LEPS: A lightweight and effective single-stage detector for pothole segmentation
IEEE Sensors Journal(2023)
摘要
Currently, the problem of potholes on urban roads is becoming increasingly severe. Identifying and locating road potholes promptly has become a major challenge for urban construction. Therefore, we proposed a lightweight and effective instance segmentation method called LEPS (Lightweight and Effective Pothole Segmentation Detector) for road pothole detection. To extract the image edge information and gradient information of potholes from the feature map more efficiently, we proposed a module that performs the convolutional super-position supplemented with a convolutional kernel to enhance spatial details for the backbone. We have designed a novel module applied to the Neck layer, improving the detection performance while reducing the parameters. To enable accurate segmentation of fine-grained features, we optimized the ProtoNet, which enables the segmentation head to generate high-quality masks for more accurate prediction. We have fully demonstrated the effectiveness of the method through a large number of comparative experiments. Our detector has excellent performance on two authoritative example datasets, URTIRI and COCO, and can successfully be applied to visual sensors to accurately detect and segment road potholes in real environments, accurately LEPS reached 0.892 and 0.648 in terms of Mask for AP50 and AP50:95, which is improved by 4.6% and 20.6% compared to the original model. These results demonstrate its strong competitiveness when compared to other models. Comprehensively, LEPS improves detection accuracy while maintaining lightweight, which allows the model to meet the practical application requirements of edge computing devices.
更多查看译文
关键词
Pothole Detection,Instance Segmentation,Lightweight,Deep Learning,Edge Computing
AI 理解论文
溯源树
样例
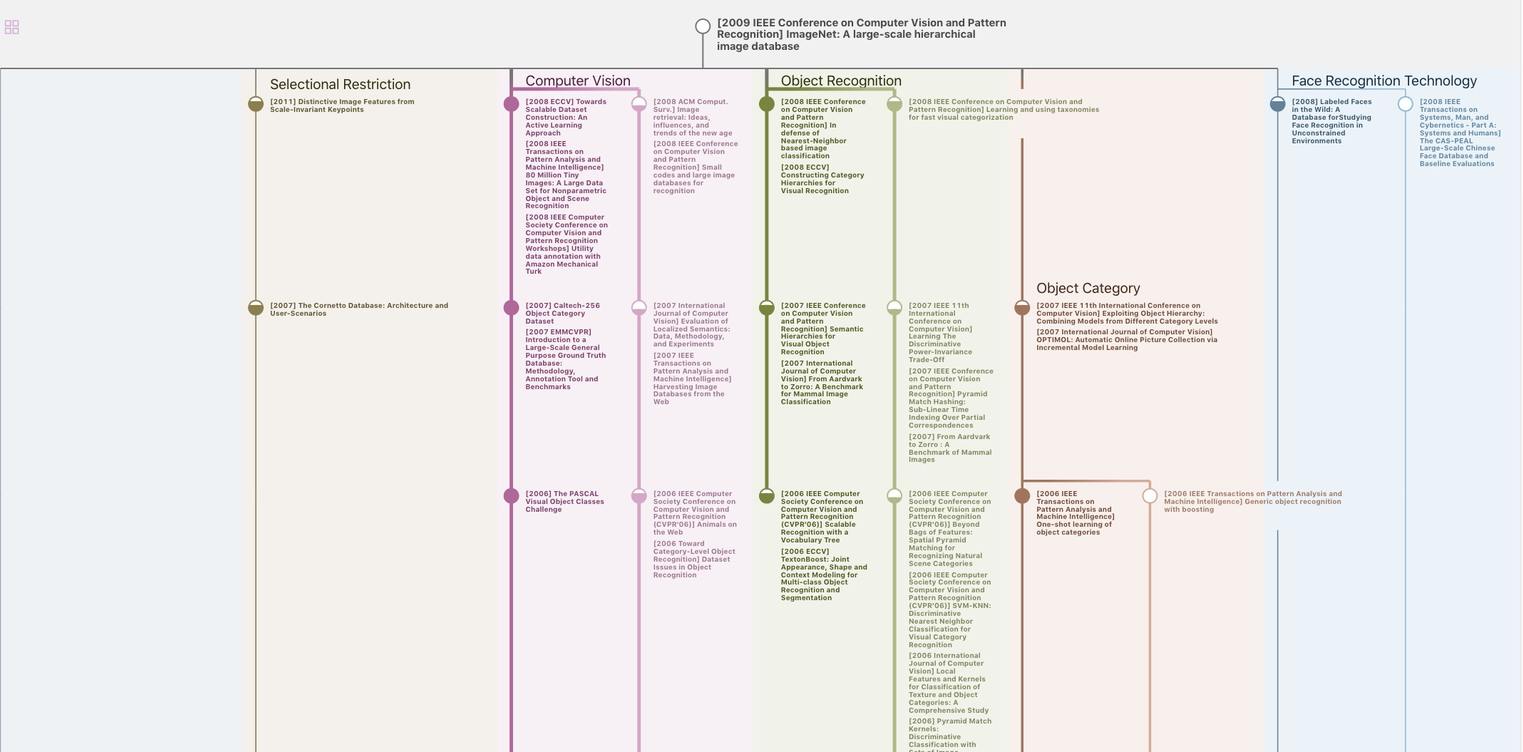
生成溯源树,研究论文发展脉络
Chat Paper
正在生成论文摘要