Robust Calibration of Vehicle Solid-State Lidar-Camera Perception System Using Line-Weighted Correspondences in Natural Environments.
IEEE TRANSACTIONS ON INTELLIGENT TRANSPORTATION SYSTEMS(2024)
摘要
With the rapid development of autonomous driving and SLAM technology, the perception system of a vehicle heavily relies on laser and image sensors to capture the real-world scenario and avoid obstacles autonomously. To achieve accurate and robust multi-sensor fusion computation, high-precision extrinsic calibration of camera and laser scanner is a necessary requirement. Traditional multi-sensor calibration methods based on manual features rely on specific scenarios and may not provide feature information over long distances. In this paper, we present a novel approach for robustly calibrating the extrinsic parameters of a solid-state(SS) lidar-camera system in a natural environment. Our proposed method begins with obtaining robust line feature information. we first innovatively employ a super-voxel clustering method to extract global 3D line features from the complete point cloud and then back-project these 3D line features into 2D space. Afterward, a transformer-based edge detection network, EDTER, is used to detect the edge features and estimate the probability pixel-by-pixel. To consider the uncertainty of two-dimensional line features and the inconsistency of residuals at different distances, we construct a line feature weight model for line feature residual calculation. Finally, we minimize the residual errors using least squares optimization to recover the relative pose of the camera and the lidar sensor. We conducted a performance study to compare our proposed method against existing targetless calibration methods on various natural scenarios. The experimental results demonstrate that our proposed method achieves higher robustness, accuracy, and consistency, making it suitable for real-world applications.
更多查看译文
关键词
Calibration,LiDAR-camera,least square,unmanned vehicles,line feature
AI 理解论文
溯源树
样例
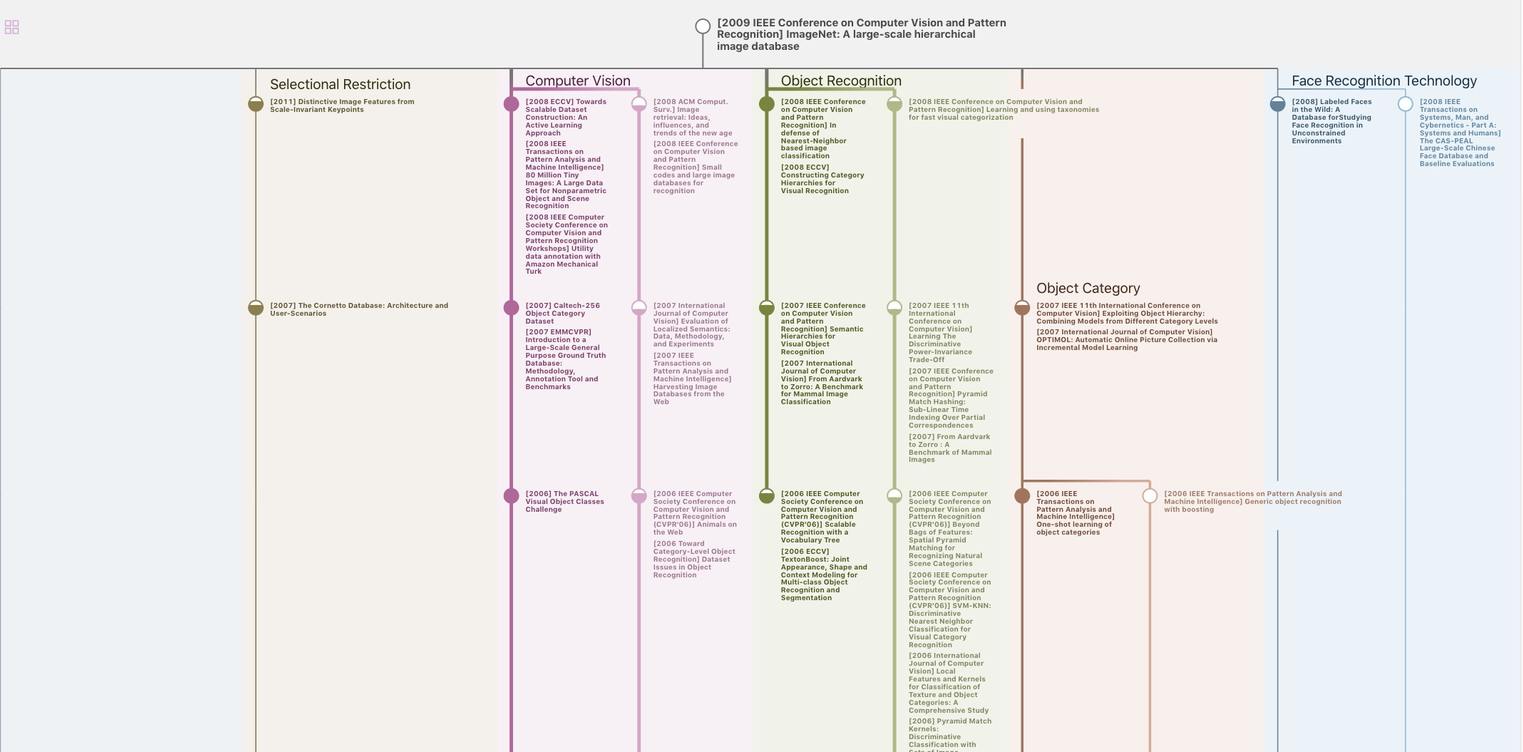
生成溯源树,研究论文发展脉络
Chat Paper
正在生成论文摘要