OxiTidy V.1: Motion Artifact Detection in Photoplethysmographic Signals Using Artificial Neural Network.
Congresso Brasileiro de Automática(2022)
摘要
Pulse oximetry is a non-invasive technique that allows the monitoring of physiological parameters in a very simplified way from photoplethysmographic (PPG) signals. What was possible to do only with high-cost and unwieldy biomedical equipment, has been popularized with the emergence of wearable devices. However, the way these devices are built and used directly influences the quality of the information provided to the user. PPG signals are susceptible to noise, which is largely caused by user movement during monitoring. This can cause errors in the readings and false alarms. In order to mitigate these undesirable effects, this paper proposes an algorithm called OxiTidy v.1 based on artifical neural network (ANN) capable of detecting noise in the PPG signals. These affected samples were not used to the measurements computation, instead a linear interpolation between two normal measurements of oxygen saturation (SpO2) or heart rate (HR) was performed. The algorithms proposed in this work were tested in the prototype of a Wi-Fi pulse oximeter developed at the Federal University of São Carlos (UFSCar). The results indicated that an ANN (3-3-1) based on multilayer perceptron (MLP) was able to improve SpO2 and HR estimations both at rest and in motion. OxiTidy v.1 identified the intervals where the measurements were incorrect and then interpolated new values with a good approximation to the readings performed by a pulse oximeter certified by Anvisa.
更多查看译文
AI 理解论文
溯源树
样例
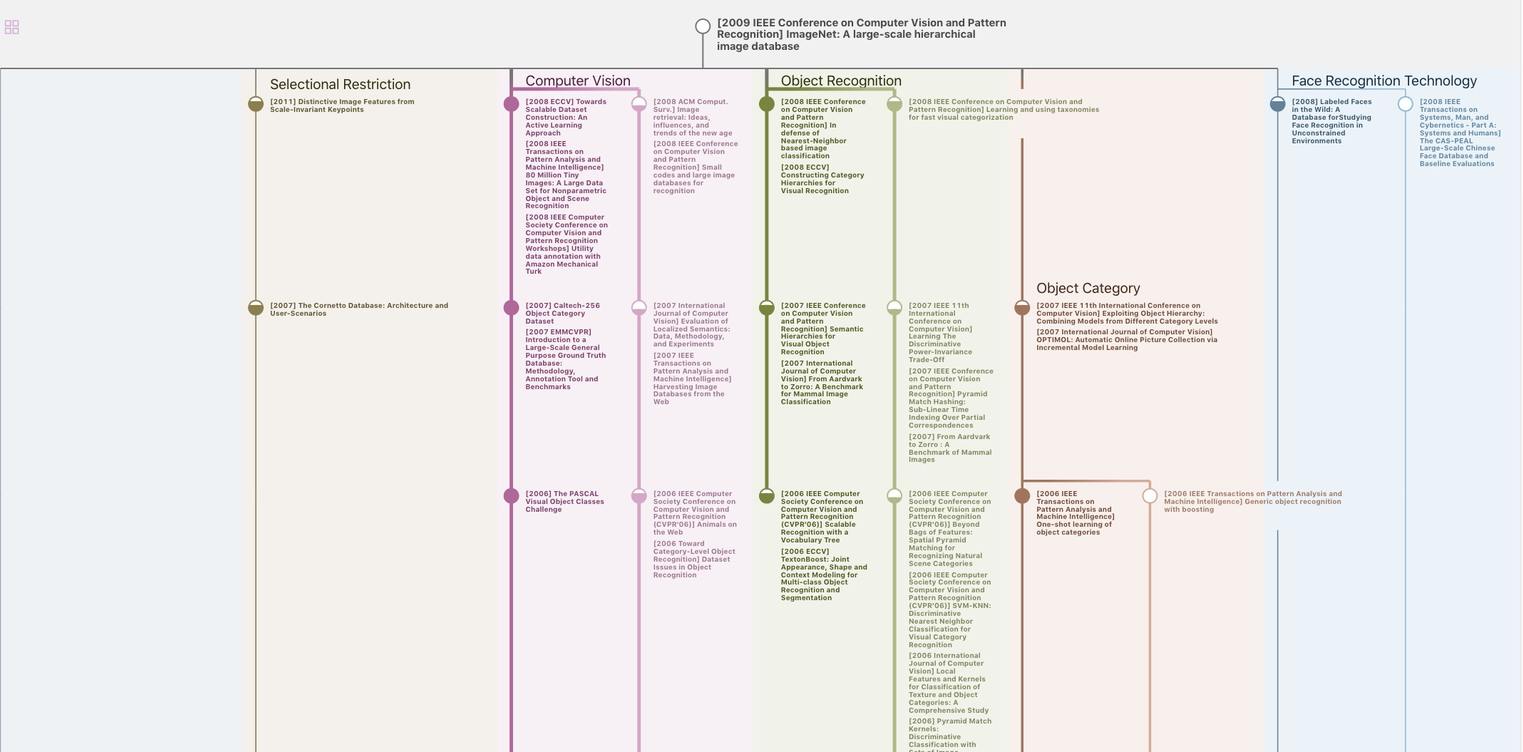
生成溯源树,研究论文发展脉络
Chat Paper
正在生成论文摘要