Prioritizing Remediation of Enterprise Hosts by Malware Execution Risk
39TH ANNUAL COMPUTER SECURITY APPLICATIONS CONFERENCE, ACSAC 2023(2023)
摘要
Defending an enterprise network requires making prioritization decisions daily; one is deciding which compromised hosts to remediate (reimage). We study the utility of endpoint monitoring data to perform this prioritization, with the driving goal being to minimize "regret" as measured by future (next-week) malware execution on hosts whose remediation was deprioritized. Leveraging data gathered by the vendor of a major endpoint protection product, we show that it is possible to prioritize remediation by training a classifier that predicts imminent malware execution. Perhaps surprisingly, while it might seem essential to maximize the amount of training data by collecting across an array of enterprises to which endpoint protection is deployed, at least in the case of the endpoint protection vendor (itself a major, worldwide company), predictive performance for a single enterprise can remain excellent when training is restricted to the enterprise itself. One advantage of single-enterprise training is the ease of combining different views of the hosts, such as via file-based and network-based monitoring. In the cases studied, although an exact comparison was impossible due to a time gap, the single-enterprise dataset with richer features resulted in superior prediction of malware execution compared to the multi-enterprise dataset.
更多查看译文
关键词
malware,remediation
AI 理解论文
溯源树
样例
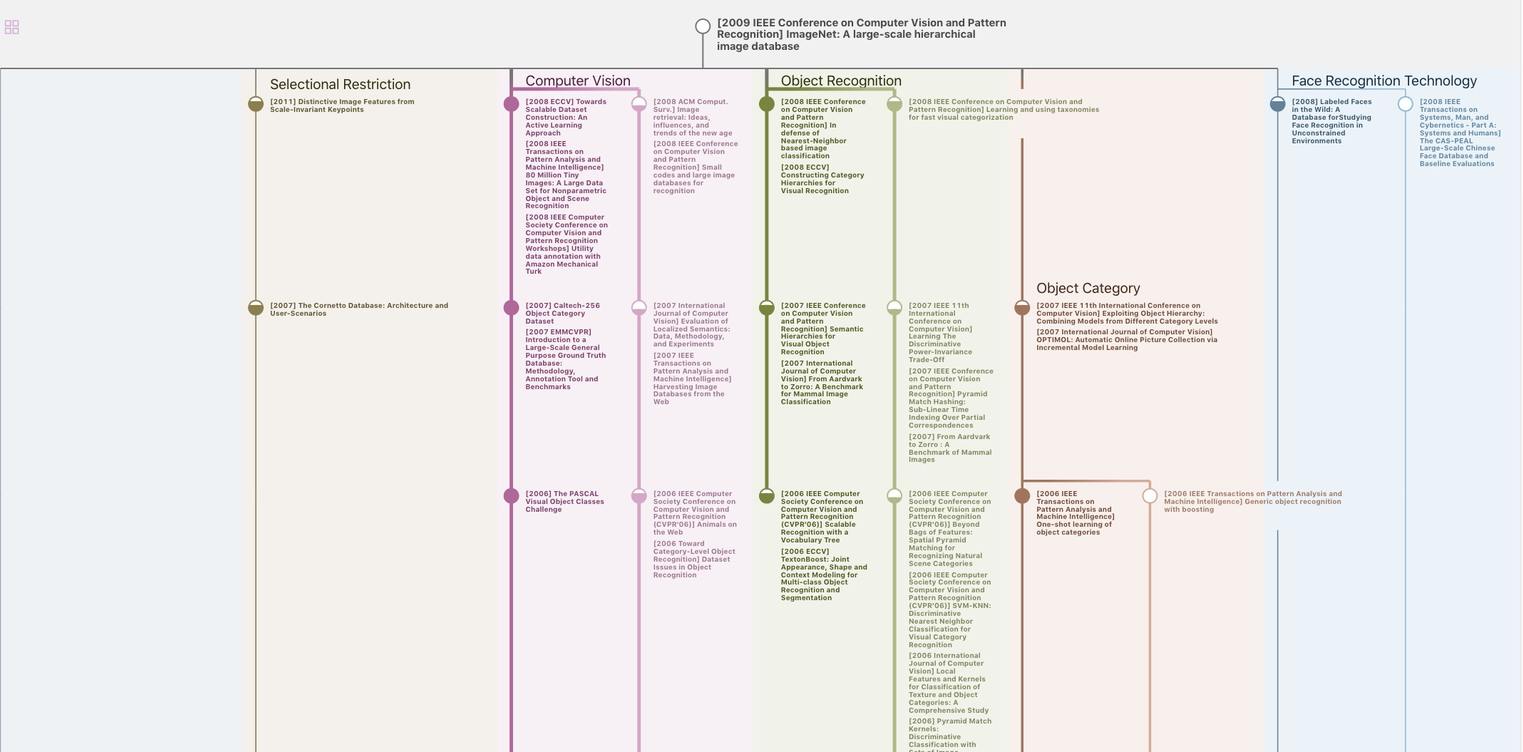
生成溯源树,研究论文发展脉络
Chat Paper
正在生成论文摘要