Affine-invariant Shape Recognition Using Grassmann Manifold
Acta Automatica Sinica(2012)
摘要
Traditional Kendall shape space theory is only applied to similar transform. However, geometric transforms of the object in the imaging process should be represented by affine transform at most situations. We analyze the nonlinear geometry structure of the affine invariant shape space and propose an affine-invariant shape recognition algorithm based on Grassmann manifold geometry. Firstly, we compute the mean shape and covariance for every shape class in the train sets. Then, we construct their norm probability models on the tangent space at each mean shape. Finally, we compute the maximum likelihood class according to the measured object and prior learned shape models. We use the proposed algorithm to recognize shapes in standard shape dataset and real images. Experiment results on MPEG-7 shape dataset show that our recognition algorithm outperforms the algorithm based on Procrustean metric in traditional Kendall shape space theory. Experiment results on real images also show that the proposed algorithm exhibits higher capacity to affine transform than the Procrustean metric based algorithm and can recognize object classes with higher posterior probability.
更多查看译文
关键词
grassmann,recognition,shape,affine-invariant
AI 理解论文
溯源树
样例
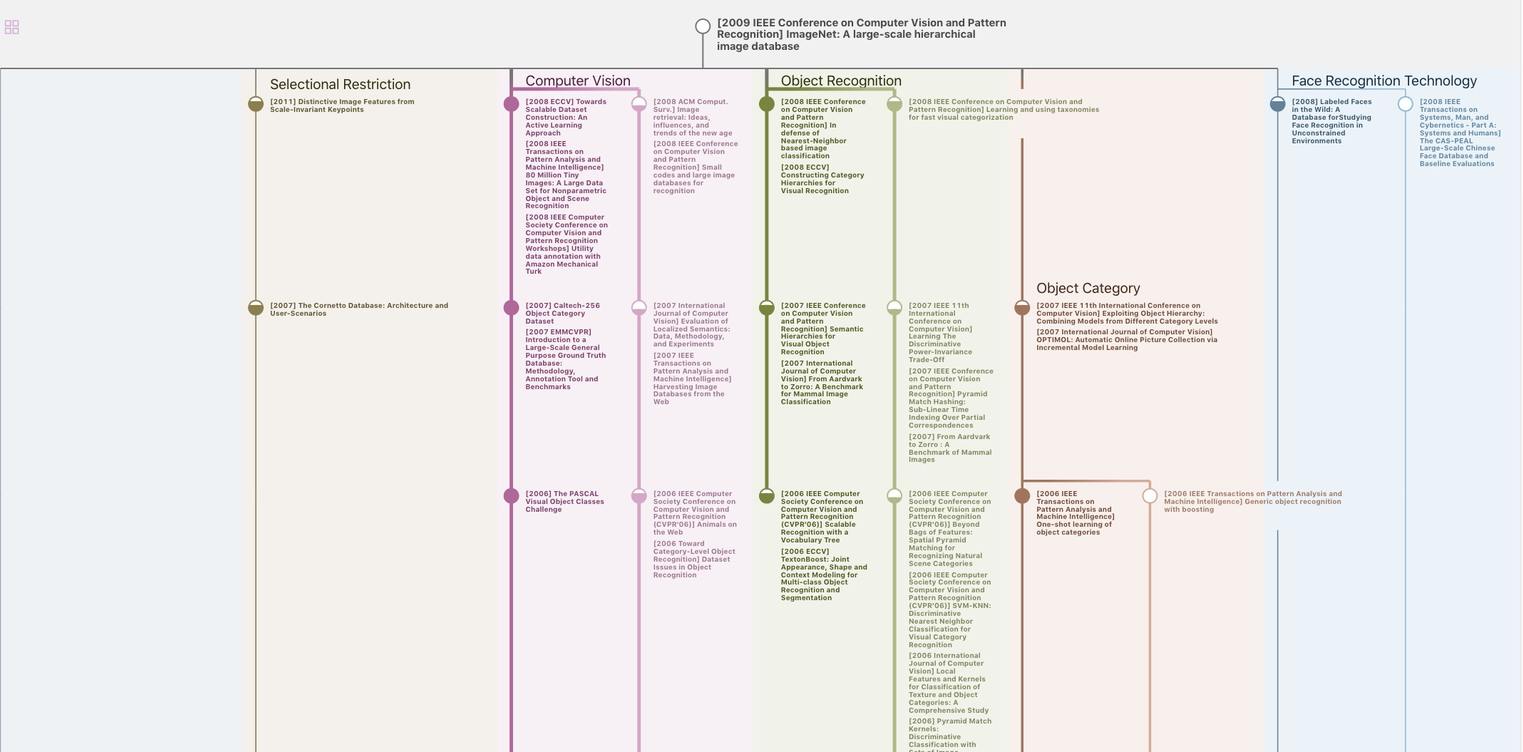
生成溯源树,研究论文发展脉络
Chat Paper
正在生成论文摘要