Nonnegative Preserving Projection for Dimensionality Reduction
2023 IEEE 6th International Conference on Pattern Recognition and Artificial Intelligence (PRAI)(2023)
摘要
Nonnegative matrix factorization (NMF) is a method that represent classical data based on matrix decomposition. NMF is more compatible with real-world applications and improves readability because the decomposed elements must be non-negative. The original data, which is very redundant and noisy, is directly decomposed using NMF. It is generally high-dimensional. This will waste a lot of time and the obtained result of decomposition is also not optimal. To obtain a low-dimensional compact representation of observational data, in which the hidden semantic information is uncovered and the partial structure information is simultaneously preserved, a novel dimensionality reduction framework named nonnegative preserving projection (NPP) is proposed. In NPP, we firstly construct an affinity graph for encoding the geometrical information needed to capture the data's local manifold. Then, the projection matrix is introduced into NMF. Meanwhile, we apply a PCA-based constraint to the objective function to ensure that the bulk of the energy from the original data is preserved. We examined the proposed NPP with existing approaches in different picture databases to verify the performance of NPP in dimensionality reduction. According to the findings of the experiments, the procedure is both feasible and effective.
更多查看译文
关键词
Nonnegative matrix factorization,Manifold learning,Nonnegative preserving projection (NPP),Dimensionality reduction
AI 理解论文
溯源树
样例
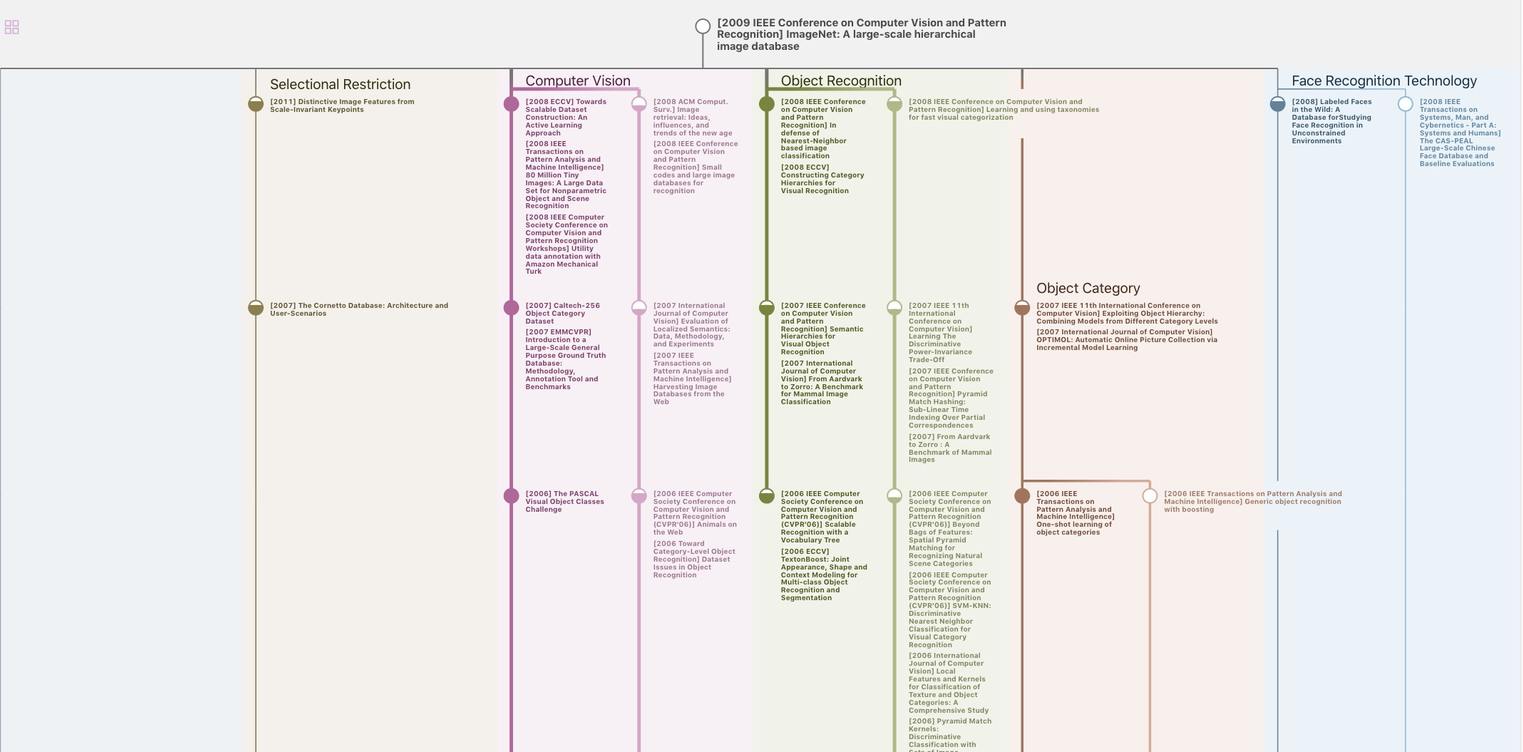
生成溯源树,研究论文发展脉络
Chat Paper
正在生成论文摘要