Stochastic Data-Driven Predictive Control: Regularization, Estimation, and Constraint Tightening
CoRR(2023)
摘要
Data-driven predictive control methods based on the Willems' fundamental lemma have shown great success in recent years. These approaches use receding horizon predictive control with nonparametric data-driven predictors instead of model-based predictors. This study addresses three problems of applying such algorithms under unbounded stochastic uncertainties: 1) tuning-free regularizer design, 2) initial condition estimation, and 3) reliable constraint satisfaction, by using stochastic prediction error quantification. The regularizer is designed by leveraging the expected output cost. An initial condition estimator is proposed by filtering the measurements with the one-step-ahead stochastic data-driven prediction. A novel constraint-tightening method, using second-order cone constraints, is presented to ensure high-probability chance constraint satisfaction. Numerical results demonstrate that the proposed methods lead to satisfactory control performance in terms of both control cost and constraint satisfaction, with significantly improved initial condition estimation.
更多查看译文
AI 理解论文
溯源树
样例
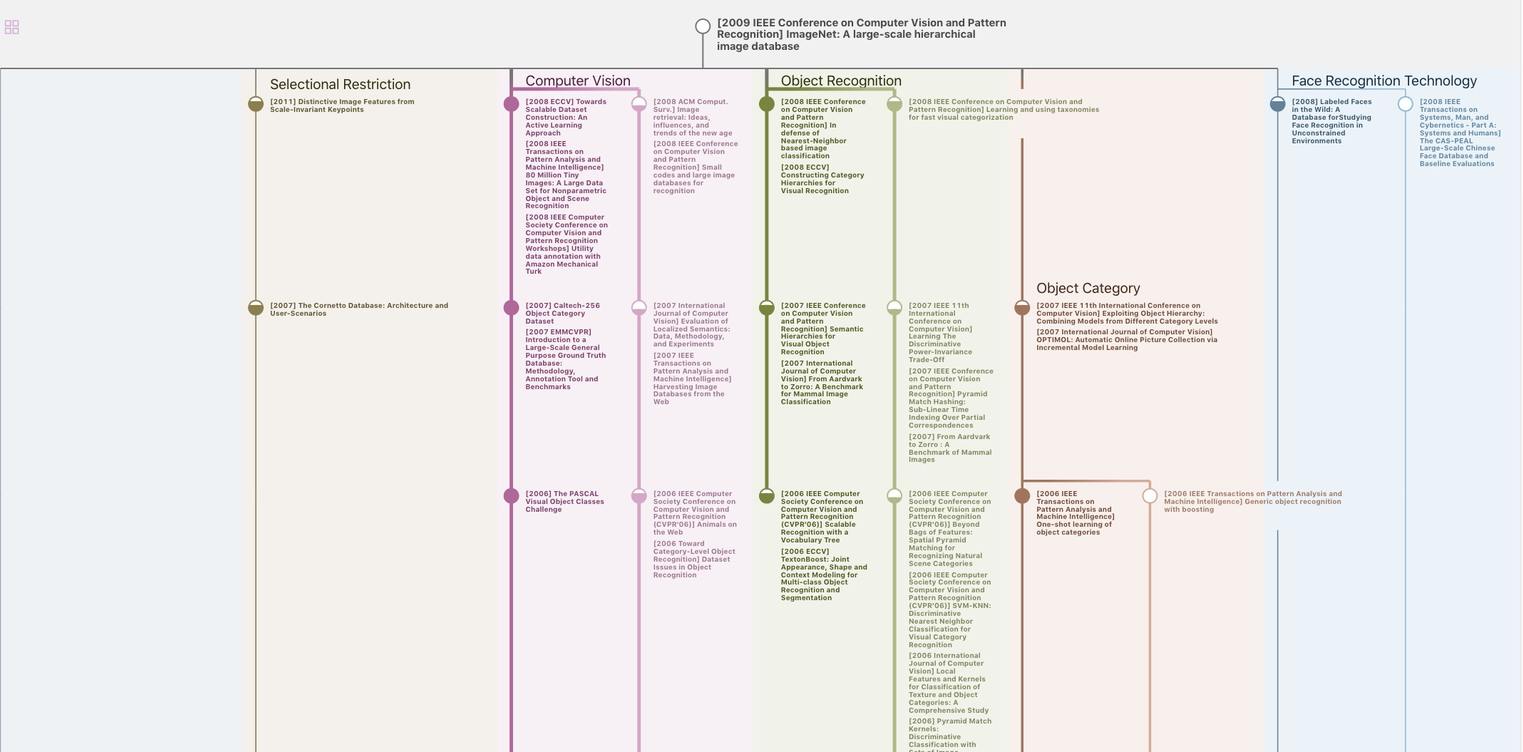
生成溯源树,研究论文发展脉络
Chat Paper
正在生成论文摘要