Improving YOLO-based Object Detection with A Universal Restoration Network in Complex Environments
2023 IEEE 6th International Conference on Pattern Recognition and Artificial Intelligence (PRAI)(2023)
摘要
Object detection based on deep learning has been widely applied in the field and has achieved many achievements, including improving accuracy, real-time performance, and expanding application scope. However, when the environment becomes complex, such as in rainy environments, camera shake leading to blurring, etc., low object detection rate and easily-missed detection problems may occur. This article proposes a YOLO-based object detection scheme to address this issue. The main idea is to restore high-resolution images from their degraded versions before sending them into the network of YOLOv5. To this end, a powerful and universal image restoration network is integrated into the solution to deal with various types of recovery tasks, such as image de-raining, deblurring, and denoising. Experimental results show that the integrated scheme performs better in object detection than the original YOLOv5 algorithm, leading it to a practical application in thepresence of complex interference.
更多查看译文
关键词
Object detection,deep learning,YOLOv5,MPRNet,Complex environment
AI 理解论文
溯源树
样例
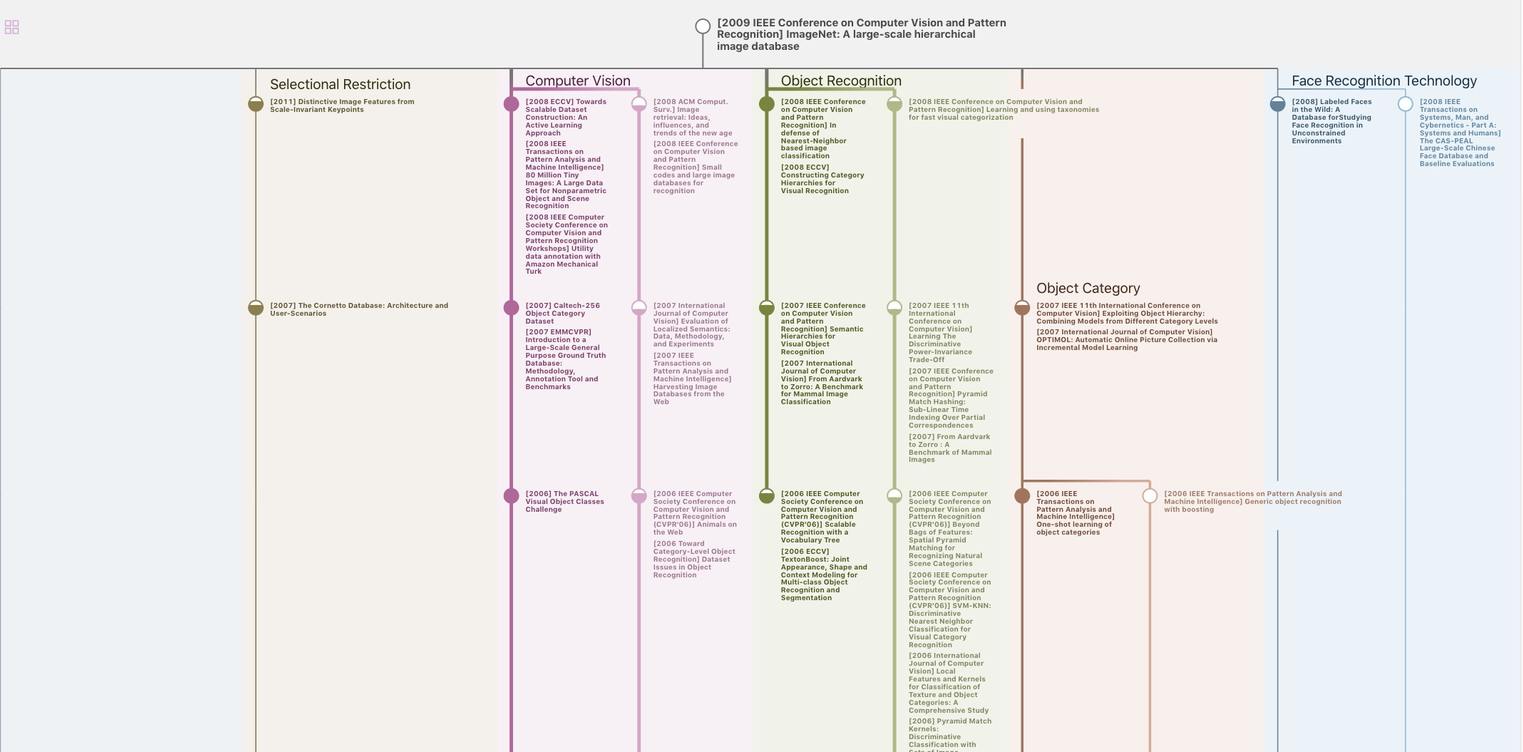
生成溯源树,研究论文发展脉络
Chat Paper
正在生成论文摘要