Benchmarking Quantum Surrogate Models on Scarce and Noisy Data.
International Conference on Agents and Artificial Intelligence(2024)
摘要
Surrogate models are ubiquitously used in industry and academia to efficiently approximate given black box functions. As state-of-the-art methods from classical machine learning frequently struggle to solve this problem accurately for the often scarce and noisy data sets in practical applications, investigating novel approaches is of great interest. Motivated by recent theoretical results indicating that quantum neural networks (QNNs) have the potential to outperform their classical analogs in the presence of scarce and noisy data, we benchmark their qualitative performance for this scenario empirically. Our contribution displays the first application-centered approach of using QNNs as surrogate models on higher dimensional, real world data. When compared to a classical artificial neural network with a similar number of parameters, our QNN demonstrates significantly better results for noisy and scarce data, and thus motivates future work to explore this potential quantum advantage in surrogate modelling. Finally, we demonstrate the performance of current NISQ hardware experimentally and estimate the gate fidelities necessary to replicate our simulation results.
更多查看译文
关键词
surrogate modeling,quantum
AI 理解论文
溯源树
样例
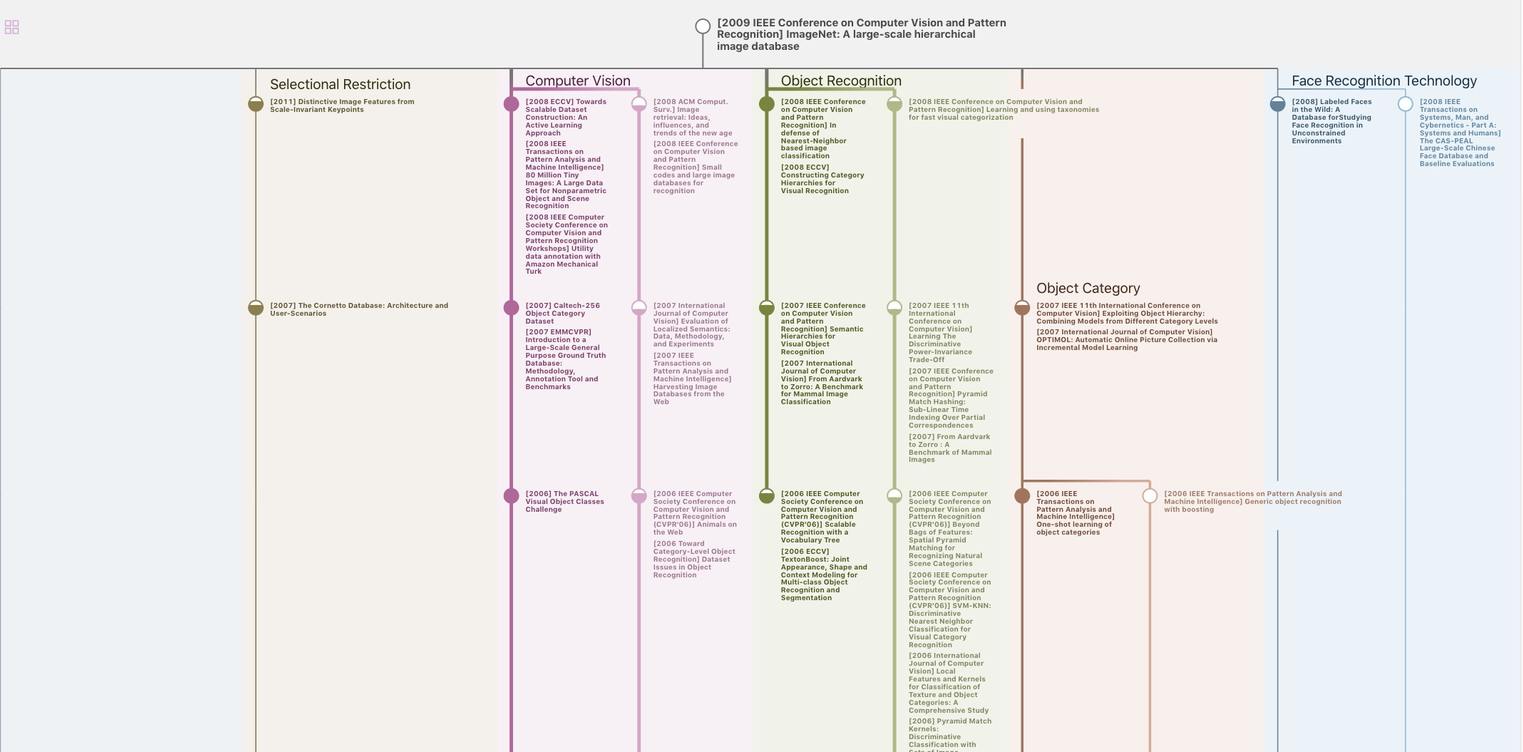
生成溯源树,研究论文发展脉络
Chat Paper
正在生成论文摘要