Sequential Bayesian-optimized graphene synthesis by direct solar-thermal chemical vapor deposition
Scientific Reports(2024)
摘要
This work reports the use of a high-flux solar simulator that mimics the solar spectrum and a cold-wall CVD reactor to demonstrate the feasibility of utilizing a renewable energy resource in synthesizing graphene under various conditions. A parametric study of process parameters was conducted using a probabilistic approach. Gaussian process regression serves as a surrogate to establish a prior for Bayesian optimization, and an information acquisition function is employed to identify conditions that yield high-quality products. Backscattered electron images and Raman mapping were used to assess the effects of growth conditions on graphene characteristic sizes, film quality, and uniformity. We report the synthesis of high-quality single-layer graphene (SLG) and AB-stacked bilayer graphene films in a one-step, short-time process with I_D/I_G ratios of 0.21 and 0.14, respectively. Electron diffraction analysis shows peak intensities that resemble SLG and AB-bilayer graphene with up to 5 and 20 m grain sizes, respectively. The optical transmissivities of SLG and AB-bilayer graphene fall between 0.959–0.977 and 0.929–0.953, whereas the sheet resistances measured by a 4-point probe with 1 mm spacing are 15.5 ± 4.6 and 3.4 ± 1.5 k Ω /sq, respectively. Further scale-up of the optimized graphene growth area was achieved by flattening the insolation profile, leading to spatial uniformity up to 13 mm in radius. Direct solar capture for CVD synthesis enable a practical and sustainable option for synthesizing graphene films applicable for photonic and electronic applications.
更多查看译文
AI 理解论文
溯源树
样例
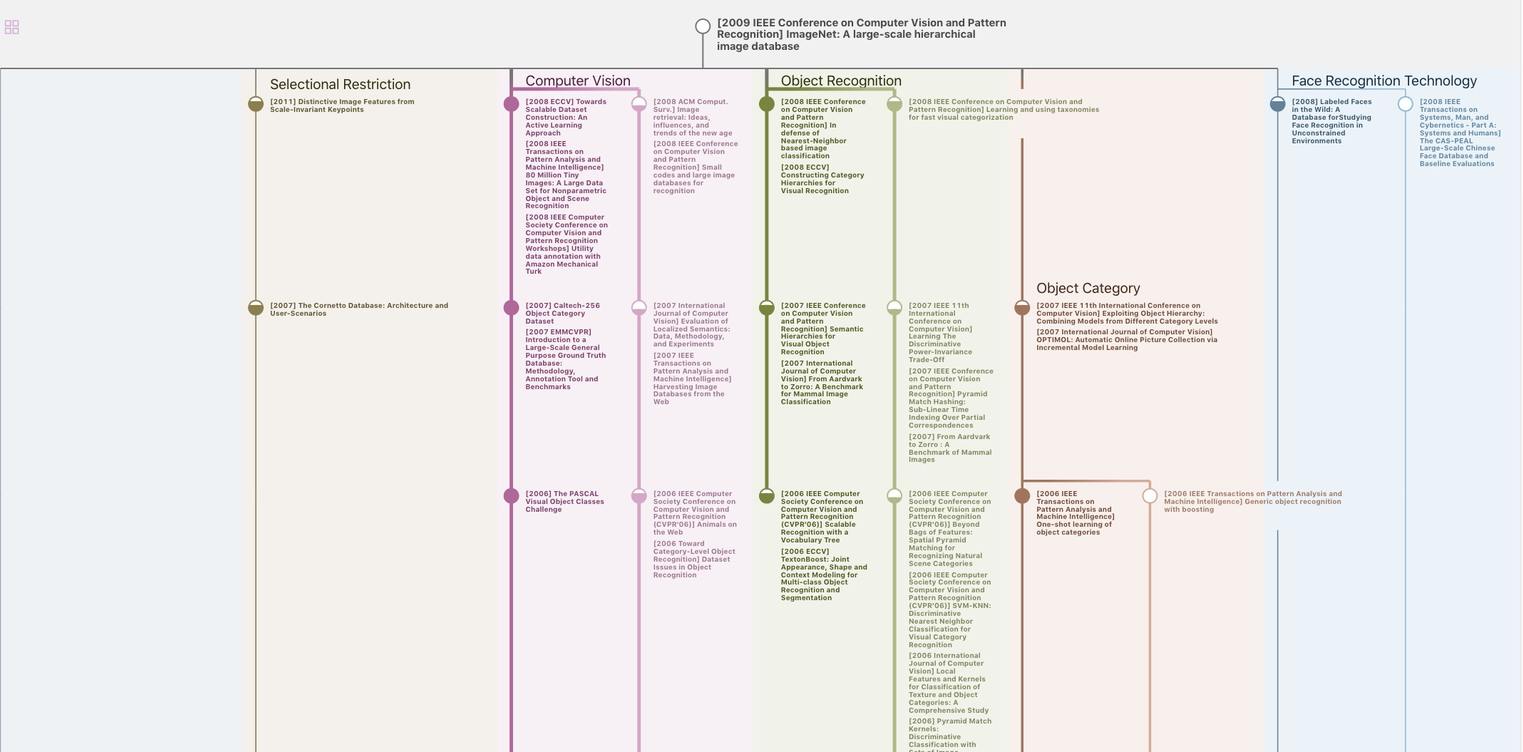
生成溯源树,研究论文发展脉络
Chat Paper
正在生成论文摘要