Robust Computer Vision in an Ever-Changing World: A Survey of Techniques for Tackling Distribution Shifts
CoRR(2023)
摘要
AI applications are becoming increasingly visible to the general public. There is a notable gap between the theoretical assumptions researchers make about computer vision models and the reality those models face when deployed in the real world. One of the critical reasons for this gap is a challenging problem known as distribution shift. Distribution shifts tend to vary with complexity of the data, dataset size, and application type. In our paper, we discuss the identification of such a prominent gap, exploring the concept of distribution shift and its critical significance. We provide an in-depth overview of various types of distribution shifts, elucidate their distinctions, and explore techniques within the realm of the data-centric domain employed to address them. Distribution shifts can occur during every phase of the machine learning pipeline, from the data collection stage to the stage of training a machine learning model to the stage of final model deployment. As a result, it raises concerns about the overall robustness of the machine learning techniques for computer vision applications that are deployed publicly for consumers. Different deep learning models each tailored for specific type of data and tasks, architectural pipelines; highlighting how variations in data preprocessing and feature extraction can impact robustness., data augmentation strategies (e.g. geometric, synthetic and learning-based); demonstrating their role in enhancing model generalization, and training mechanisms (e.g. transfer learning, zero-shot) fall under the umbrella of data-centric methods. Each of these components form an integral part of the neural-network we analyze contributing uniquely to strengthening model robustness against distribution shifts. We compare and contrast numerous AI models that are built for mitigating shifts in hidden stratification and spurious correlations, ...
更多查看译文
AI 理解论文
溯源树
样例
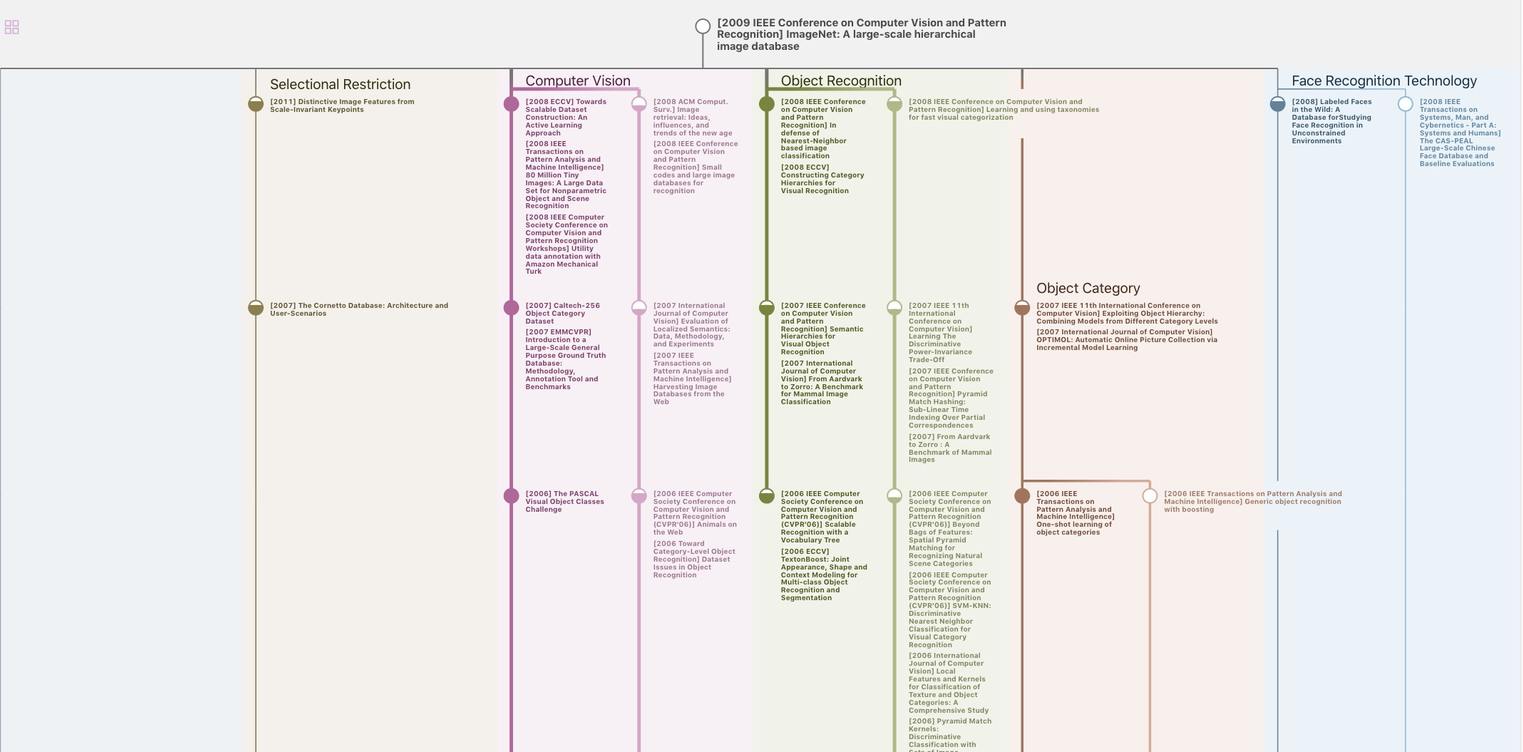
生成溯源树,研究论文发展脉络
Chat Paper
正在生成论文摘要