Unsupervised Anomaly Detection for Hard Drives
Proceedings of European Conference of the Prognostics and Health Management Society(2021)
摘要
In the age of smartsensors and industry 4.0 continuous monitoring of different machinery produce enormousamount of data, because of that datacenters are now-a-days a very important asset not only for large scale cloud providers, but also for medium to large enterprises,which decide to store in-house the ever increasing data collected during business operations. An efficient method for the maintenance of the great number of hard-drives housed in datacenters is critical to assure avaiability in a cost effective manner.Since 2013, Backblaze \url{https://www.backblaze.com/} has published statisticsand datasets for researchers to gain insights on hard drive performaces andtheir failures, in this paper more than 2.5 million records, following hard-drives S.M.A.R.T readings for over a year, will be analyzed. The objective of this paper is to show that it is possible to build a completelyunsupervised pipeline which produces an anomaly score that highlycorrelates to hard drives time to failure (TTF), in such a way a decisionto replace them can be made before failure, with minimal waste due to false alarms. Favorable comparisons with state of the art supervised classifiers will be presented. A brief example of how such a pipeline can beextended for data streams and continuos sensor monitoring will be given.
更多查看译文
AI 理解论文
溯源树
样例
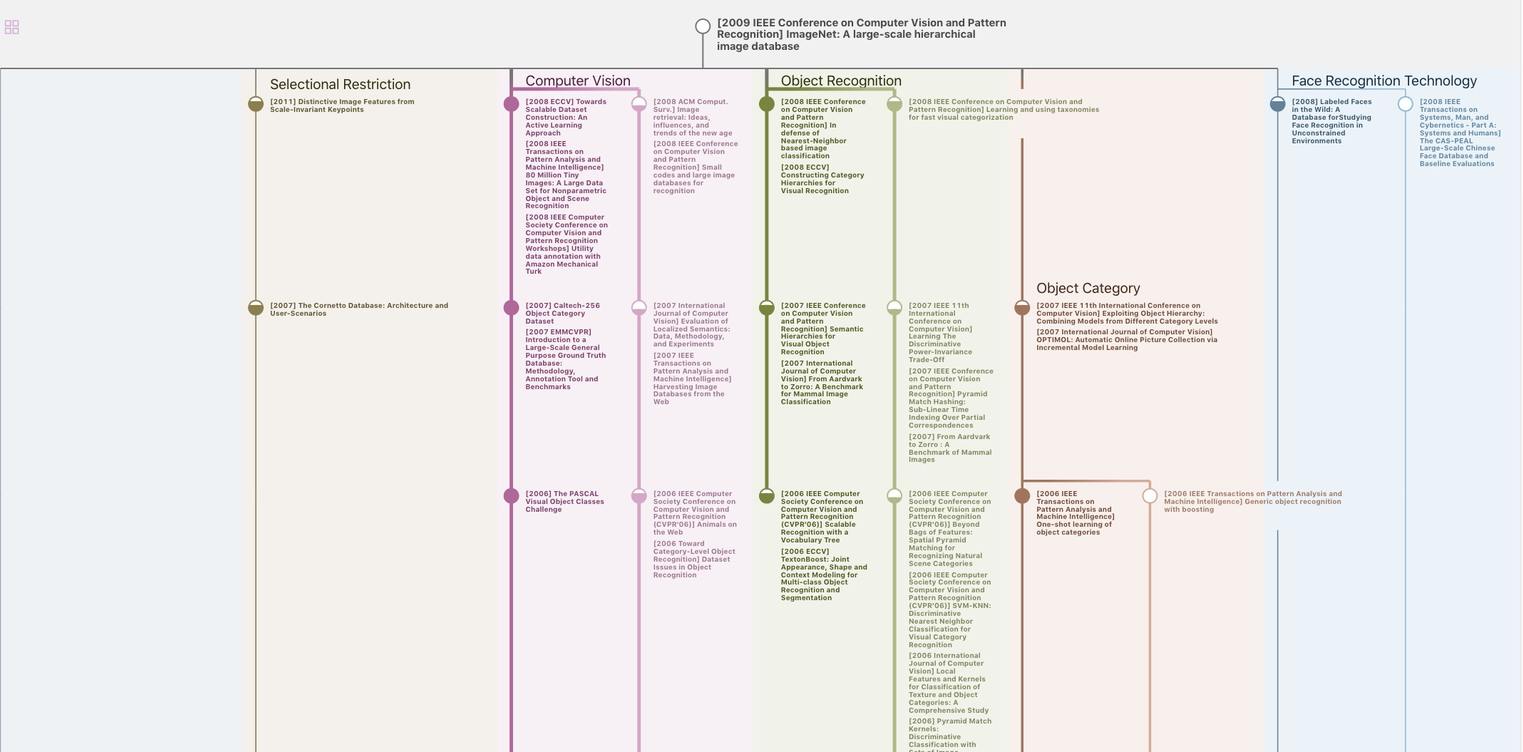
生成溯源树,研究论文发展脉络
Chat Paper
正在生成论文摘要