Integration of Adaptive Control and Reinforcement Learning for Real-Time Control and Learning
IEEE TRANSACTIONS ON AUTOMATIC CONTROL(2023)
摘要
This article considers the problem of real-time control and learning in dynamic systems subjected to parameteric uncertainties. We propose a combination of a reinforcement learning (RL)-based policy in the outer loop suitably chosen to ensure stability and optimality for the nominal dynamics, together with adaptive control (AC) in the inner loop so that in real-time AC contracts the closed-loop dynamics toward a stable trajectory traced out by RL. In total, two classes of nonlinear dynamic systems are considered, both of which are control affine. The first class of dynamic systems utilizes equilibrium points and a Lyapunov approach, whereas second class of nonlinear systems uses contraction theory. AC-RL controllers are proposed for both classes of systems and shown to lead to online policies that guarantee stability using a high-order tuner and accommodate parameteric uncertainties and magnitude limits on the input. In addition to establishing a stability guarantee with real-time control, the AC-RL controller is also shown to lead to parameter learning with persistent excitation for the first class of systems. Numerical validations of all algorithms are carried out using a quadrotor landing task on a moving platform.
更多查看译文
关键词
Adaptive control,parameter learning,persistent excitation,reinforcement learning,stability
AI 理解论文
溯源树
样例
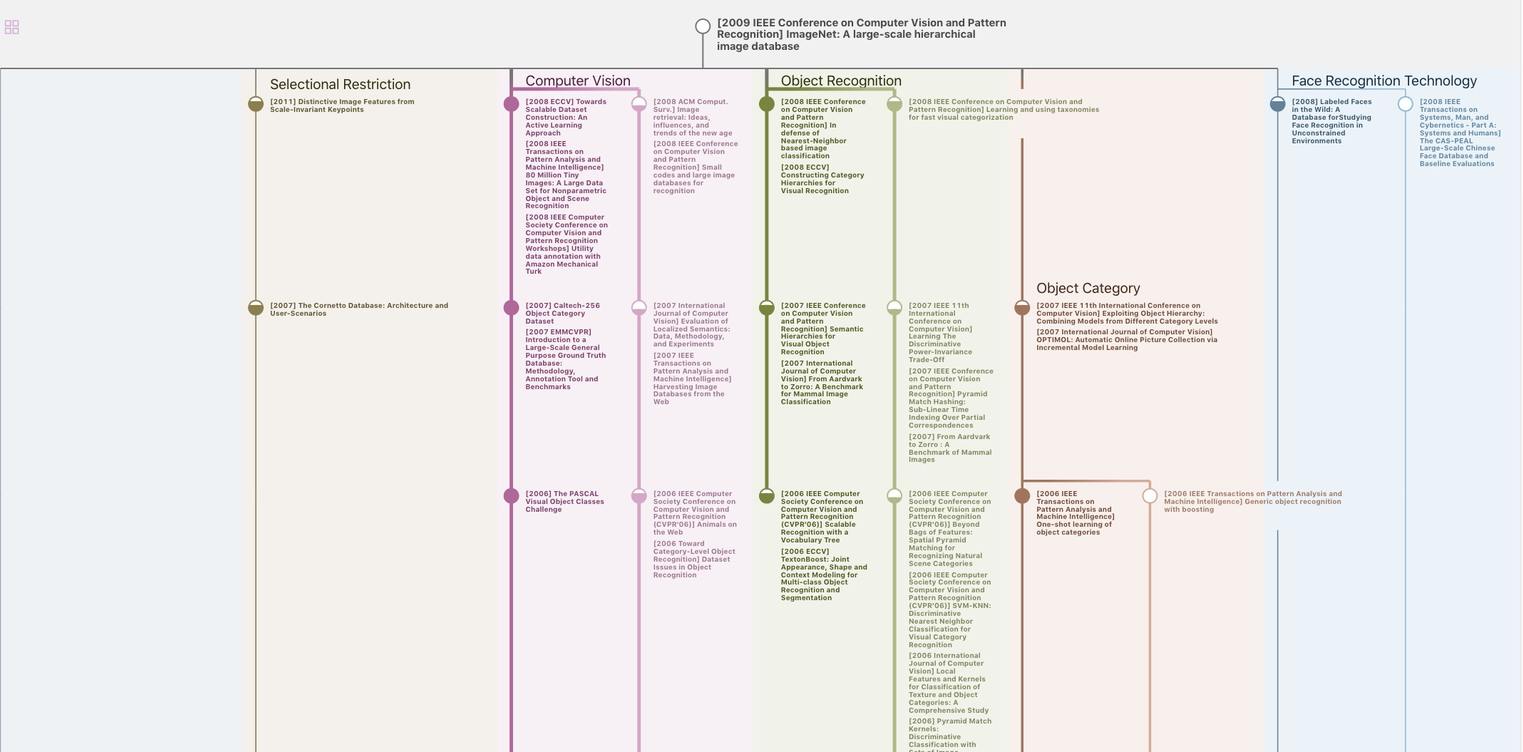
生成溯源树,研究论文发展脉络
Chat Paper
正在生成论文摘要