ξ-π: a nonparametric model for neural power spectra decomposition
biorxiv(2024)
摘要
The power spectra estimated from the brain recordings are the mixed representation of aperiodic transient activity and periodic oscillations, i.e., aperiodic component (AC) and periodic component (PC). Quantitative neurophysiology requires precise decomposition preceding parameterizing each component. However, the shape, statistical distribution, scale, and mixing mechanism of AC and PCs are unclear, challenging the effectiveness of current popular parametric models such as FOOOF, IRASA, BOSC, etc. Here, ξ - π was proposed to decompose the neural spectra by embedding the nonparametric spectra estimation with penalized Whittle likelihood and the shape language modeling into the expectation maximization frame-work. ξ - π was validated on the synthesized spectra with loss statistics and on the sleep EEG and the large sample iEEG with evaluation metrics and neurophysiological evidence. Compared to FOOOF, both the simulation presenting shape irregularities and the batch simulation with multiple isolated peaks indicated that ξ - π improved the fit of AC and PCs with less loss and higher F1-score in recognizing the centering frequencies and the number of peaks; the sleep EEG revealed that ξ - π produced more distinguishable AC exponents and improved the sleep state classification accuracy; the iEEG showed that ξ - π approached the clinical findings in peak discovery. Overall, ξ - π offered good performance in the spectra decomposition, which allows flexible parameterization using descriptive statistics or kernel functions. ξ - π may be a promising tool for brain signal decoding in fields such as cognitive neuroscience, brain-computer interface, neurofeedback, and brain diseases.
### Competing Interest Statement
The authors have declared no competing interest.
更多查看译文
AI 理解论文
溯源树
样例
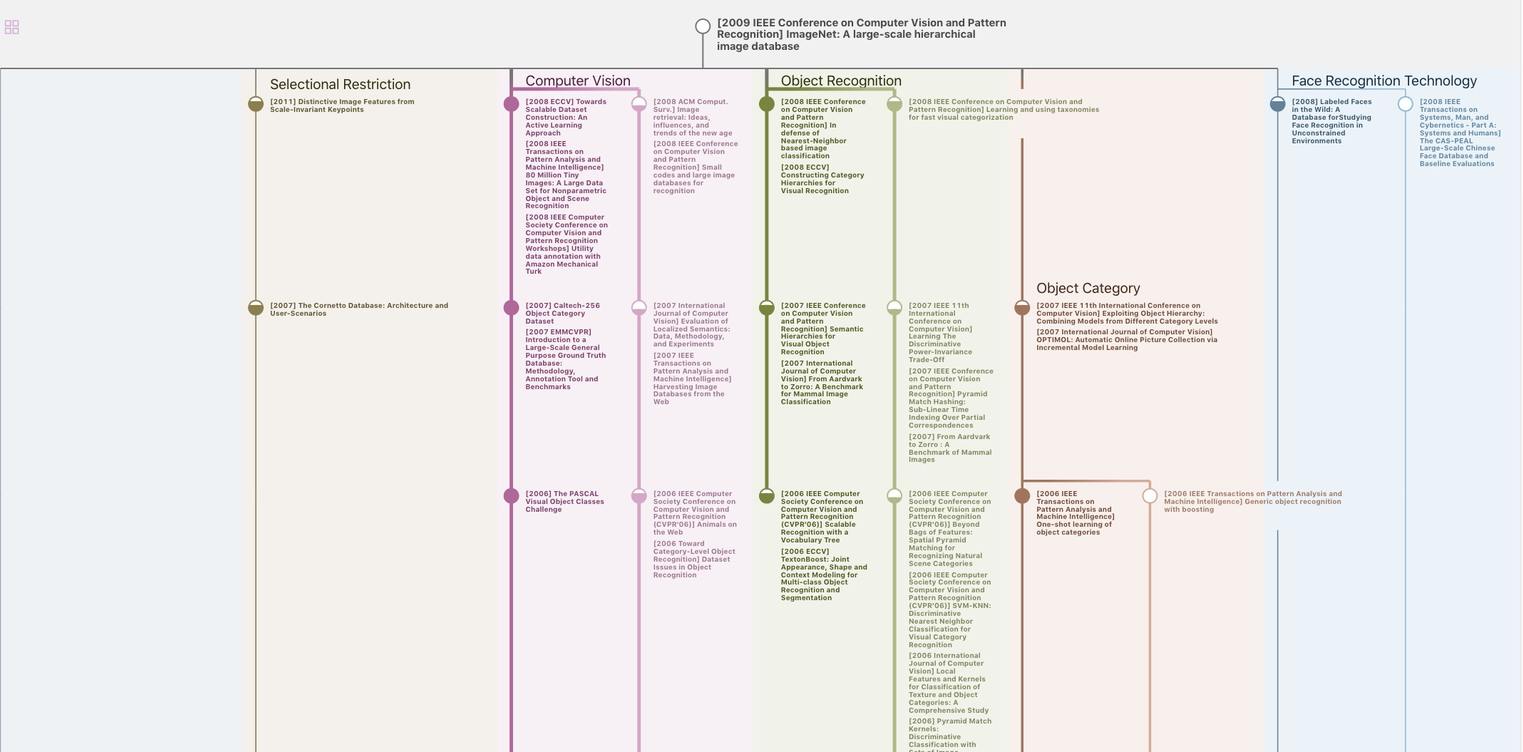
生成溯源树,研究论文发展脉络
Chat Paper
正在生成论文摘要