Equalized Aggregation for Heterogeneous Federated Mobile Edge Learning.
IEEE Trans. Mob. Comput.(2024)
摘要
Federated Learning (FL) is widely used in mobile edge applications. However, the heterogeneity issues of mobile edge devices pose significant challenges to the generalization of the global model in FL. In this paper, we propose LegoFL to simultaneously solve multiple heterogeneity issues in response to mobile edge computing characteristics. LegoFL identifies two types of heterogeneous behaviors in FL, namely heterogeneous parameter training and communication behaviors, to address multiple heterogeneity issues. These two types of heterogeneous behaviors result in feature and feature representation range mismatches between local communication parameters. To reduce these mismatches and improve the generalization of the global model, LegoFL dynamically distinguishes the parameter feature representation of different nodes using the global model's common feature as guidance. Then, under the connection states and system communication constraints, LegoFL dynamically selects contribution parameters on each device that can guarantee the generalization and performance of the global model for communication. Finally, to avoid the overfitting problem of the global model, heterogeneous local models are aggregated at the central server with matched feature representations. Extensive experiments on various datasets show that LegoFL achieves competitive performance. The accuracy and communication efficiency are improved by up to 12.86
$\%$
and 4.09× compared to state-of-the-art approaches.
更多查看译文
关键词
Federated Learning,heterogeneous mobile edge computing,equalized aggregation
AI 理解论文
溯源树
样例
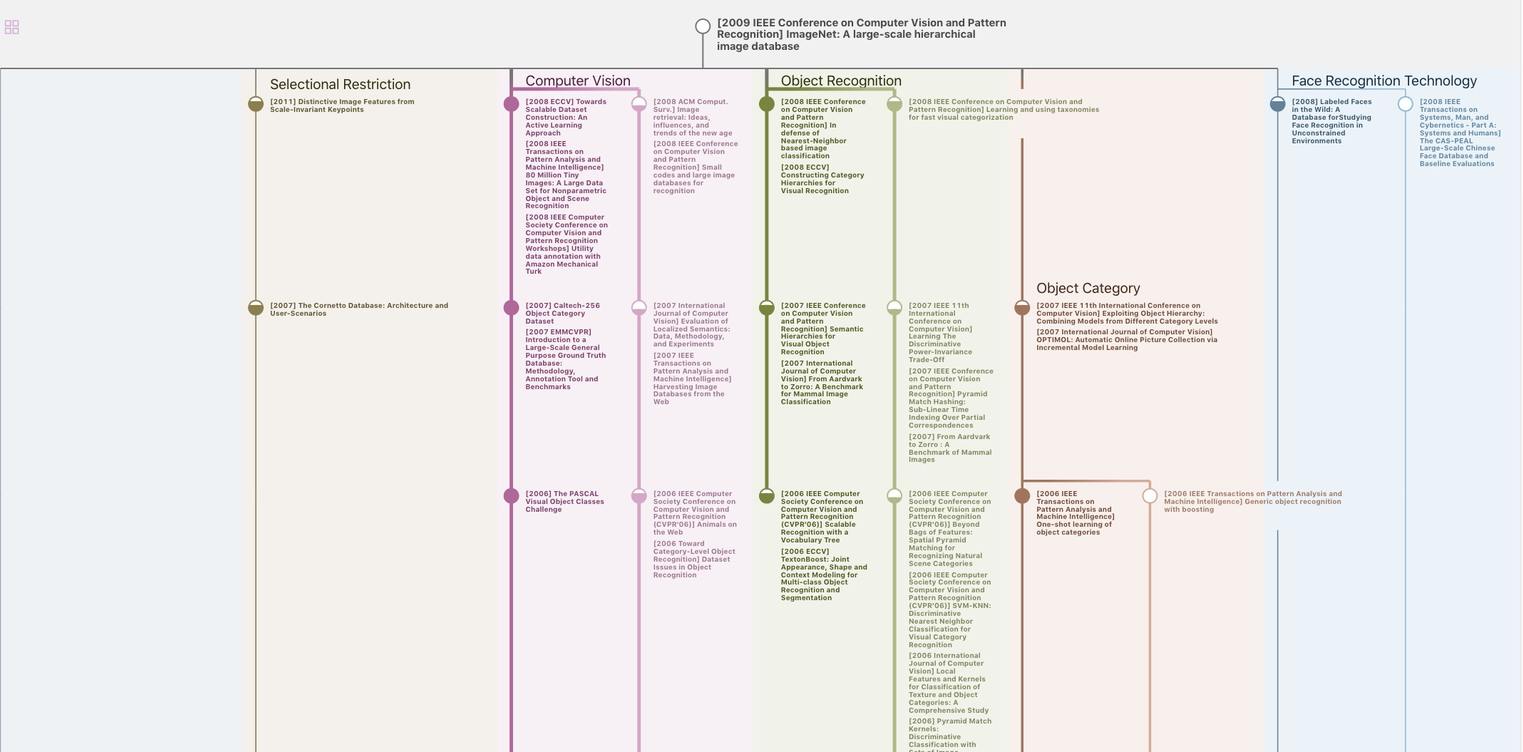
生成溯源树,研究论文发展脉络
Chat Paper
正在生成论文摘要