Classification of breast ultrasound images in BI-RADS categories using binary decomposition strategies with convolutional neural networks.
2023 20th International Conference on Electrical Engineering, Computing Science and Automatic Control (CCE)(2023)
摘要
The Breast Imaging Reporting & Data System (BI-RADS) assesses breast findings in terms of categories of risk of malignancy from which the clinical conduct of patients is determined. In particular, categories 2 and 3 are related to benign tumors, recommending routine imaging studies. In contrast, categories 4 and 5 are associated with suspicious tumors analyzed by biopsy exam to determine the pathology. Recently, this multiclass classification problem has been addressed by a single convolutional neural network (CNN) model that provides a posterior probability to each BI-RADS category. Nevertheless, multiclass classification can be improved by using binary decomposition strategies that map a multiclass problem on several two-class problems. This paper compares three binary decomposition strategies applied to breast ultrasound (BUS) image classification: one-versus-all (OVA), one-versus-one (OVO), and all-and-one (A&O), where the CNN Xception model is used as a base learner. Five BI-RADS classes are considered: categories 2, 3, and 4 in benign tumors and categories 4 and 5 in malignant tumors. The results show that the OVA strategy presents the best classification performance regarding OVO and A&O with a general accuracy of 60%, while a single CNN model reaches 57%. Besides, OVA obtains a sensitivity and specificity of 0.80 and 0.93, while a single CNN attains 0.77 and 0.94. Hence, dividing the multiclass classification problem into simpler binary classification sub-problems improves BUS classification.
更多查看译文
关键词
breast ultrasound,BI-RADS,tumor classification,binary decomposition strategy,convolutional neural network
AI 理解论文
溯源树
样例
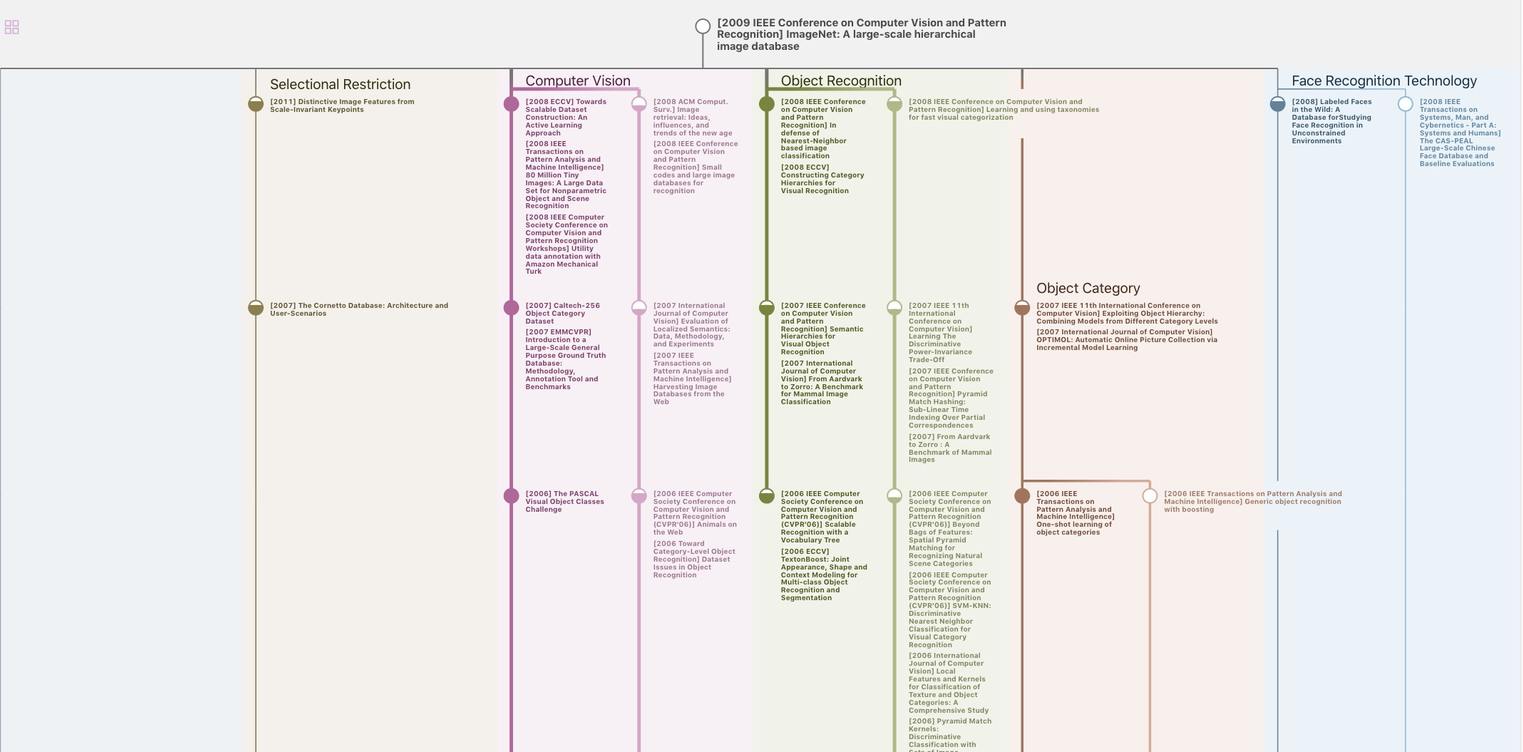
生成溯源树,研究论文发展脉络
Chat Paper
正在生成论文摘要