Detecting Teacher Expertise in an Immersive VR Classroom: Leveraging Fused Sensor Data with Explainable Machine Learning Models
2023 IEEE INTERNATIONAL SYMPOSIUM ON MIXED AND AUGMENTED REALITY, ISMAR(2023)
Abstract
Currently, VR technology is increasingly being used in applications to enable immersive yet controlled research settings. One such area of research is expertise assessment, where novel technological approaches to collecting process data, specifically eye tracking, in combination with explainable models, can provide insights into assessing and training novices, as well as fostering expertise development. We present a machine learning approach to predict teacher expertise by leveraging data from an off-the-shelf VR device collected in a VirATec study. By fusing eye-tracking and controller-tracking data, teachers' recognition and handling of disruptive events in the classroom are taken into account or considered. Three classification models were compared, including SVM, Random Forest, and LightGBM, with Random Forest achieving the best ROC-AUC score of 0.768 in predicting teacher expertise. The SHAP approach to model interpretation revealed informative features (e.g., fixations on identified disruptive students) for distinguishing teacher expertise. Our study serves as a pioneering effort in assessing teacher expertise using eye tracking within an interactive virtual setting, paving the way for future research and advancements in the field.
MoreTranslated text
Key words
Computing methodologies,Machine learning,Machine learning approaches,Classification and regression trees,Human-centered computing,Human computer interaction (HCI),Interaction paradigms,Virtual reality
AI Read Science
Must-Reading Tree
Example
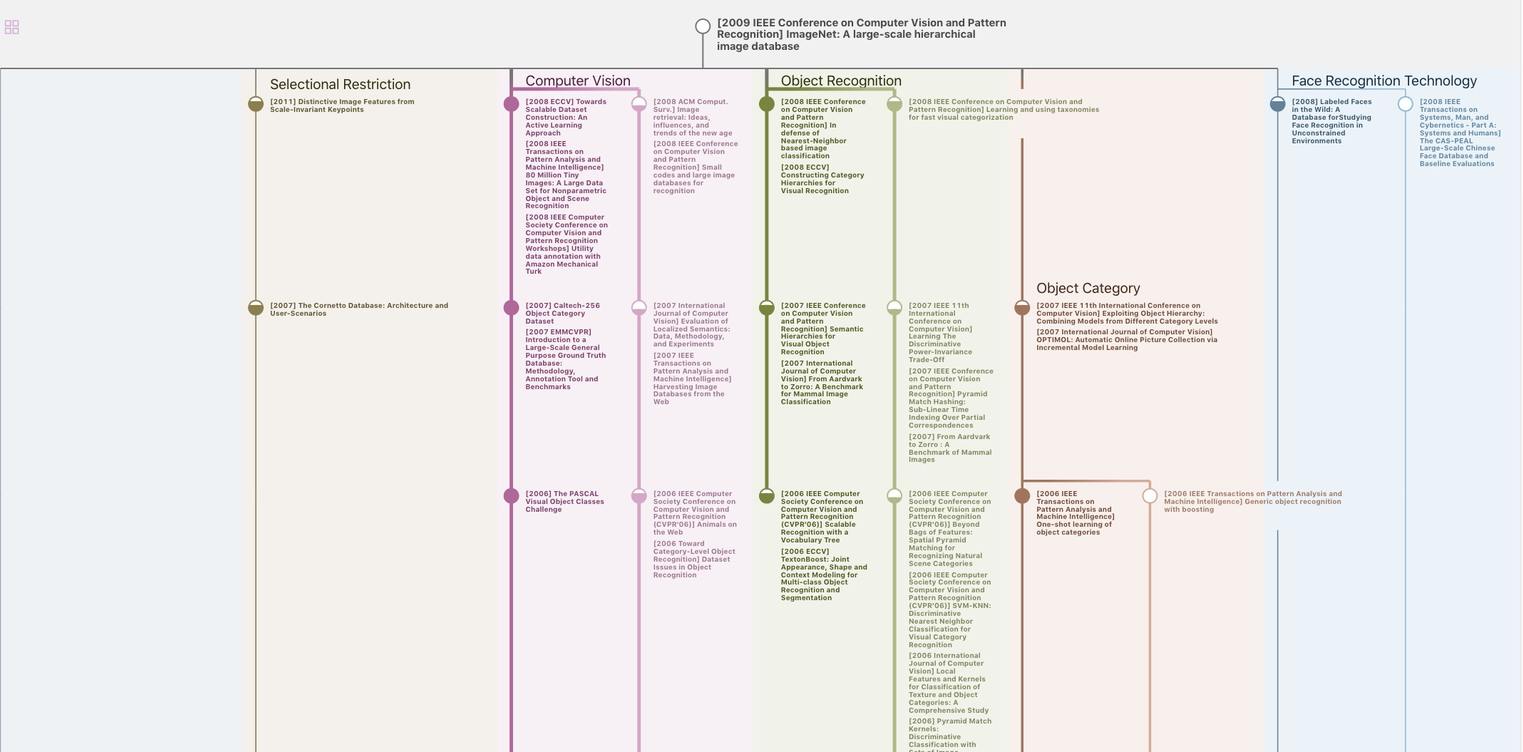
Generate MRT to find the research sequence of this paper
Chat Paper
Summary is being generated by the instructions you defined