Hybrid Attention Transformer Based on Dual-Path for Time-Domain Single-Channel Speech Separation
2023 IEEE 6th International Conference on Pattern Recognition and Artificial Intelligence (PRAI)(2023)
摘要
Transformer allows each position to interact with all other positions in the input sequence, enabling powerful capturing of global interaction information. However, in speech separation tasks, fine-grained local information is crucial in speech sequences, and relying solely on self-attention mechanisms may not extract these local details information effectively. To address this limitation, this paper proposes a dual-path hybrid attention transformer network (DPHAT-Net) for time-domain single-channel speech separation. Specifically, the hybrid attention transformer (HA-Transformer) module is designed to capture global and local information in speech sequences. Furthermore, a Simple Recurrent Unit (SRU) is introduced to replace traditional positional encoding better to utilize the temporal position information in speech sequences. This paper conducts experimental evaluations on the WSJ0-2mix benchmark dataset and shows that the proposed DPHAT-Net realizes state-of-the-art speech separation performance while maintaining a relatively small model size.
更多查看译文
关键词
hybrid attention,speech separation,dual-path
AI 理解论文
溯源树
样例
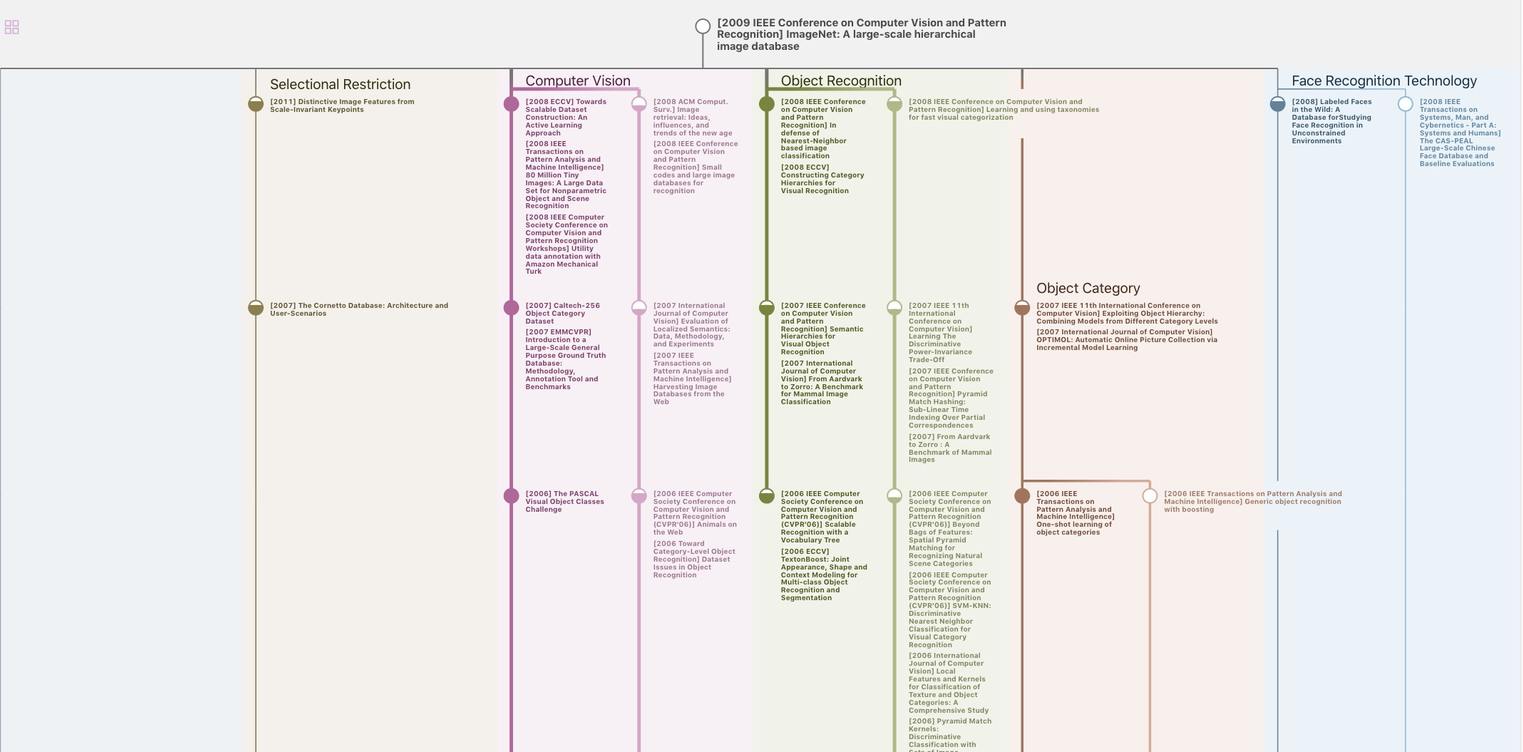
生成溯源树,研究论文发展脉络
Chat Paper
正在生成论文摘要