Physics-inspired Equivariant Descriptors of Non-bonded Interactions
arXiv (Cornell University)(2023)
摘要
One essential ingredient in many machine learning (ML) based methods for atomistic modeling of materials and molecules is the use of locality. While allowing better system-size scaling, this systematically neglects long-range (LR) effects, such as electrostatics or dispersion interaction. We present an extension of the long distance equivariant (LODE) framework that can handle diverse LR interactions in a consistent way, and seamlessly integrates with preexisting methods by building new sets of atom centered features. We provide a direct physical interpretation of these using the multipole expansion, which allows for simpler and more efficient implementations. The framework is applied to simple toy systems as proof of concept, and a heterogeneous set of molecular dimers to push the method to its limits. By generalizing LODE to arbitrary asymptotic behaviors, we provide a coherent approach to treat arbitrary two- and many-body non-bonded interactions in the data-driven modeling of matter.
更多查看译文
关键词
equivariant descriptors,interactions,physics-inspired,non-bonded
AI 理解论文
溯源树
样例
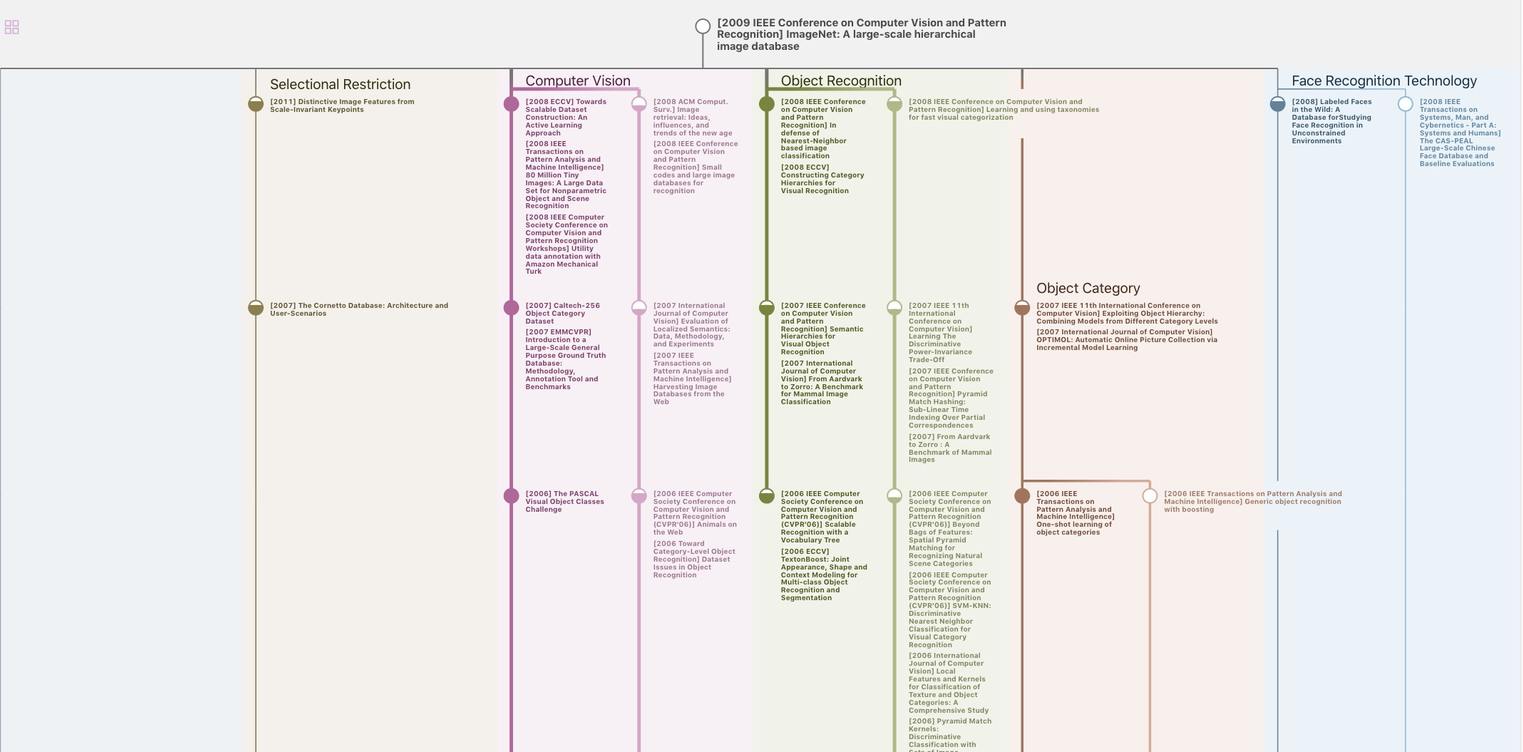
生成溯源树,研究论文发展脉络
Chat Paper
正在生成论文摘要