ContriMix: Unsupervised disentanglement of content and attribute for domain generalization in microscopy image analysis
arXiv (Cornell University)(2023)
摘要
Domain generalization is critical for real-world applications of machine learning models to microscopy images, including histopathology and fluorescence imaging. Artifacts in histopathology arise through a complex combination of factors relating to tissue collection and laboratory processing, as well as factors intrinsic to patient samples. In fluorescence imaging, these artifacts stem from variations across experimental batches. The complexity and subtlety of these artifacts make the enumeration of data domains intractable. Therefore, augmentation-based methods of domain generalization that require domain identifiers and manual fine-tuning are inadequate in this setting. To overcome this challenge, we introduce ContriMix, a domain generalization technique that learns to generate synthetic images by disentangling and permuting the biological content ("content") and technical variations ("attributes") in microscopy images. ContriMix does not rely on domain identifiers or handcrafted augmentations and makes no assumptions about the input characteristics of images. We assess the performance of ContriMix on two pathology datasets (Camelyon17-WILDS and a prostate cell classification dataset) and one fluorescence microscopy dataset (RxRx1-WILDS). ContriMix outperforms current state-of-the-art methods in all datasets, motivating its usage for microscopy image analysis in real-world settings where domain information is hard to come by.
更多查看译文
关键词
microscopy,domain generalization,disentanglement,contrimix,image
AI 理解论文
溯源树
样例
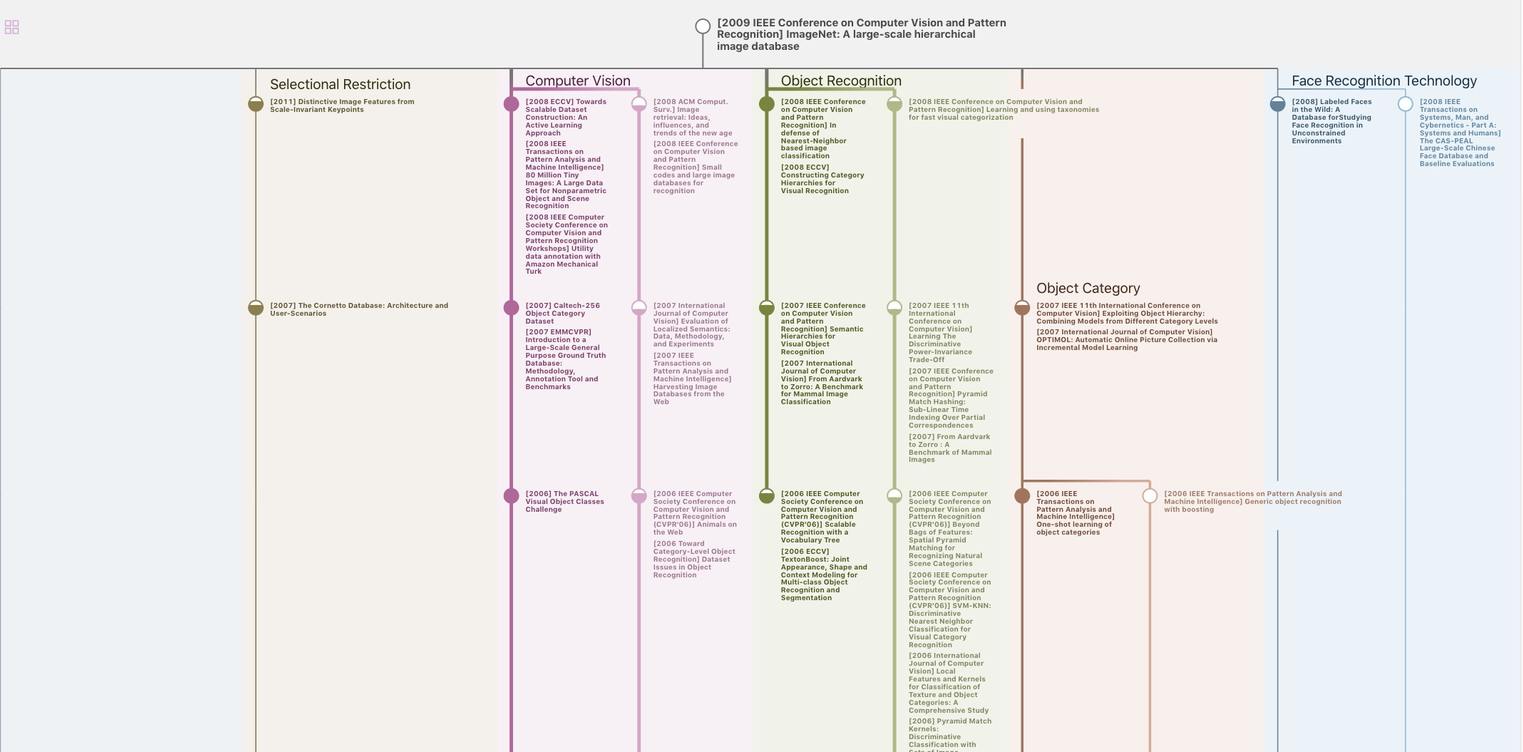
生成溯源树,研究论文发展脉络
Chat Paper
正在生成论文摘要