An Effective Lightweight Crowd Counting Method Based on an Encoder-Decoder Network for Internet of Video Things
IEEE internet of things journal(2024)
摘要
An emerging Internet of Video Things (IoVT) application, crowd counting is a computer vision task where the number of heads in a crowded scene is estimated. In recent years, it has attracted increasing attention from academia and industry because of its great potential value in public safety and urban planning. However, it has become a challenge to cross the gap between the increasingly heavy and complex network architecture widely used for the pursuit of counting with high accuracy and the constrained computing and storage resources in the edge computing environment. To address this issue, an effective lightweight crowd counting method based on an encoder–decoder network, named lightweight crowd counting network (LEDCrowdNet), is proposed to achieve an optimal tradeoff between counting performance and running speed for edge applications of IoVT. In particular, an improved MobileViT module as an encoder is designed to extract global-local crowd features of various scales. The decoder is composed of the adaptive multiscale large kernel attention module (AMLKA) and the lightweight counting atrous spatial pyramid pooling process module (LC-ASPP), which can perform end-to-end training to obtain the final density map. The proposed LEDCrowdNet is suitable for deployment on two edge computing platforms (NVIDIA Jetson Xavier NX and Coral Edge TPU) to reduce the number of floating point operations (FLOPs) without a significant drop in accuracy. Extensive experiments on five mainstream benchmarks (ShanghaiTech Part_A/B, UCF_CC_50, UCF-QNRF, WorldExpo’10, and RSOC data sets) verify the correctness and efficiency of our method.
更多查看译文
关键词
Convolution neural network,crowd counting,edge computing,lightweight network
AI 理解论文
溯源树
样例
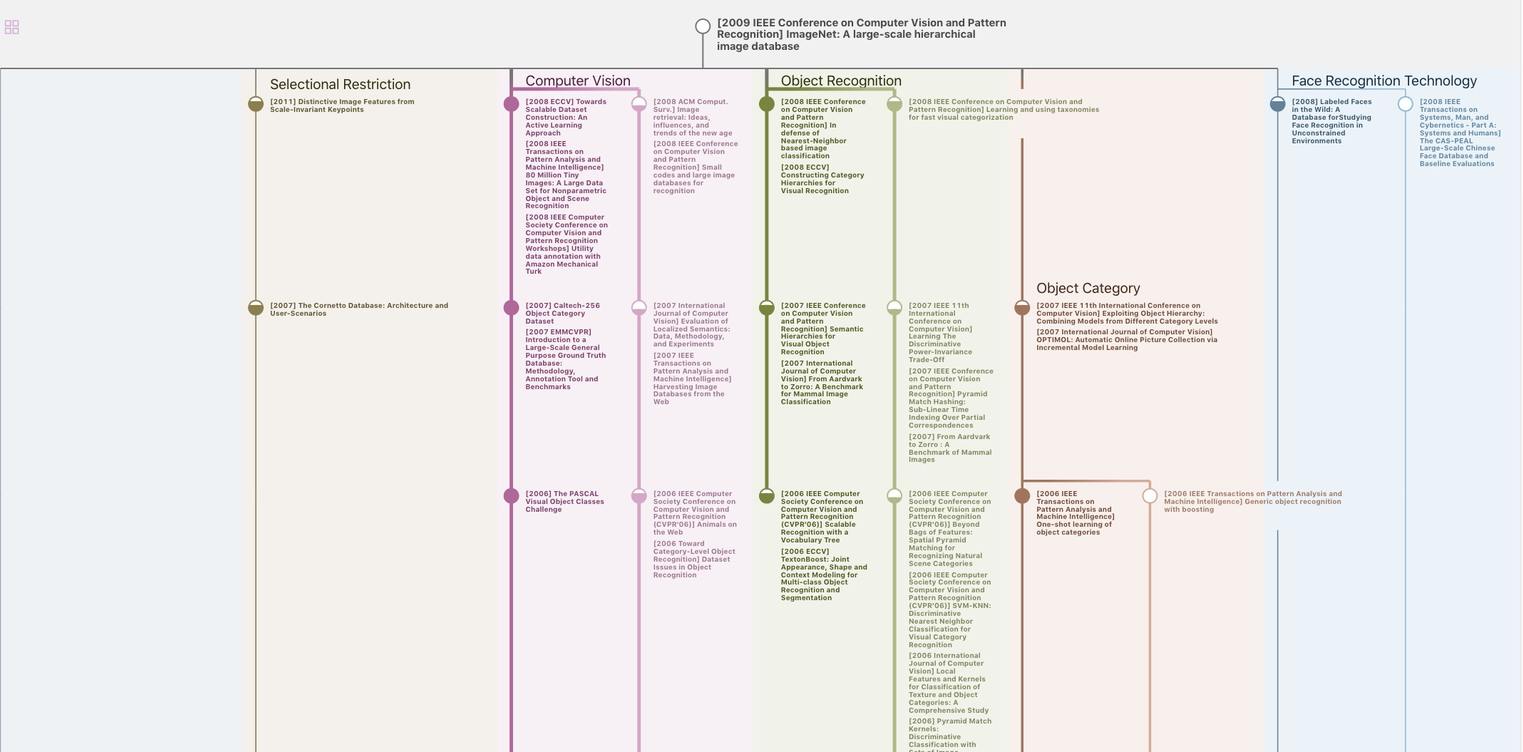
生成溯源树,研究论文发展脉络
Chat Paper
正在生成论文摘要