Enhancing data-limited assessments: Optimal utilization of fishery-dependent data through random effects -- A case study on Korea chub mackerel ($\textit{Scomber japonicus}$)
arxiv(2023)
摘要
In a state-space framework, temporal variations in fishery-dependent processes, such as selectivity and catchability, can be modeled as random effects. This makes state-space models (SSMs) powerful tools for data-limited assessments, especially when conventional CPUE standardization is inapplicable. However, the flexibility of this modeling approach can lead to challenges such as overfitting and parameter non-identifiability. To demonstrate and address these challenges, we developed a state-space length-based age-structured model, which we applied to the Korea chub mackerel ($\textit{Scomber japonicus}$) stock as a case study. The model underwent rigorous scrutiny using various model checking methods to detect potential model mis-specification and non-identifiability under diverse scenarios. Our results demonstrated that incorporating temporal variations in fishery-dependent processes through random effects resolved model mis-specification, but excessive inclusion of random effects rendered the model sensitive to a small number of observations, even when the model was identifiable. For the non-identifiability issue, we employed a non-degenerate estimator, using a gamma distribution as a penalty for the standard deviation (SD) parameters of observation errors. This approach made the SD parameters identifiable and facilitated the simultaneous estimation of both process and observation error variances with minimal bias, known to be a challenging task in SSMs. These findings underscore the importance of model checking in SSMs and emphasize the need for careful consideration of overfitting and non-identifiability when developing such models for data-limited assessments. Additionally, novel assessment results for the mackerel stock were presented, and implications for future stock assessment and management were discussed.
更多查看译文
AI 理解论文
溯源树
样例
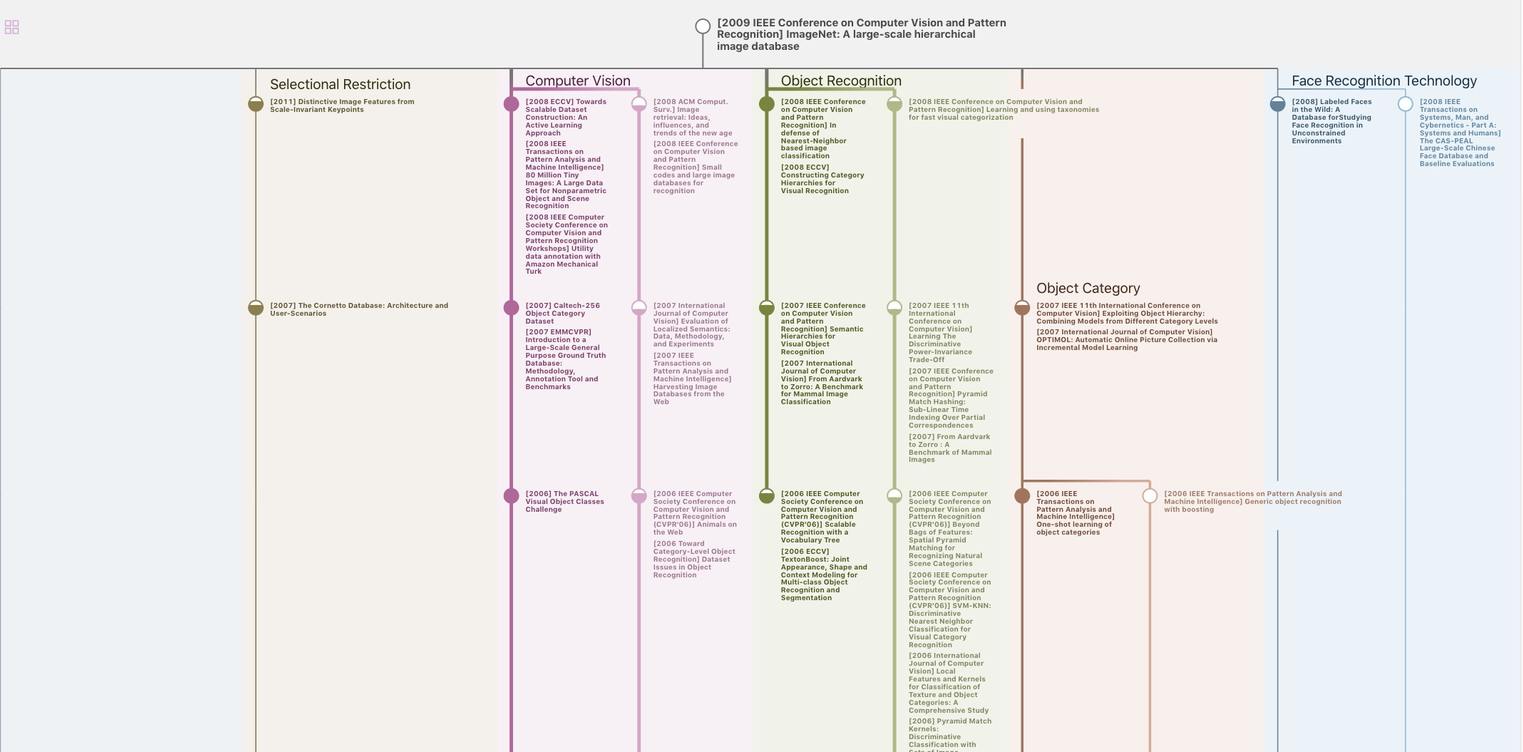
生成溯源树,研究论文发展脉络
Chat Paper
正在生成论文摘要