On the Role of the Action Space in Robot Manipulation Learning and Sim-to-Real Transfer
IEEE Robotics and Automation Letters(2023)
摘要
We study the choice of action space in robot manipulation learning and
sim-to-real transfer. We define metrics that assess the performance, and
examine the emerging properties in the different action spaces. We train over
250 reinforcement learning (RL) agents in simulated reaching and pushing tasks,
using 13 different control spaces. The choice of spaces spans combinations of
common action space design characteristics. We evaluate the training
performance in simulation and the transfer to a real-world environment. We
identify good and bad characteristics of robotic action spaces and make
recommendations for future designs. Our findings have important implications
for the design of RL algorithms for robot manipulation tasks, and highlight the
need for careful consideration of action spaces when training and transferring
RL agents for real-world robotics.
更多查看译文
关键词
Reinforcement Learning,Machine Learning for Robot Control,Deep Learning in Grasping and Manipulation
AI 理解论文
溯源树
样例
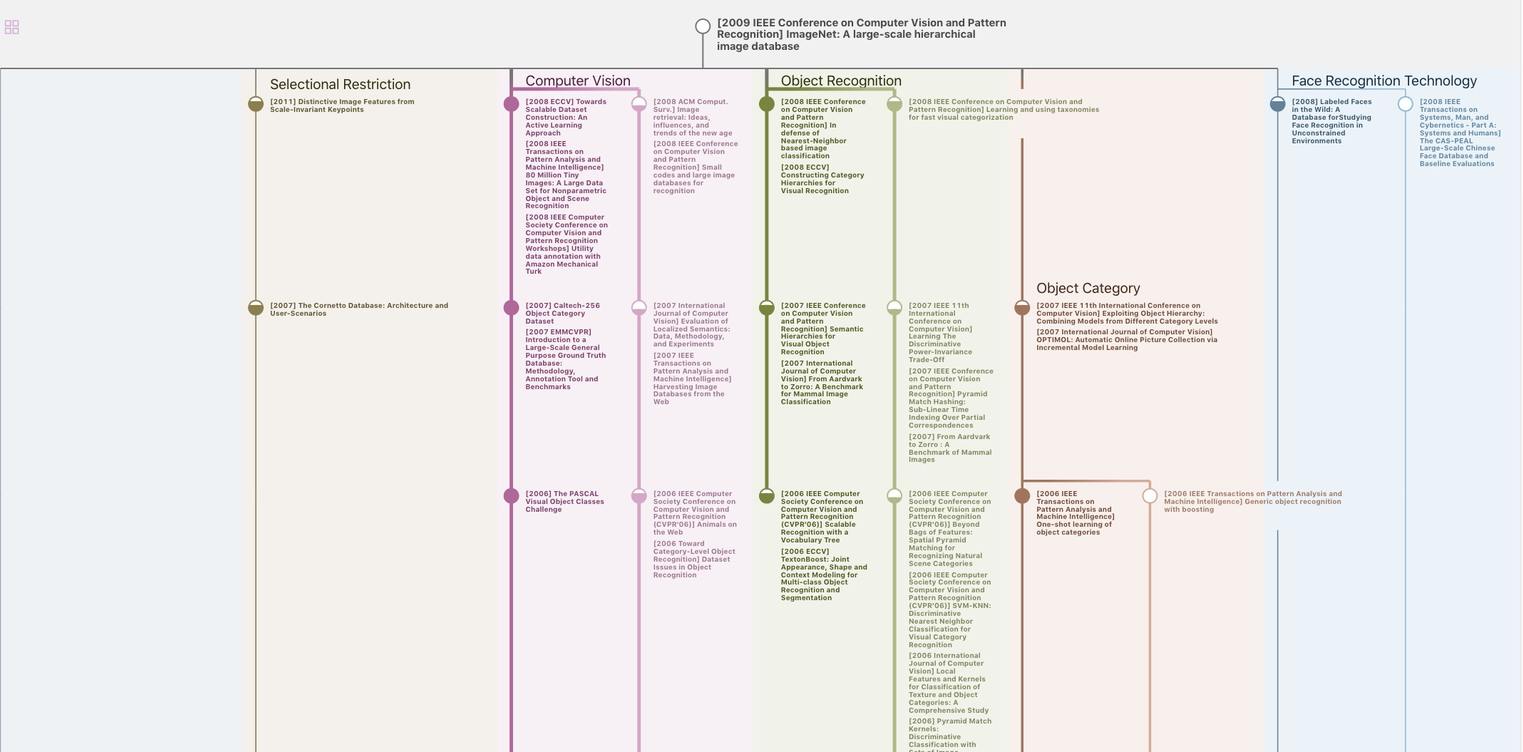
生成溯源树,研究论文发展脉络
Chat Paper
正在生成论文摘要