Learning Erd\H{o}s-R\'enyi Random Graphs via Edge Detecting Queries
arXiv (Cornell University)(2019)
摘要
In this paper, we consider the problem of learning an unknown graph via queries on groups of nodes, with the result indicating whether or not at least one edge is present among those nodes. While learning arbitrary graphs with $n$ nodes and $k$ edges is known to be hard in the sense of requiring $\Omega( \min\{ k^2 \log n, n^2\})$ tests (even when a small probability of error is allowed), we show that learning an Erdős-Renyi random graph with an average of $\bar{k}$ edges is much easier; namely, one can attain asymptotically vanishing error probability with only $O(\bar{k}\log n)$ tests. We establish such bounds for a variety of algorithms inspired by the group testing problem, with explicit constant factors indicating a near-optimal number of tests, and in some cases asymptotic optimality including constant factors. In addition, we present an alternative design that permits a near-optimal sublinear decoding time of $O(\bar{k} \log^2 \bar{k} + \bar{k} \log n)$.
更多查看译文
关键词
graphs,edge,learning
AI 理解论文
溯源树
样例
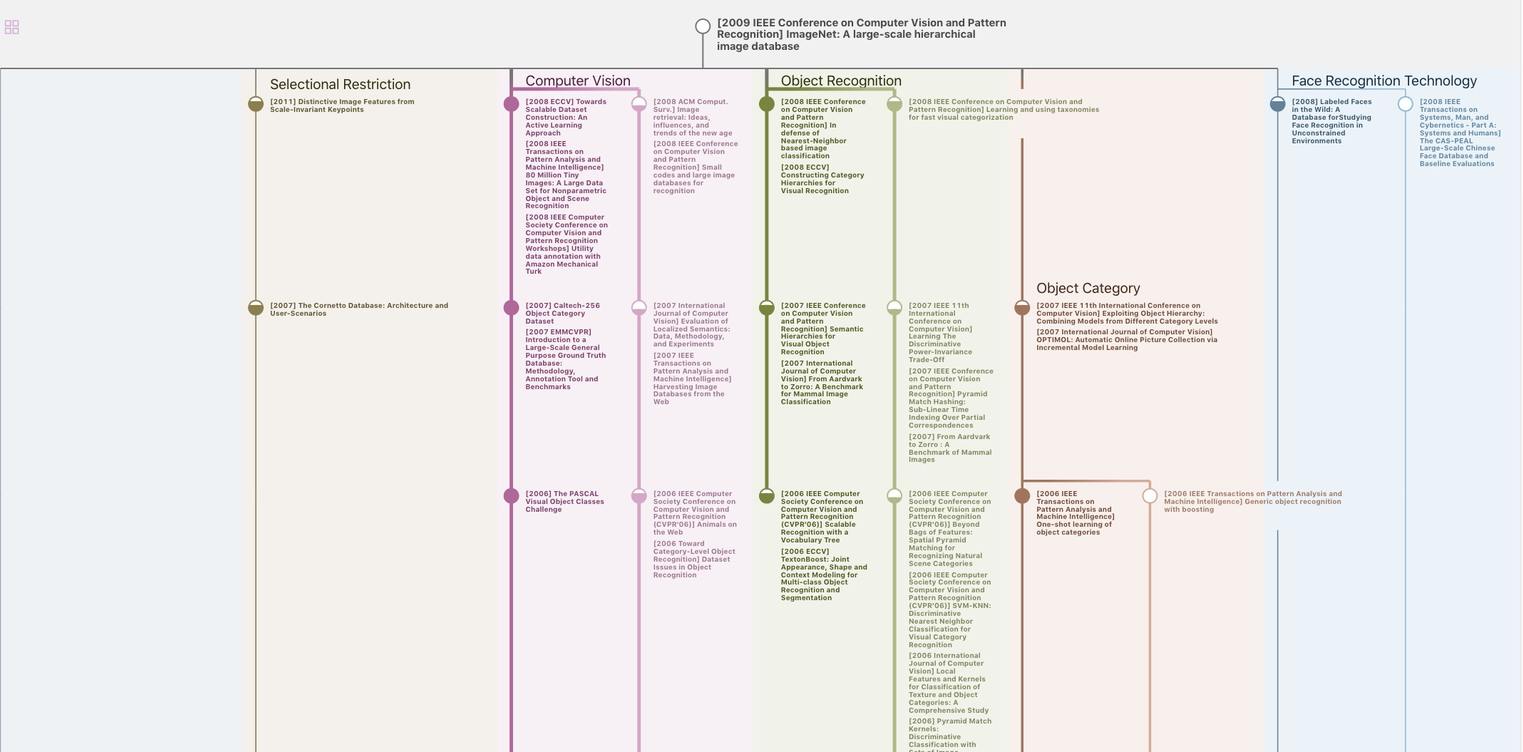
生成溯源树,研究论文发展脉络
Chat Paper
正在生成论文摘要