Solute transport prediction in heterogeneous porous media using random walks and machine learning
GEM - International Journal on Geomathematics(2023)
摘要
Solute transport processes in heterogeneous porous media have been traditionally studied through the parameterization of macroscale properties using upscaling approaches over a representative elementary volume. As a result, our ability to accurately model solute transport at fine-scale is limited. Combining multiple transport and geometrical observations from the pore scale in a multiphysics framework can enhance the understanding of transport mechanisms that manifest at larger scales. In this paper, we predict conservative solute transport in three sandstone geometries (Castlegate, Bentheimer, and sandpack) that range across different degrees of heterogeneity using a machine learning approach. Our approach, which is based on the random forests (RF) algorithm, performs simulated transport predictions such as solute breakthrough curves. The RF algorithm used in our workflow is a tree-based ensemble method, which builds several different decision tree models independently and then computes a final prediction by combining the outputs of the individual trees. We employ observations, such as solute arrival time and distance traveled, as input to train the predictive model using random walk particle tracking (RWPT) simulations in the sandstones. We employ Bayesian optimization techniques to select the hyperparameter values controlling the structure of the RF model in order to avoid overfitting. Results of our workflow show accurate RF predictions of the RWPT breakthrough curves demonstrating the ability of the RF algorithm to capture the critical flow and transport properties of porous media. We also examine the sensitivity to geometrical sample effects in the training data, which can impact machine learning predictions. The RF algorithm used is able to provide accurate results in real rock samples spanning from unconsolidated granular to consolidated media, highlighting the ability of the model to generalize solute transport problems in porous media.
更多查看译文
关键词
Machine learning, Random forest algorithm, Random walks model, Solute transport, Anomalous transport, Heterogeneous porous media
AI 理解论文
溯源树
样例
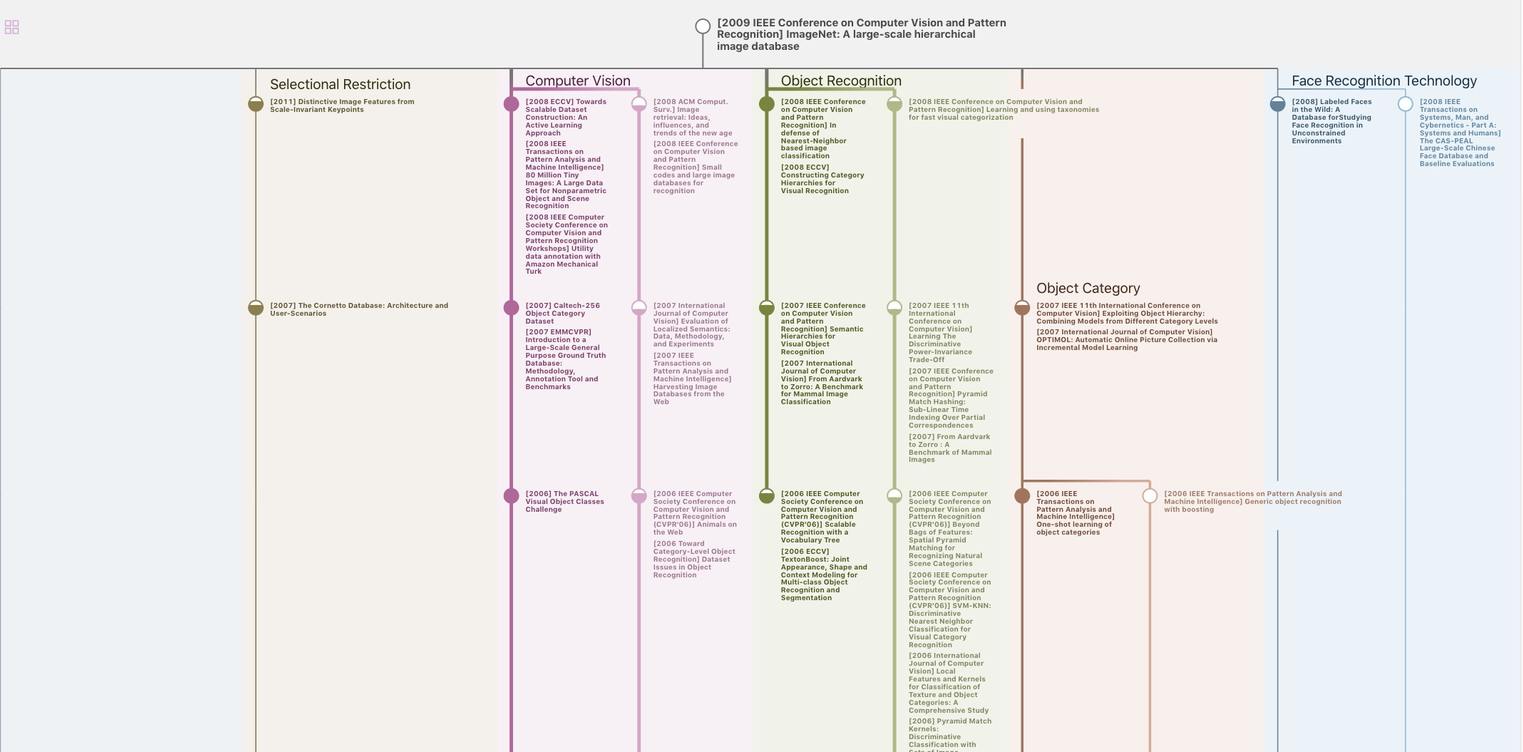
生成溯源树,研究论文发展脉络
Chat Paper
正在生成论文摘要