Light-Weight Deep Learning Models for Acoustic Scene Classification Using Teacher-Student Scheme and Multiple Spectrograms
2023 4TH INTERNATIONAL SYMPOSIUM ON THE INTERNET OF SOUNDS(2023)
摘要
In this paper, we present a light-weight deep learning based system for acoustic scene classification (ASC), which is armed to be integrated into an Internet of Sound (IoS) system with a limitation of hardware resource. To achieve the light-weight ASC model, we develop a teacher-student deep learning scheme with a two-phase training strategy. In the first phase (Phase I), a Teacher network architecture, which shows a large model footprint, is proposed. After training the Teacher, the embeddings, which are the feature map of the teacher, are extracted. In the second phase (Phase II), we propose Students which presents light-weight network architectures. We train the Students with leveraging embeddings extracted from the Teacher. To further improve the accuracy performance, we apply an ensemble of multiple spectrograms on both the Teacher and Students. Our experiments conducted on DCASE 2023 Task 1 dataset with ten target classes ('Airport', 'Bus', 'Metro', 'Metro station', 'Park', 'Public square', 'Shopping mall', 'Street pedestrian', 'Street traffic', 'Tram') helps to achieve the best Student with the accuracy performance of 57.4% on the Development set and 55.6% on the blind Evaluation set, which improve the DCASE baseline by 14.5% and 10.8%, respectively. The best Student also achieves 82.3% with three target classes ('Indoor', 'Outdoor', and 'Transportation') on the Development set and presents a light-weight model of 88.7 KB memory occupation and 29.27 M MACs, which is potential to apply on a wide range of edge devices.
更多查看译文
关键词
Acoustic scene classification,residual-inception architecture,spectrogram,deep neural network
AI 理解论文
溯源树
样例
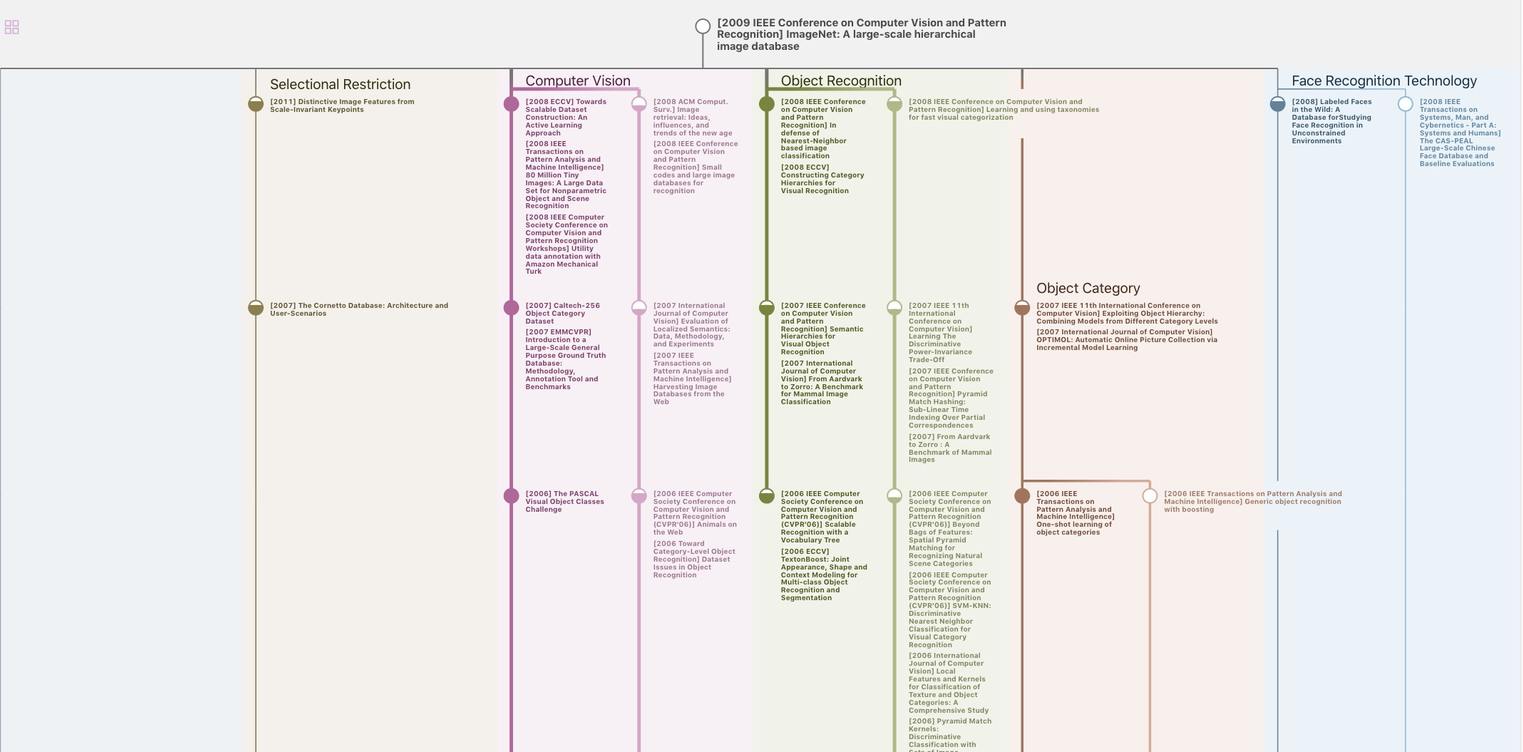
生成溯源树,研究论文发展脉络
Chat Paper
正在生成论文摘要