ProSpect: Prompt Spectrum for Attribute-Aware Personalization of Diffusion Models
ACM TRANSACTIONS ON GRAPHICS(2023)
摘要
Personalizing generative models offers a way to guide image generation with user-provided references. Current personalization methods can invert an object or concept into the textual conditioning space and compose new natural sentences for text-to-image diffusion models. However, representing and editing specific visual attributes such as material, style, and layout remains a challenge, leading to a lack of disentanglement and editability. To address this problem, we propose a novel approach that leverages the step-by-step generation process of diffusion models, which generate images from low to high frequency information, providing a new perspective on representing, generating, and editing images. We develop the Prompt Spectrum Space P*, an expanded textual conditioning space, and a new image representation method called ProSpect. ProSpect represents an image as a collection of inverted textual token embeddings encoded from per-stage prompts, where each prompt corresponds to a specific generation stage (i.e., a group of consecutive steps) of the diffusion model. Experimental results demonstrate that P* and ProSpect offer better disentanglement and controllability compared to existing methods. We apply ProSpect in various personalized attribute-aware image generation applications, such as image-guided or text-driven manipulations of materials, style, and layout, achieving previously unattainable results from a single image input without fine-tuning the diffusion models. Our source code is available at https: //github.com/zyxElsa/ProSpect.
更多查看译文
关键词
Image generation,Diffusion models,Attribute-aware editing,Model personalization
AI 理解论文
溯源树
样例
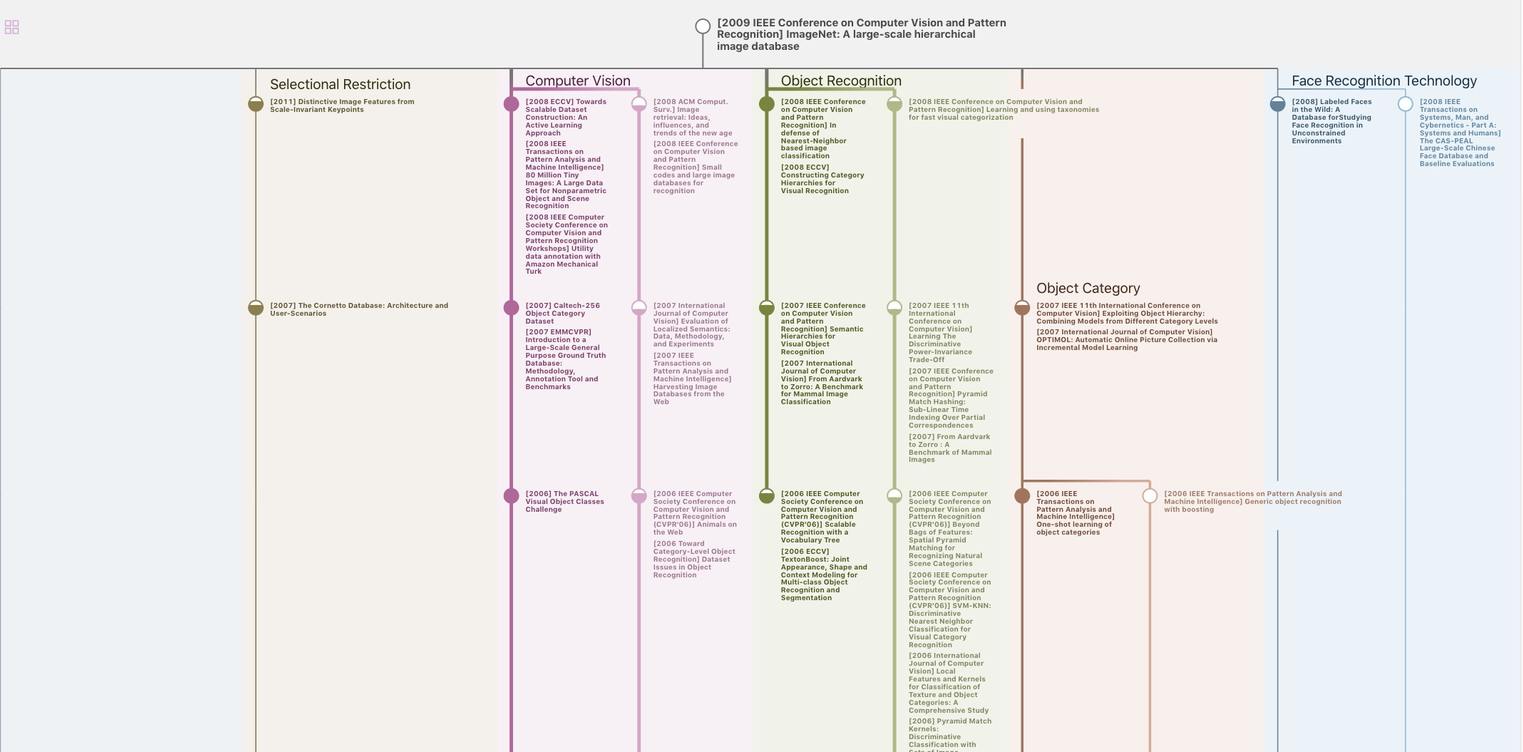
生成溯源树,研究论文发展脉络
Chat Paper
正在生成论文摘要