E-values as unnormalized weights in multiple testing
BIOMETRIKA(2023)
摘要
We study how to combine p-values and e-values, and design multiple testing procedures where both p-values and e-values are available for every hypothesis. Our results provide a new perspective on multiple testing with data-driven weights: while standard weighted multiple testing methods require the weights to deterministically add up to the number of hypotheses being tested, we show that this normalization is not required when the weights are e-values that are independent of the p-values. Such e-values can be obtained in meta-analysis where a primary dataset is used to compute p-values, and an independent secondary dataset is used to compute e-values. Going beyond meta-analysis, we showcase settings wherein independent e-values and p-values can be constructed on a single dataset itself. Our procedures can result in a substantial increase in power, especially if the nonnull hypotheses have e-values much larger than one.
更多查看译文
关键词
e-value,False discovery rate,Normalization,p-value,Weighted multiple testing
AI 理解论文
溯源树
样例
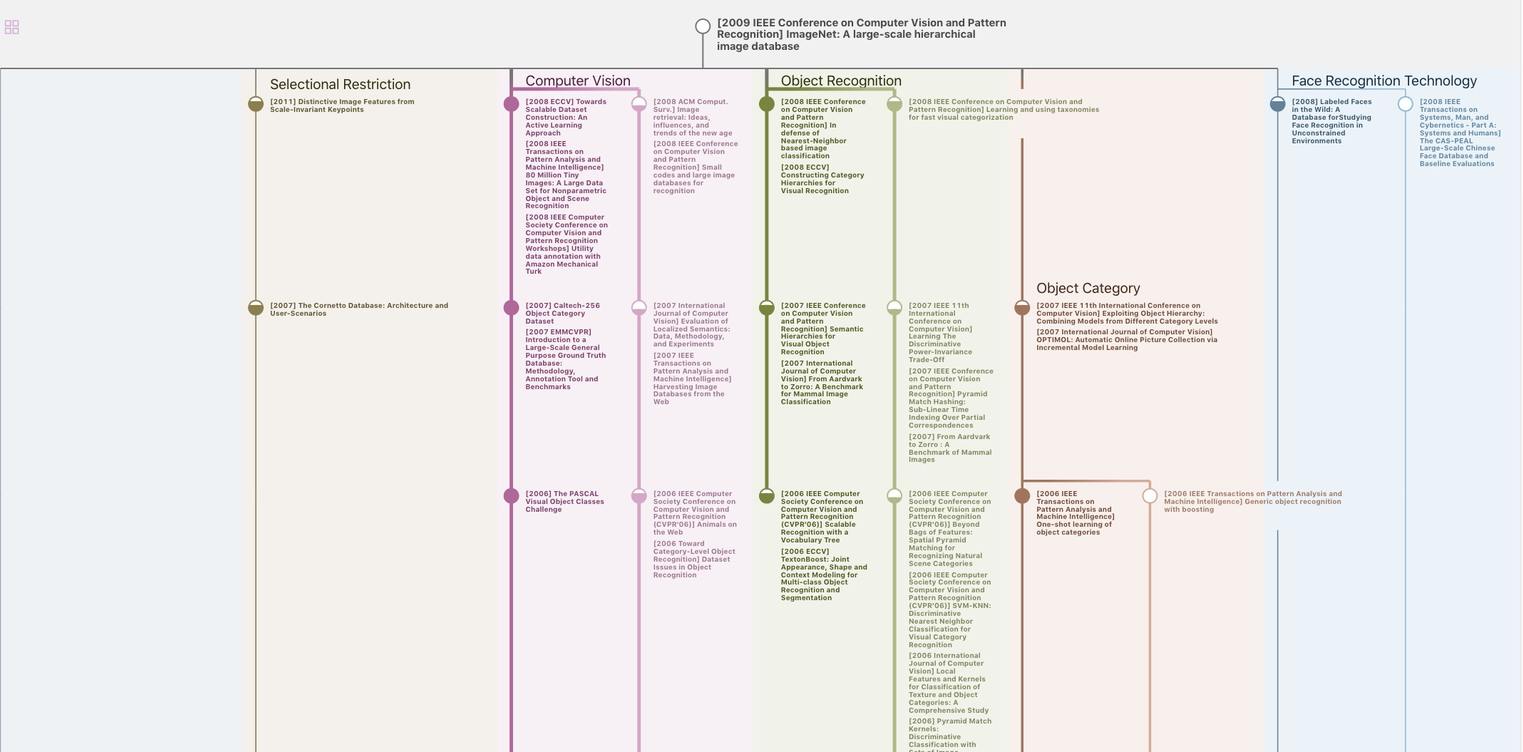
生成溯源树,研究论文发展脉络
Chat Paper
正在生成论文摘要