Optimization of building demand flexibility using reinforcement learning and rule-based expert systems
APPLIED ENERGY(2023)
摘要
The increasing use of renewable energy in buildings requires optimization of building demand flexibility to reduce energy costs and carbon emissions. Nevertheless, the optimization process is generally challenging that needs to consider the on-site intermittent energy supply, dynamic building energy demand, and proper utilization of energy storage systems. Leveraging the growing availability of operational data in buildings, datadriven strategies such as reinforcement learning (RL) have emerged as effective approaches to optimizing building demand flexibility. However, training a reliable RL agent is practically data-demanding and timeconsuming, limiting its practical applicability. This study proposes a new strategy that integrates a rule-based expert system (RBES) and RL agents into the decision-making process to jointly reduce building energy costs, minimize the peak-to-average ratio (PAR) of grid power, and maximize PV self-consumption. In this strategy, the RBES determines system operation directly in less complex decision-making scenarios, while, in more intricate decision-making environments, it provides a reference decision for RL to explore optimal solutions further. This integration empowers RL agents to avoid unnecessary exploration and significantly enhance learning efficiency. The proposed strategy was tested using PV generation data and energy consumption data of a low energy office building. The results demonstrated an 85.7% improvement in RL learning efficiency and this strategy can
更多查看译文
关键词
Demand flexibility,Reinforcement learning,Learning efficiency,Heuristic rules
AI 理解论文
溯源树
样例
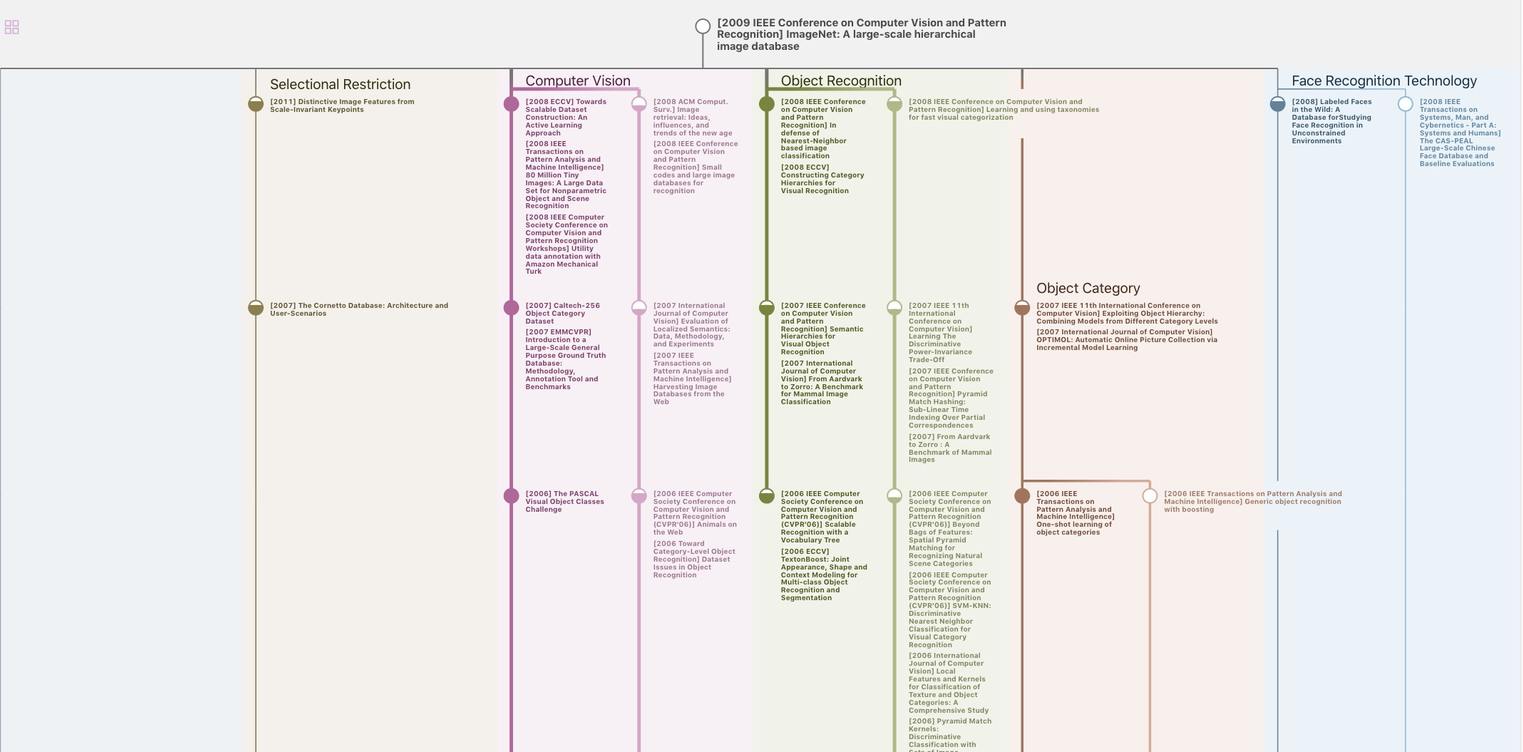
生成溯源树,研究论文发展脉络
Chat Paper
正在生成论文摘要