GeoLatent: A Geometric Approach to Latent Space Design for Deformable Shape Generators
ACM TRANSACTIONS ON GRAPHICS(2023)
摘要
We study how to optimize the latent space of neural shape generators that map latent codes to 3D deformable shapes. The key focus is to look at a deformable shape generator from a differential geometry perspective. We define a Riemannian metric based on as-rigid-as-possible and as-conformal-as-possible deformation energies. Under this metric, we study two desired properties of the latent space: 1) straight-line interpolations in latent codes follow geodesic curves; 2) latent codes disentangle pose and shape variations at different scales. Strictly enforcing the geometric interpolation property, however, only applies if the metric matrix is a constant. We show how to achieve this property approximately by enforcing that geodesic interpolations are axis-aligned, i.e., interpolations along coordinate axis follow geodesic curves. In addition, we introduce a novel approach that decouples pose and shape variations via generalized eigendecomposition. We also study efficient regularization terms for learning deformable shape generators, e.g., that promote smooth interpolations. Experimental results on benchmark datasets show that our approach leads to interpretable latent codes, improves the generalizability of synthetic shapes, and enhances performance in geodesic interpolation and geodesic shooting.
更多查看译文
关键词
generative model,Riemannian geometry
AI 理解论文
溯源树
样例
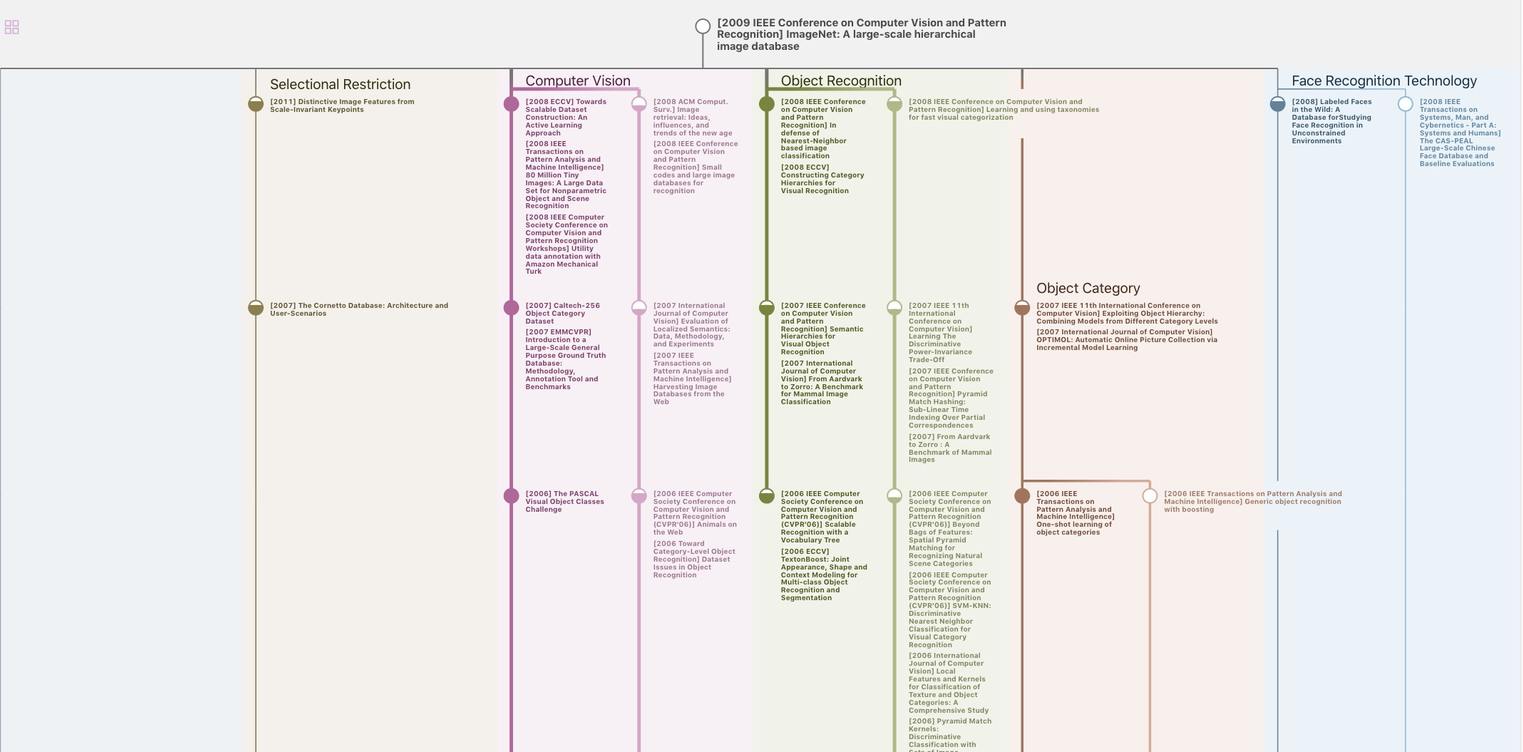
生成溯源树,研究论文发展脉络
Chat Paper
正在生成论文摘要