Stochastic MPC With Robustness to Bounded Parameteric Uncertainty
IEEE TRANSACTIONS ON AUTOMATIC CONTROL(2023)
摘要
In this article, we present a stochastic model predictive control approach for discrete-time LTI systems subject to bounded parameteric uncertainty and potentially unbounded stochastic additive noise. The proposed scheme makes use of homothetic tubes along the prediction horizon for a robust treatment of parameteric uncertainty. Stochastic noise is handled by tightening constraints using the concept of probabilistic reachable sets (PRS), which are typically constructed offline by exploiting noise distribution information. In order to address the presence of additional parameteric uncertainty, we introduce a strategy for generating "robustified" PRS based only on the first and second moments of the noise sequence. In the case of quadratic cost functions, and under a further independent identically distributed assumption on the noise distribution, we also provide an average asymptotic performance bound for the l2-norm of the closed-loop state. Finally, the proposed approach is demonstrated in both an illustrative example, and for a building temperature control problem.
更多查看译文
关键词
Bounded parameteric uncertainty,chance constraints,stochastic model predictive control (SMPC)
AI 理解论文
溯源树
样例
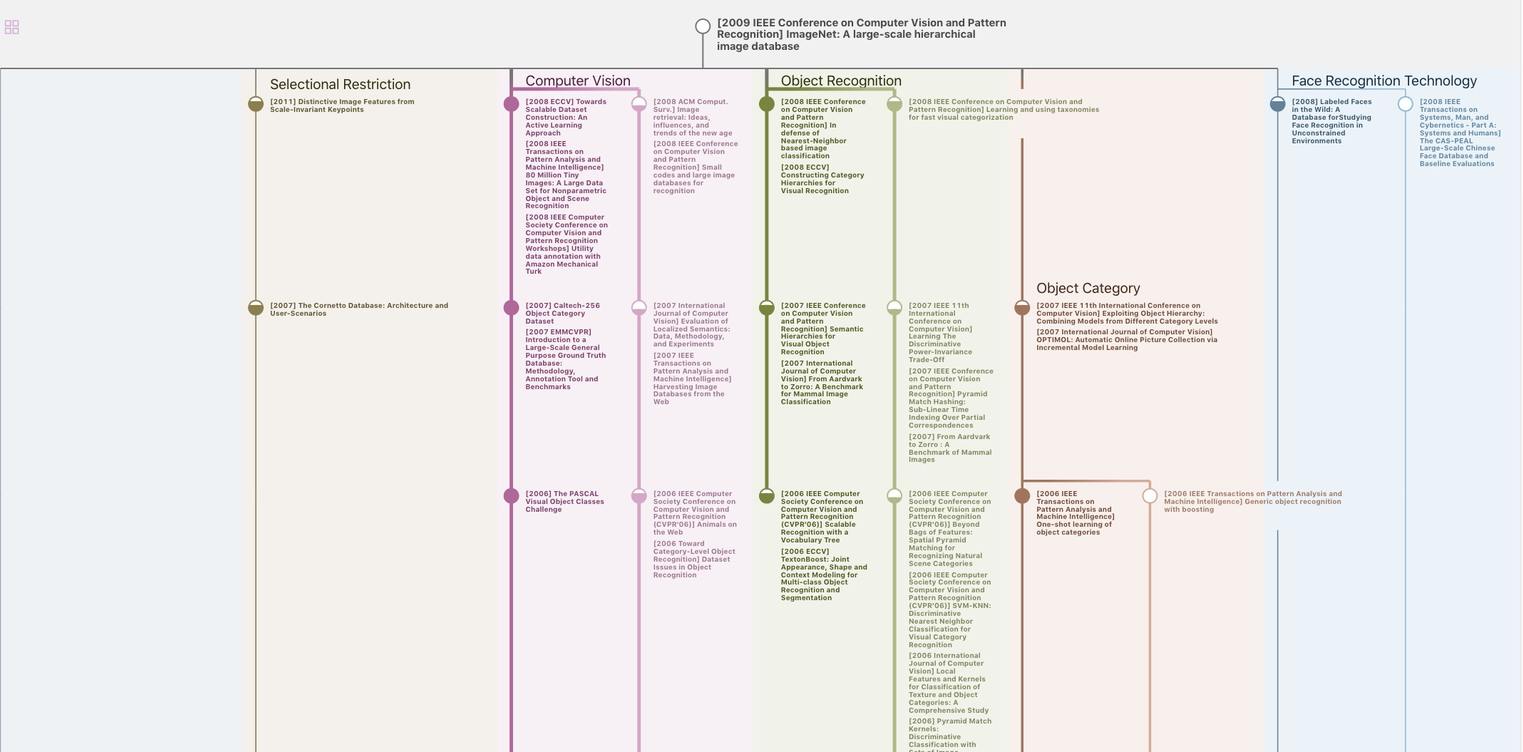
生成溯源树,研究论文发展脉络
Chat Paper
正在生成论文摘要