Self-Supervised Temporal Consistency applied to Domain Adaptation in Semantic Segmentation of Urban Scenes
2023 LATIN AMERICAN ROBOTICS SYMPOSIUM, LARS, 2023 BRAZILIAN SYMPOSIUM ON ROBOTICS, SBR, AND 2023 WORKSHOP ON ROBOTICS IN EDUCATION, WRE(2023)
摘要
Deep Learning-based Semantic Segmentation has reached remarkable levels of accuracy throughout the years. Nonetheless, applying pretrained models to new domains-even though related-leads to considerable drops in performance due to domain shift. In addition to that, semantic labels are costly to obtain for new application scenarios, what makes the process of transfer learning even harder. Finally, since the majority of works in semantic segmentation rely on single-frame predictions, they miss a huge opportunity offered by reasoning on the temporal properties of video data. In light of that, this work addresses all previous problems by implementing a domain adaptation strategy based on self-supervised learning of temporal consistency in videos. The results show that our strategy greatly improves model precision and stability on a given unlabeled target domain.
更多查看译文
关键词
semantic segmentation,self-supervised learning,transfer learning,domain adaptation,temporal consistency
AI 理解论文
溯源树
样例
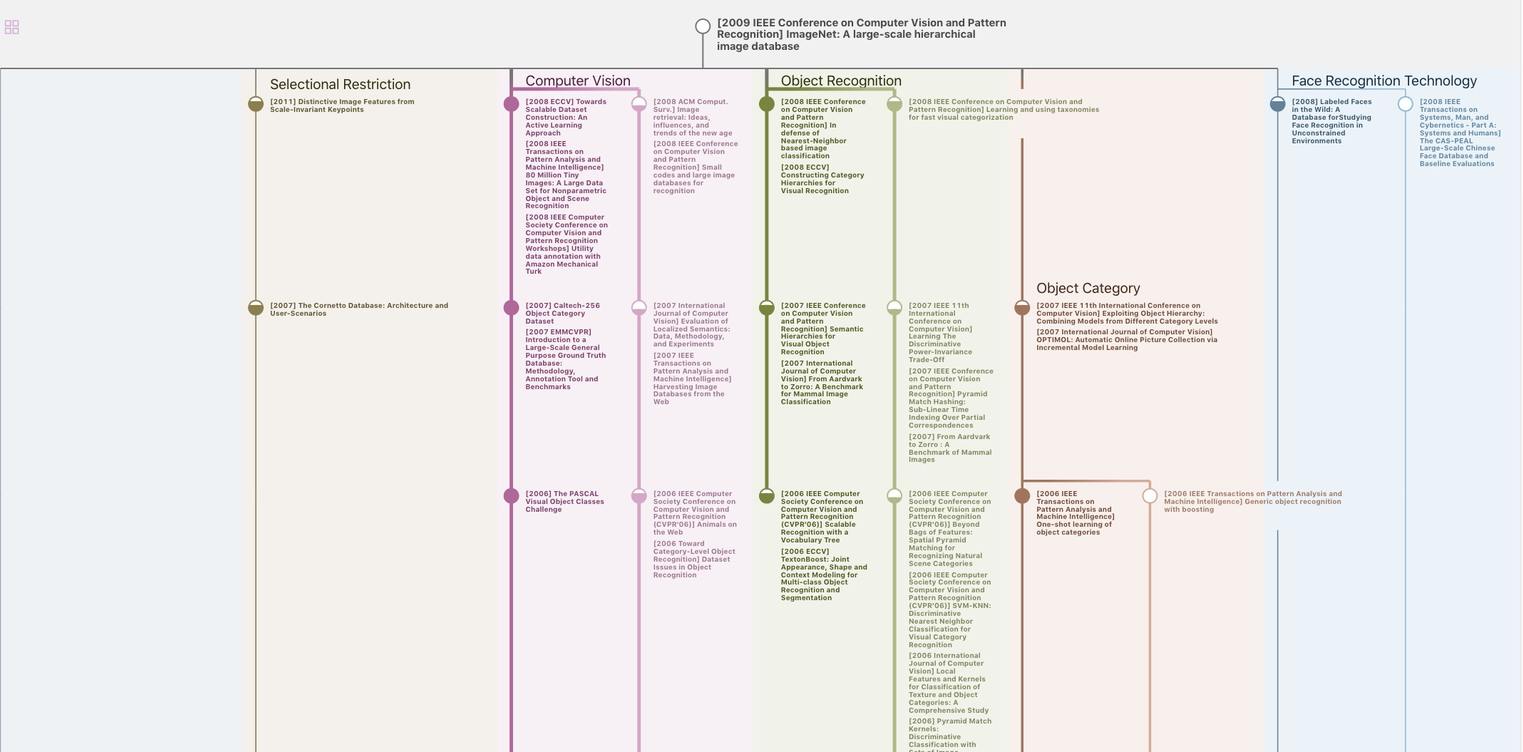
生成溯源树,研究论文发展脉络
Chat Paper
正在生成论文摘要