Evolutionary prototype selection for multi-output regression
Neurocomputing(2019)
摘要
A novel approach to prototype selection for multi-output regression data sets is presented. A multi-objective evolutionary algorithm is used to evaluate the selections using two criteria: training data set compression and prediction quality expressed in terms of root mean squared error. A multi-target regressor based on k-NN was used for that purpose during the training to evaluate the error, while the tests were performed using four different multi-target predictive models. The distance matrices used by the multi-target regressor were cached to accelerate operational performance. Multiple Pareto fronts were also used to prevent overfitting and to obtain a broader range of solutions, by using different probabilities in the initialization of populations and different evolutionary parameters in each one. The results obtained with the benchmark data sets showed that the proposed method greatly reduced data set size and, at the same time, improved the predictive capabilities of the multi-output regressors trained on the reduced data set.
更多查看译文
关键词
evolutionary prototype selection,regression,multi-output
AI 理解论文
溯源树
样例
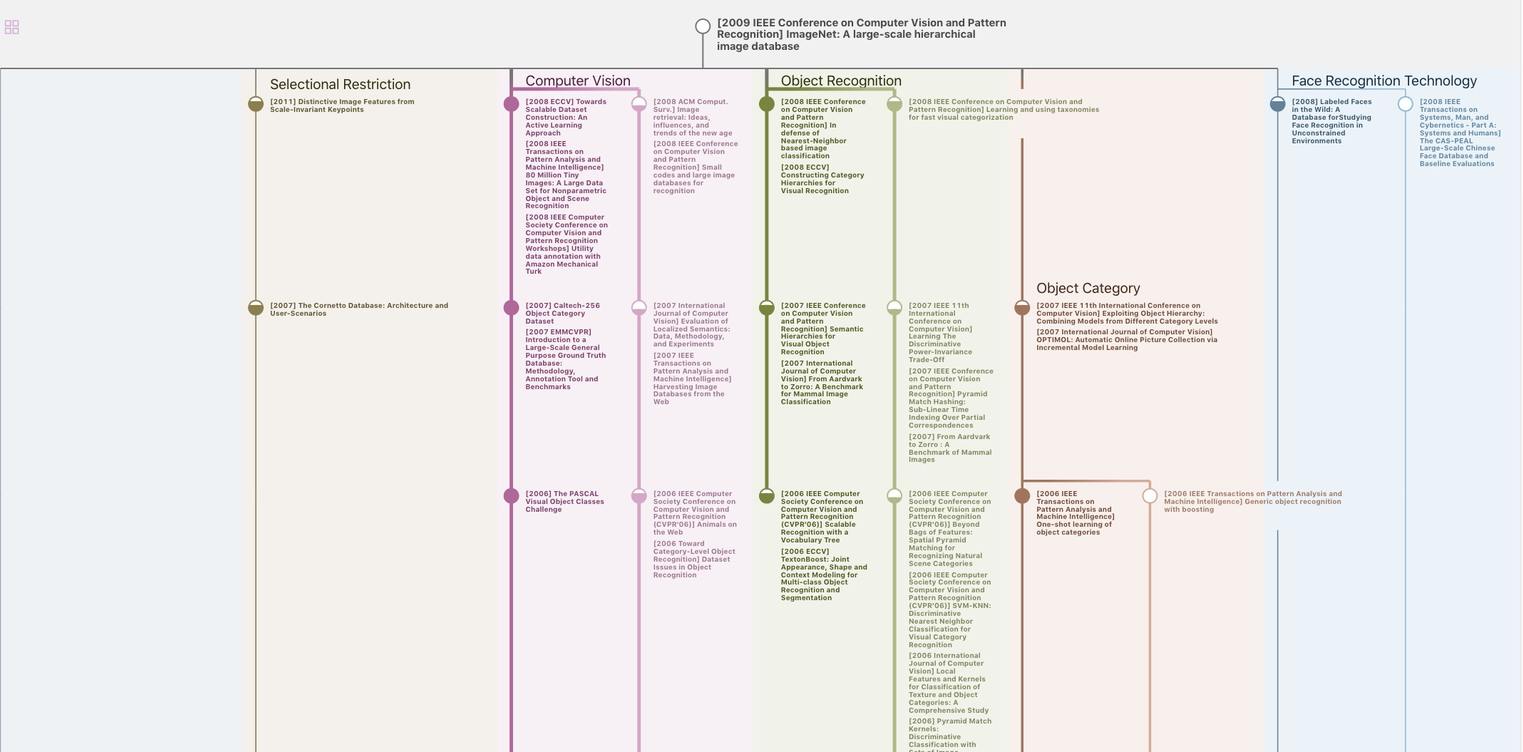
生成溯源树,研究论文发展脉络
Chat Paper
正在生成论文摘要