Learning Efficient, Explainable and Discriminative Representations for Pulmonary Nodules Classification
arXiv (Cornell University)(2021)
摘要
Automatic pulmonary nodules classification is significant for early diagnosis of lung cancers. Recently, deep learning techniques have enabled remarkable progress in this field. However, these deep models are typically of high computational complexity and work in a black-box manner. To combat these challenges, in this work, we aim to build an efficient and (partially) explainable classification model. Specially, we use \emph{neural architecture search} (NAS) to automatically search 3D network architectures with excellent accuracy/speed trade-off. Besides, we use the convolutional block attention module (CBAM) in the networks, which helps us understand the reasoning process. During training, we use A-Softmax loss to learn angularly discriminative representations. In the inference stage, we employ an ensemble of diverse neural networks to improve the prediction accuracy and robustness. We conduct extensive experiments on the LIDC-IDRI database. Compared with previous state-of-the-art, our model shows highly comparable performance by using less than 1/40 parameters. Besides, empirical study shows that the reasoning process of learned networks is in conformity with physicians' diagnosis. Related code and results have been released at: https://github.com/fei-hdu/NAS-Lung.
更多查看译文
关键词
discriminative representations,classification,learning efficient,nodules
AI 理解论文
溯源树
样例
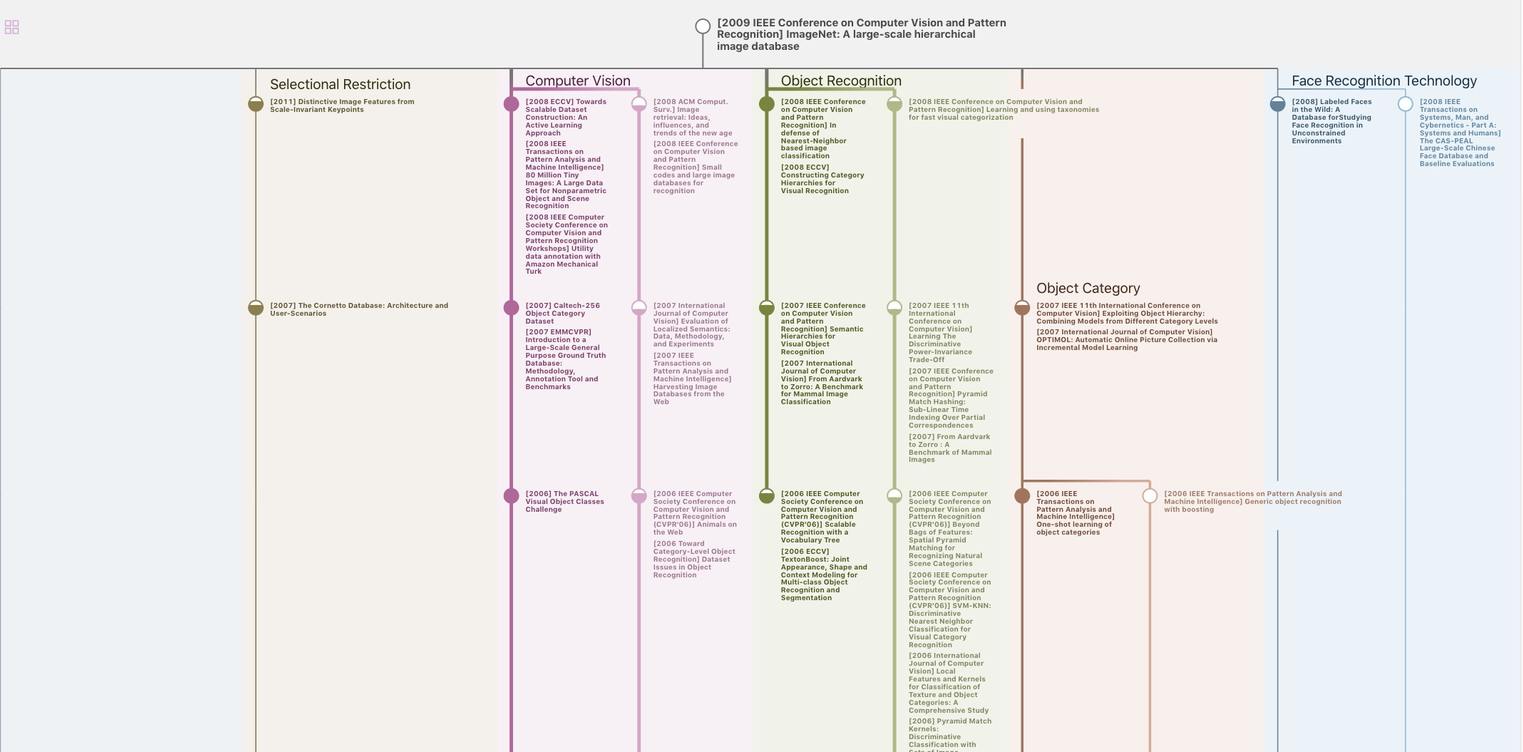
生成溯源树,研究论文发展脉络
Chat Paper
正在生成论文摘要