A Comparison of Client Weighting Schemes in Federated Learning
Lecture notes in networks and systems(2023)
摘要
Data is the new oil of the digital economy. Many business organizations are gathering and using their data to optimize their business performance. However, in some cases, an individual organization may not have a sufficient amount of data or data quality to build a well-performing model, especially in a dynamic environment. In some cases, the companies, which collect similar data, may be willing to exchange their knowledge, yet without sharing their data, e.g. due to privacy or legal issues. In such a case, federated learning is a solution. In horizontal federated learning, each client (organization) iteratively improves its model, so that it can be regularly aggregated and shared with all clients participating in the federation for further improvements. In the aggregation mechanism based on the weighted average, weights depend on the model’s quality for each client. In this paper, we extend our previous approach to federated learning with missing information, and we investigate different weighting schemes, that depend on the effectiveness of the local models. The initial results are promising.
更多查看译文
关键词
federated learning,client weighting schemes
AI 理解论文
溯源树
样例
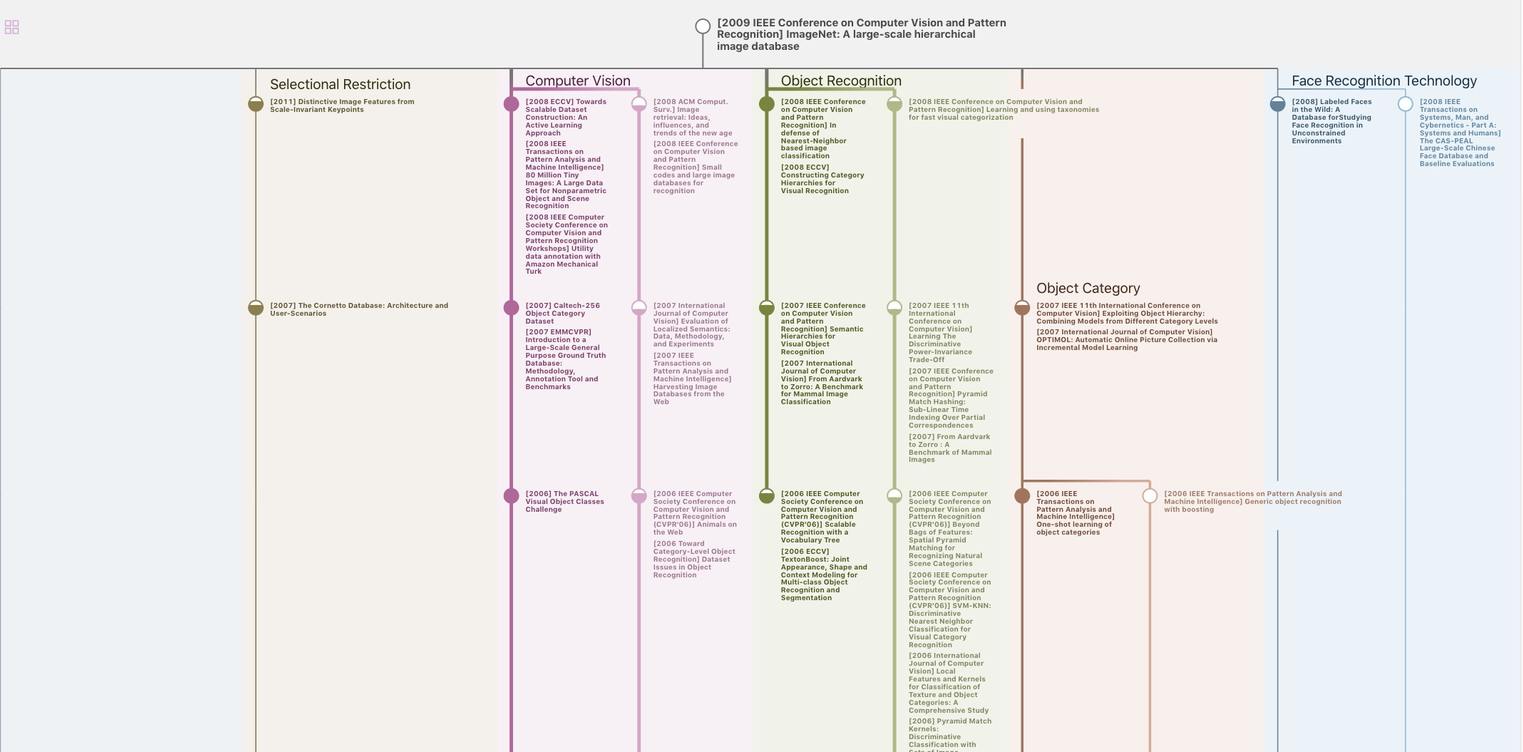
生成溯源树,研究论文发展脉络
Chat Paper
正在生成论文摘要