Fragility Fracture of Pelvis Prediction from Computed Tomography Using Boring Survey and Convolutional Neural Network
JOURNAL OF ADVANCED COMPUTATIONAL INTELLIGENCE AND INTELLIGENT INFORMATICS(2023)
摘要
Fragility fracture of pelvis (FFP) is increasingly affecting elderly population. Although computed tomography (CT) imaging is considered superior to conventional radiographic image for diagnosing FFP, clinicians face challenges in recognizing pelvic fractures owing to imaging contrast or feature size. This study proposes a method that combines boring survey based FFP candidate extraction from CT images and a newly developed convolutional neural network model. In addition, the proposed method also visualizes the probability of fracture on 3D bone surface data. The accuracy, precision, and recall of the proposed method were found to be 79.7%, 60.0%, and 80.6%, respectively. Furthermore, the 3D view of fracture probability on the pelvic bone surface allows for qualitative assessment and can support physicians to diagnose FFP. The findings indicate that the proposed method has potential for predicting FFP.
更多查看译文
关键词
FFP,boring survey,CT,CNN
AI 理解论文
溯源树
样例
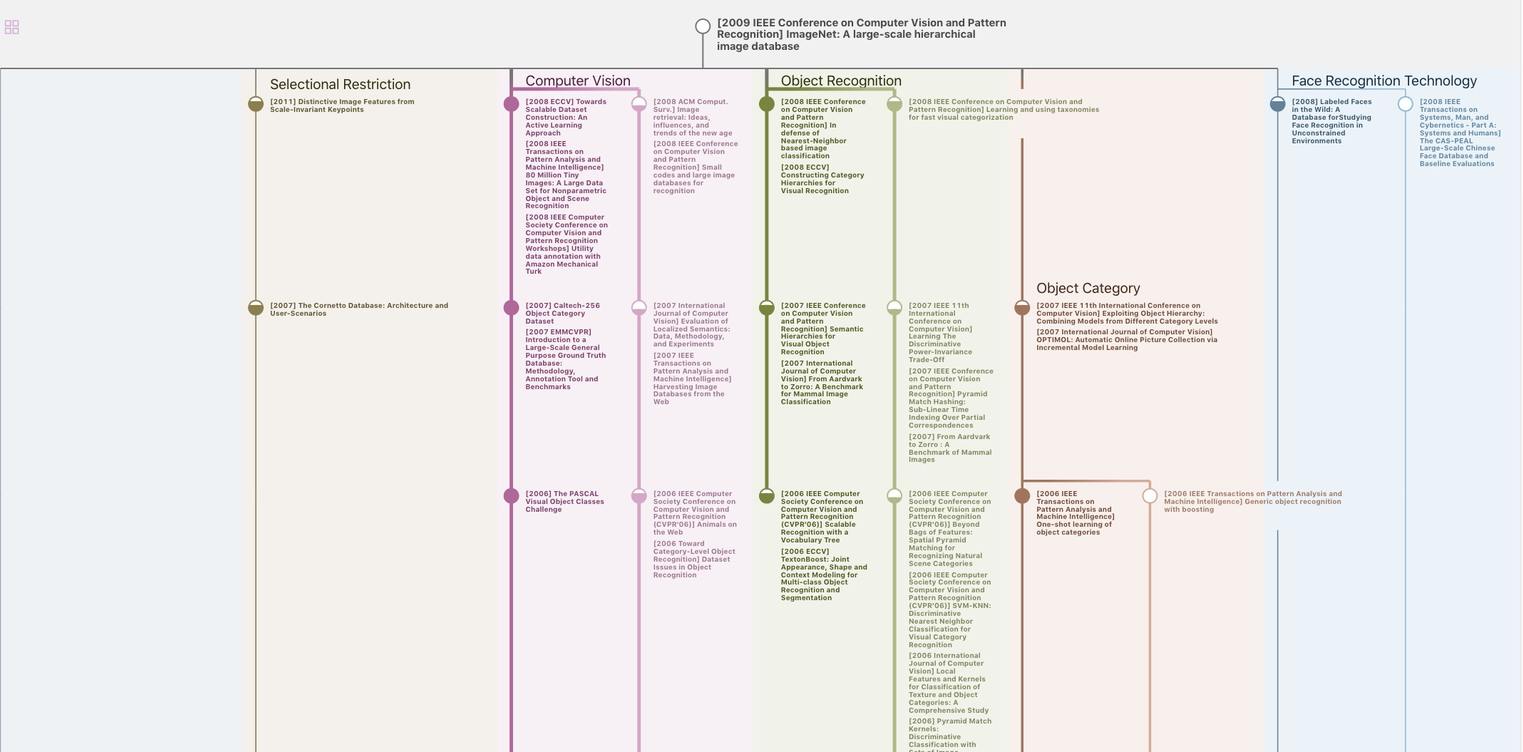
生成溯源树,研究论文发展脉络
Chat Paper
正在生成论文摘要