Learning Feature Fusion for Unsupervised Domain Adaptive Person Re-identification
arXiv (Cornell University)(2022)
摘要
Unsupervised domain adaptive (UDA) person re-identification (ReID) has gained increasing attention for its effectiveness on the target domain without manual annotations. Most fine-tuning based UDA person ReID methods focus on encoding global features for pseudo labels generation, neglecting the local feature that can provide for the fine-grained information. To handle this issue, we propose a Learning Feature Fusion (LF2) framework for adaptively learning to fuse global and local features to obtain a more comprehensive fusion feature representation. Specifically, we first pre-train our model within a source domain, then fine-tune the model on unlabeled target domain based on the teacher-student training strategy. The average weighting teacher network is designed to encode global features, while the student network updating at each iteration is responsible for fine-grained local features. By fusing these multi-view features, multi-level clustering is adopted to generate diverse pseudo labels. In particular, a learnable Fusion Module (FM) for giving prominence to fine-grained local information within the global feature is also proposed to avoid obscure learning of multiple pseudo labels. Experiments show that our proposed LF2 framework outperforms the state-of-the-art with 73.5% mAP and 83.7% Rank1 on Market1501 to DukeMTMC-ReID, and achieves 83.2% mAP and 92.8% Rank1 on DukeMTMC-ReID to Market1501.
更多查看译文
关键词
unsupervised domain adaptive person
AI 理解论文
溯源树
样例
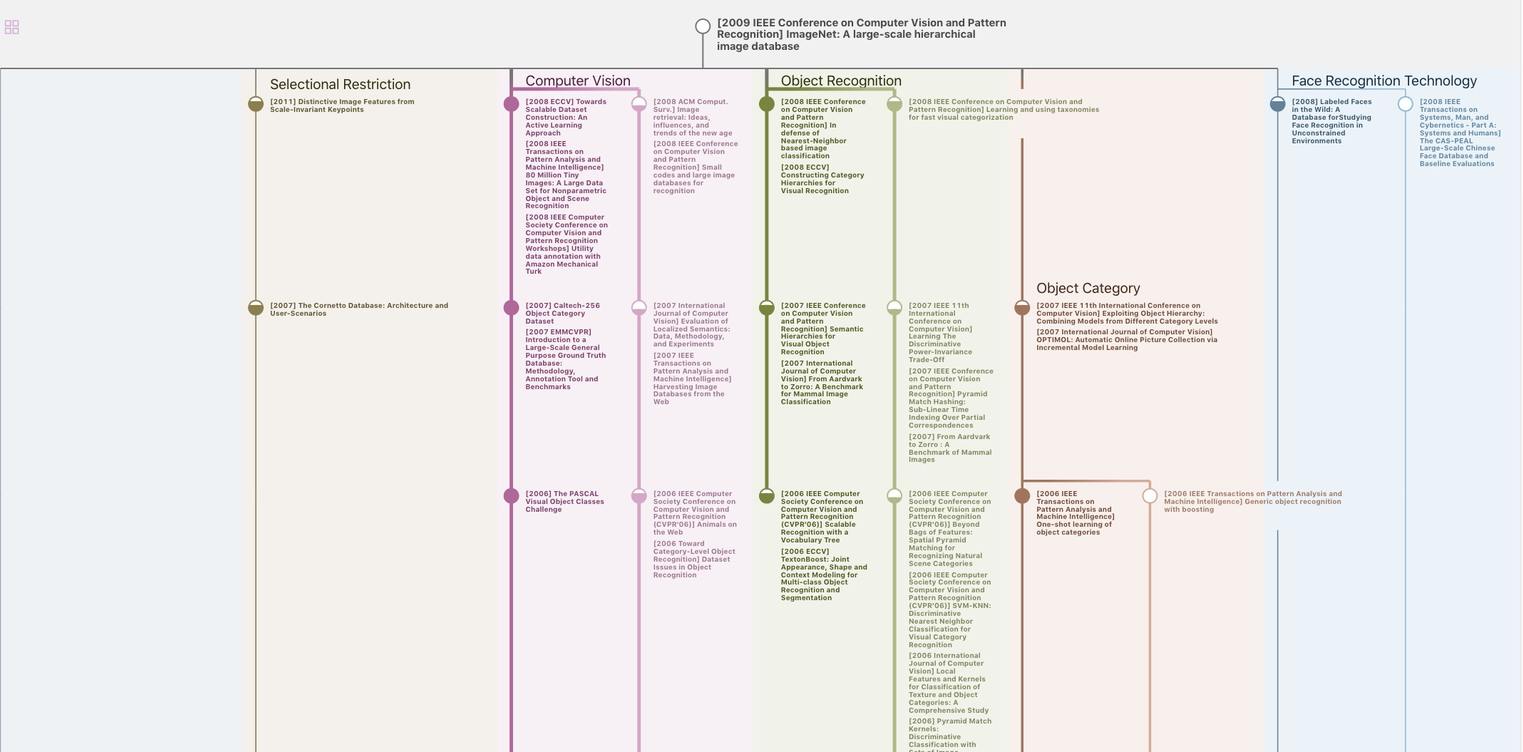
生成溯源树,研究论文发展脉络
Chat Paper
正在生成论文摘要