suited to our approach, and we use them to derive a robust learning algorithm for Markov policy evaluation. Finally, we discuss structured policy improvement in the feature-based risk-averse setting. The considerations are illustrated with an underwater robot navigation problem in which several waypoints must be visited and the observation results must be reported from selected transmission locations. We identify the relevant features, we test the simulation-based learning method, and we optimize a structured policy in a hyperspace containing all problems with the same number of relevant points.
更多查看译文
AI 理解论文
溯源树
样例
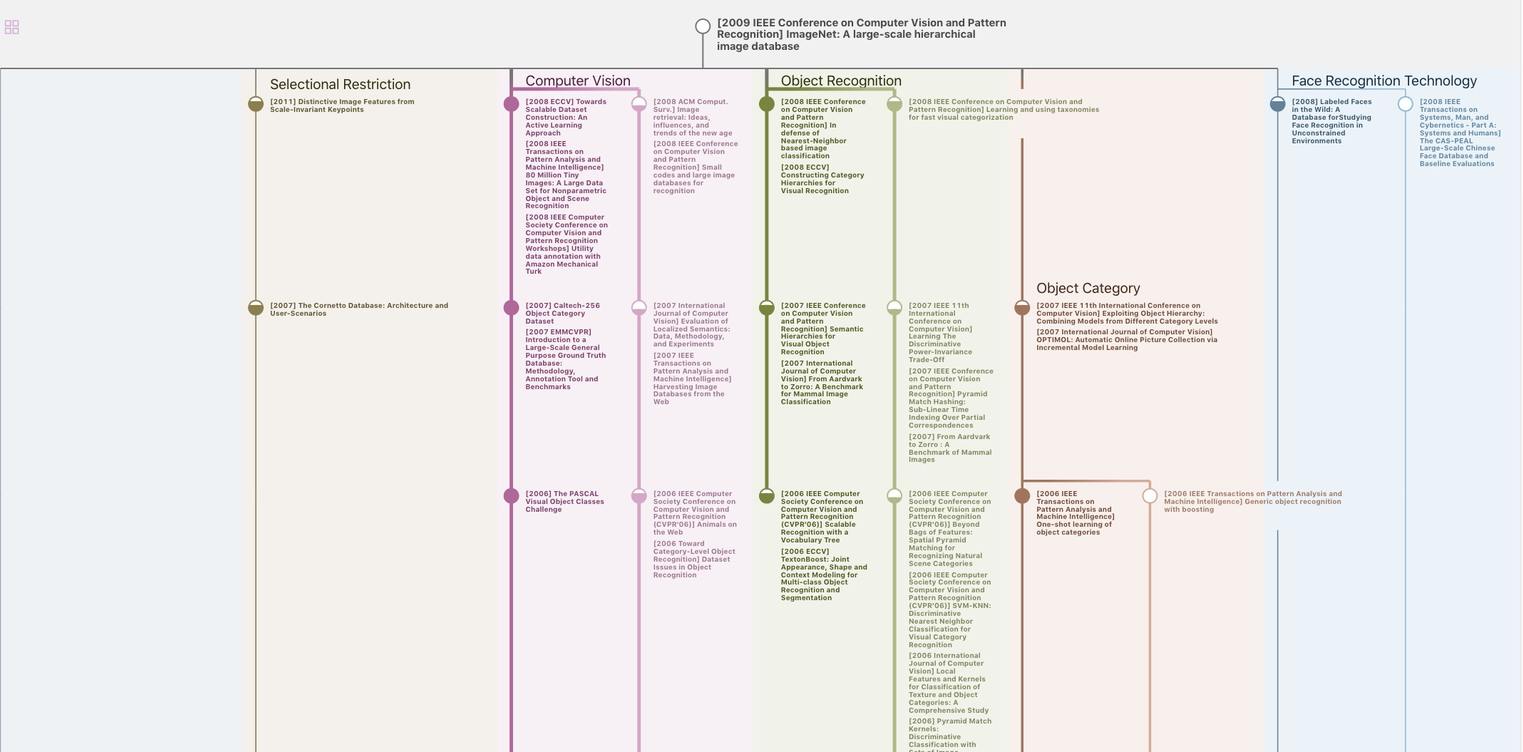
生成溯源树,研究论文发展脉络
Chat Paper
正在生成论文摘要