SegFormer: Semantic Segmentation Based Tranformers For Corrosion Detection
2023 International Conference on Networking and Advanced Systems (ICNAS)(2023)
摘要
Corrosion detection plays a critical role in various industries for ensuring the safety and durability of structures. Traditional manual inspection methods are time-consuming and prone to human error, necessitating the development of automated techniques. In recent years, artificial intelligence and deep learning have shown promise in corrosion detection. This paper focuses on the evaluation of SegFormer, a pretrained model that combines Transformers with semantic segmentation, for corrosion detection. This paper investigates the application of fine-tuned SegFormer, a transformer-based model, for corrosion detection. Initially, challenges are identified due to class imbalance and limited annotations on the semantic dataset. To address this, a specialized corrosion segmentation dataset is created. The fine-tuned SegFormer model achieves promising results on this dataset, accurately detecting corrosion regions with a test loss of 0.2621, mean accuracy of 0.8139, and mean IoU of 0.7116. This study demonstrates the model's potential for corrosion detection and its significance in advancing semantic segmentation for critical applications.
更多查看译文
关键词
corrosion detection,semantic segmentation,Seg-Former,pretrained model,Transformers,deep-learning,artificial intelligence
AI 理解论文
溯源树
样例
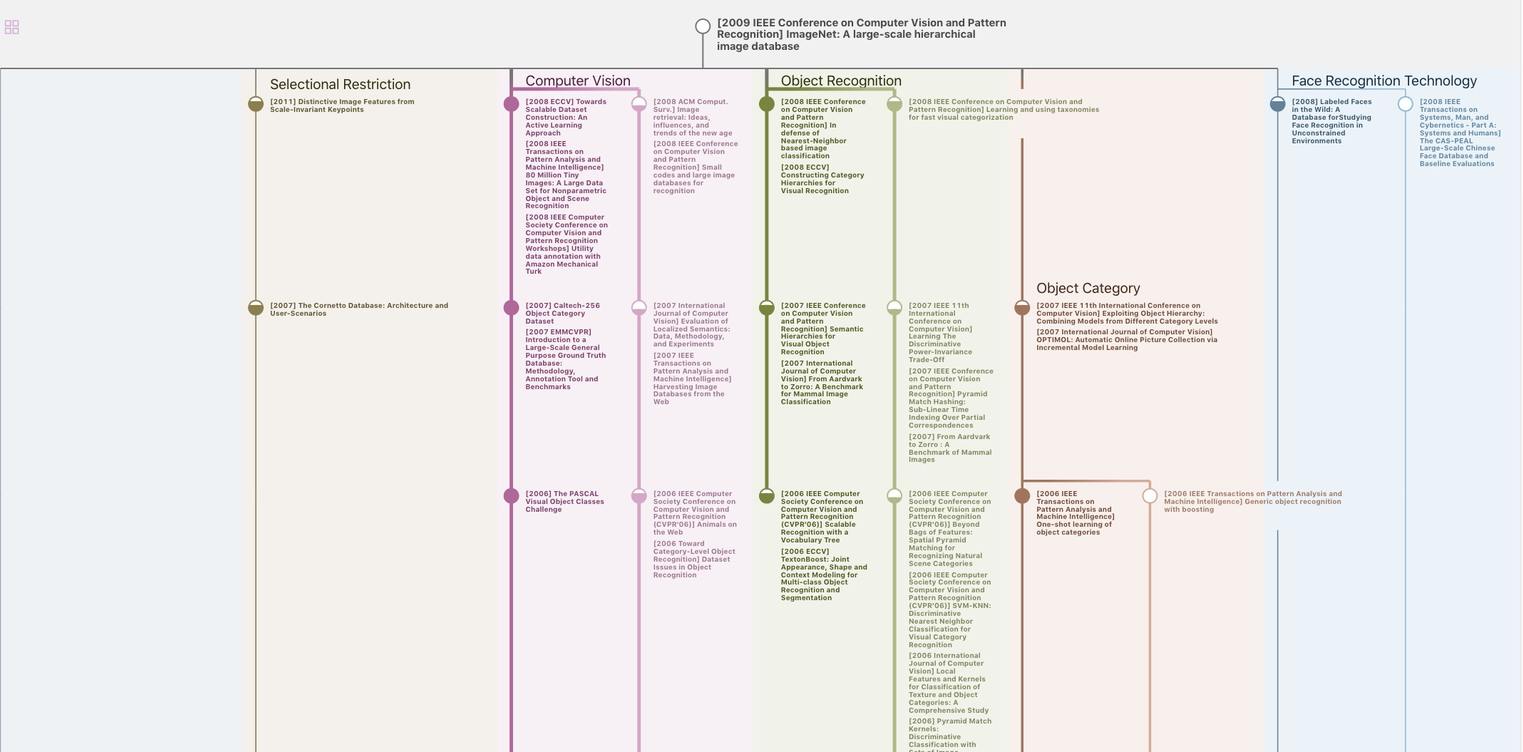
生成溯源树,研究论文发展脉络
Chat Paper
正在生成论文摘要