LaFiCMIL: Rethinking Large File Classification from the Perspective of Correlated Multiple Instance Learning
arXiv (Cornell University)(2023)
摘要
Transformer-based models, such as BERT, have revolutionized various language tasks, but still struggle with large file classification due to their input limit (e.g., 512 tokens). Despite several attempts to alleviate this limitation, no method consistently excels across all benchmark datasets, primarily because they can only extract partial essential information from the input file. Additionally, they fail to adapt to the varied properties of different types of large files. In this work, we tackle this problem from the perspective of correlated multiple instance learning. The proposed approach, LaFiCMIL, serves as a versatile framework applicable to various large file classification tasks covering binary, multi-class, and multi-label classification tasks, spanning various domains including Natural Language Processing, Programming Language Processing, and Android Analysis. To evaluate its effectiveness, we employ eight benchmark datasets pertaining to Long Document Classification, Code Defect Detection, and Android Malware Detection. Leveraging BERT-family models as feature extractors, our experimental results demonstrate that LaFiCMIL achieves new state-of-the-art performance across all benchmark datasets. This is largely attributable to its capability of scaling BERT up to nearly 20K tokens, running on a single Tesla V-100 GPU with 32G of memory.
更多查看译文
关键词
correlated multiple instance learning,large file classification,laficmil
AI 理解论文
溯源树
样例
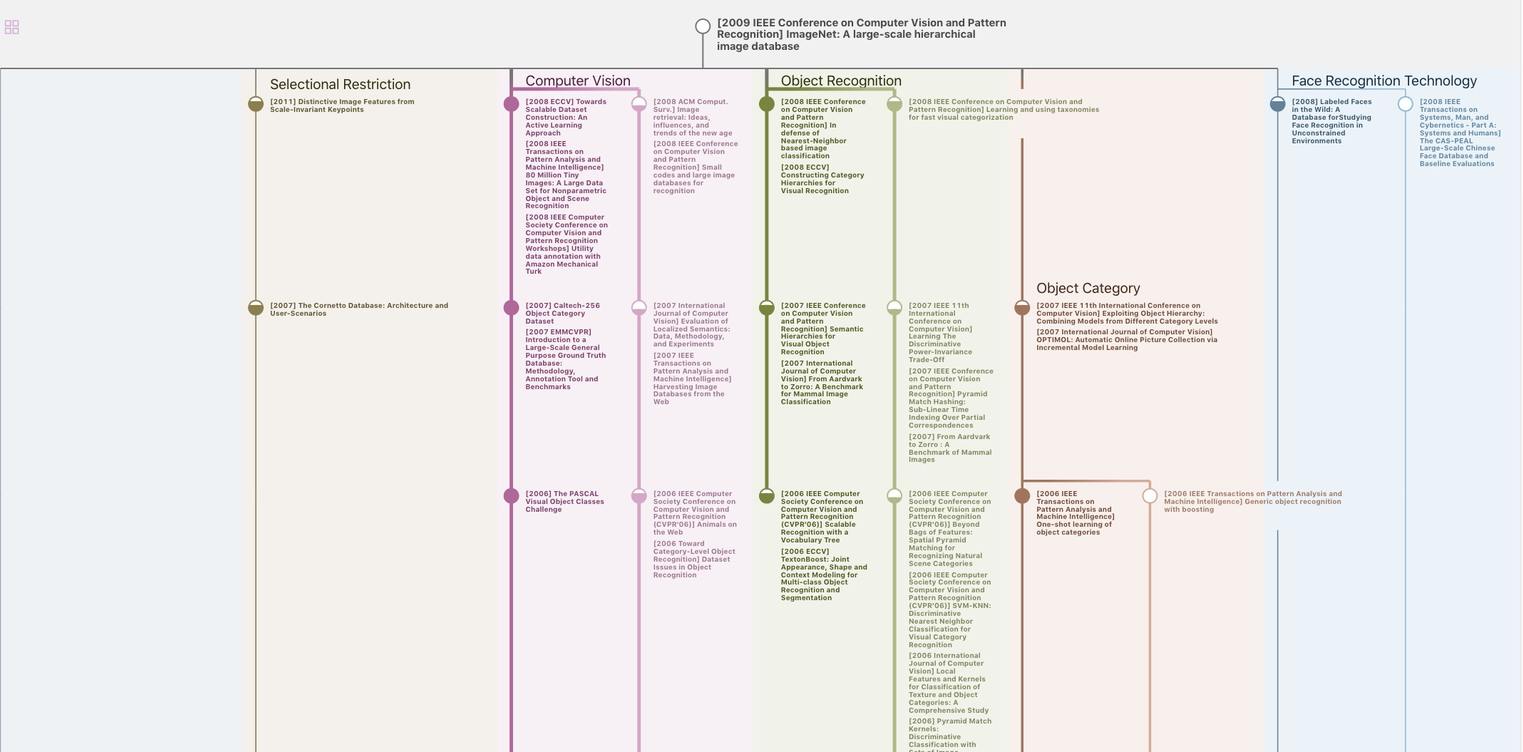
生成溯源树,研究论文发展脉络
Chat Paper
正在生成论文摘要