Longitudinal drug synergy assessment using convolutional neural network image-decoding of glioblastoma single-spheroid cultures
NEURO-ONCOLOGY ADVANCES(2023)
摘要
Background In recent years, drug combinations have become increasingly popular to improve therapeutic outcomes in various diseases, including difficult to cure cancers such as the brain cancer glioblastoma. Assessing the interaction between drugs over time is critical for predicting drug combination effectiveness and minimizing the risk of therapy resistance. However, as viability readouts of drug combination experiments are commonly performed as an endpoint where cells are lysed, longitudinal drug-interaction monitoring is currently only possible through combined endpoint assays.Methods We provide a method for massive parallel monitoring of drug interactions for 16 drug combinations in 3 glioblastoma models over a time frame of 18 days. In our assay, viabilities of single neurospheres are to be estimated based on image information taken at different time points. Neurosphere images taken on the final day (day 18) were matched to the respective viability measured by CellTiter-Glo 3D on the same day. This allowed to use of machine learning to decode image information to viability values on day 18 as well as for the earlier time points (on days 8, 11, and 15).Results Our study shows that neurosphere images allow us to predict cell viability from extrapolated viabilities. This enables to assess of the drug interactions in a time window of 18 days. Our results show a clear and persistent synergistic interaction for several drug combinations over time.Conclusions Our method facilitates longitudinal drug-interaction assessment, providing new insights into the temporal-dynamic effects of drug combinations in 3D neurospheres which can help to identify more effective therapies against glioblastoma.
更多查看译文
关键词
convolutional networks,drug combination,glioblastoma,image processing,synergistic effect
AI 理解论文
溯源树
样例
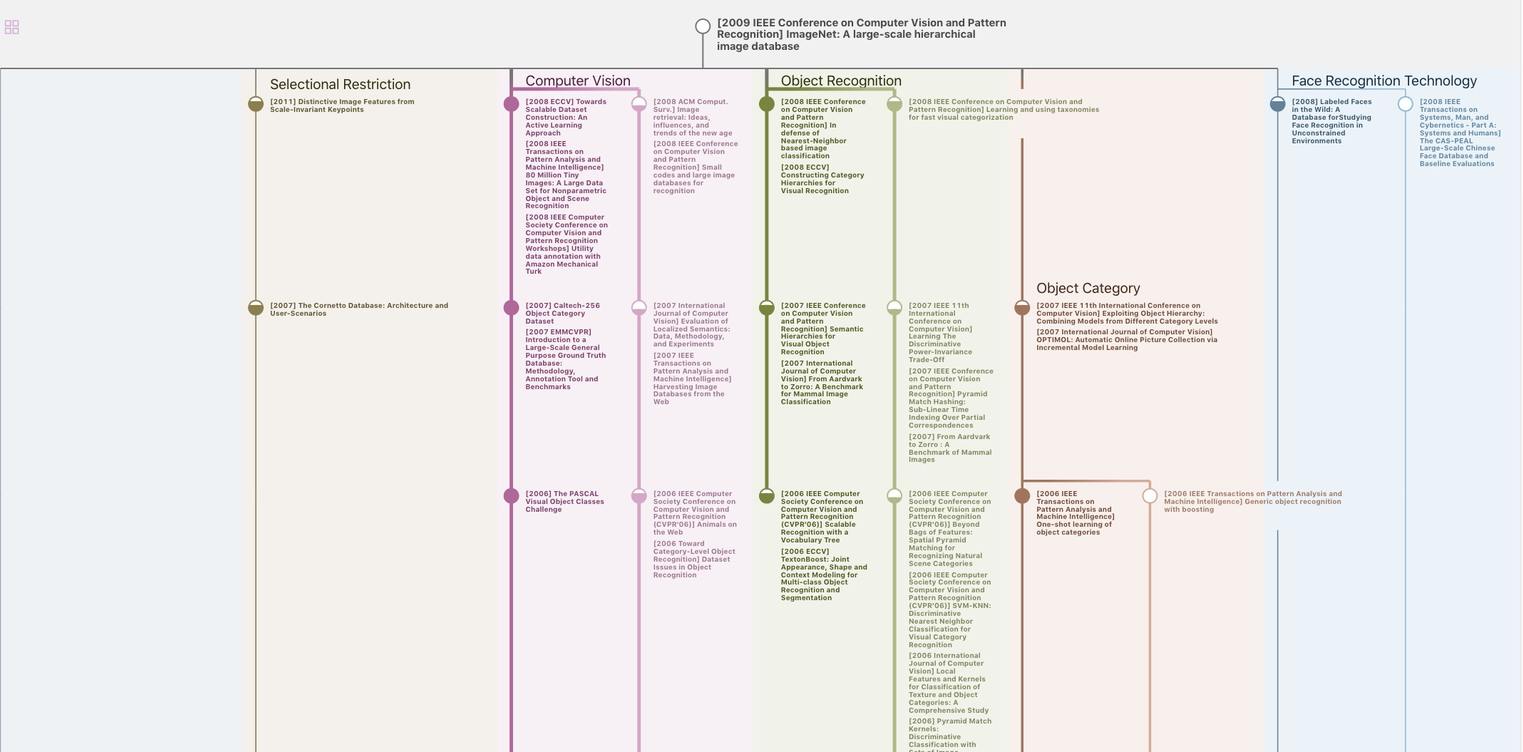
生成溯源树,研究论文发展脉络
Chat Paper
正在生成论文摘要