Symbolic regression based interpretable data-driven soft-sensor for process quality control
Computer-aided chemical engineering(2023)
摘要
Accurately predicting key performance indicators for product quality control is a research priority within the process industry. Physical models capable of predicting real-time process indicators of complex formulations are traditionally difficult to construct or retrofit, especially when the process is operated under dynamic process conditions and incorporates complex mixing phenomena. A solution to this employs the use of machine learning models to develop a soft-sensor using the information rich process data available, however this method lacks interpretability as it is difficult to extract physical information from the machine learning models, and the models are less user-friendly to implement within an existing factory advance process control system. To fill this gap, in this study we propose the use of symbolic regression, an interpretable machine learning technique, which aims to search the space over a subset of pre-defined mathematical functions to make accurate predictions of product quality. The high dimensional historical industrial data is first screened, and partial least squares is used to extract critical information regarding important operating regions and process parameters. Statistical features of critical process parameters are then employed within different symbolic regression based frameworks to obtain suitable models for batch quality prediction. Moreover, Monte Carlo sampling is adopted to estimate model uncertainty and evaluate reliability of the constructed interpretable models. To evaluate the model’s performance, predictions were made for batches operated over different seasons and formulations. In addition, these models are benchmarked against a recently developed heteroscedastic noise neural network based data-driven soft-sensor. It is found that symbolic regression provided expressions with high accuracy, robustness, and responsiveness to changing process data suggesting that information about the true underlying correlation between the process data and a key performance indicator has been extracted. This research highlights the great potential of interpretable machine learning techniques for future industrial application.
更多查看译文
关键词
process quality control,symbolic regression,quality control,data-driven,soft-sensor
AI 理解论文
溯源树
样例
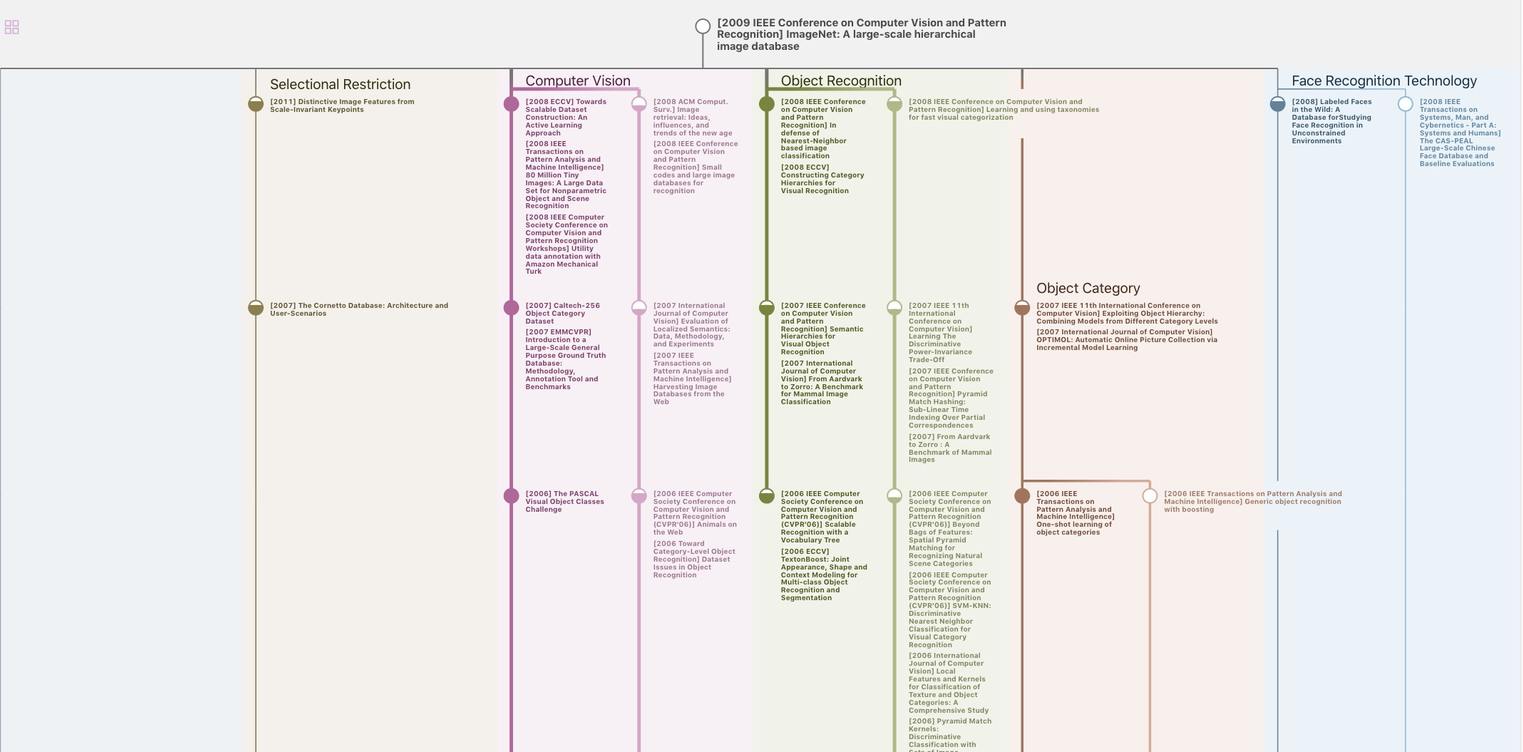
生成溯源树,研究论文发展脉络
Chat Paper
正在生成论文摘要