Acute Stress Classification with a Stroop task and in-office biophilic Relaxation in Virtual Reality based on Behavioral and Physiological data.
2023 IEEE International Symposium on Mixed and Augmented Reality Adjunct (ISMAR-Adjunct)(2023)
摘要
Acute stress in Virtual Reality (VR) requires more experimental inputs to determine how VR affects human psychophysiology, how to alleviate stress, and whether visual fatigue can impact its detection with machine learning. We randomly assigned 93 participants to three experimental conditions: Control, Stressful, and Stereoscopy. Participants fulfilled a rest-baseline period, a Stroop task, an STAI-6 questionnaire, and a relaxation period. We recorded behavioral and physiological data from eye-tracking (ET), electrocardiogram (ECG), and electrodermal activity (EDA). We identified three acute stress levels based on STAI-6 scores and behavioral data. We deployed linear models penalized with L1 constraints to select the most relevant features correlated with stress levels. The EDA sensor provided the most selected features compared to ECG and ET. We compared SVM, Logistic Regression, and Gradient boosting classifiers. The Logistic regression with ElasticNet constraint provides the best feature selection. The Gradient boosting method is the most performant Acute stress classifier, with 89.86% accuracy and an 89.8% F1 score.
更多查看译文
关键词
Virtual Reality,Stress,Physiology,Machine Learning,Machine Learning Human Factors
AI 理解论文
溯源树
样例
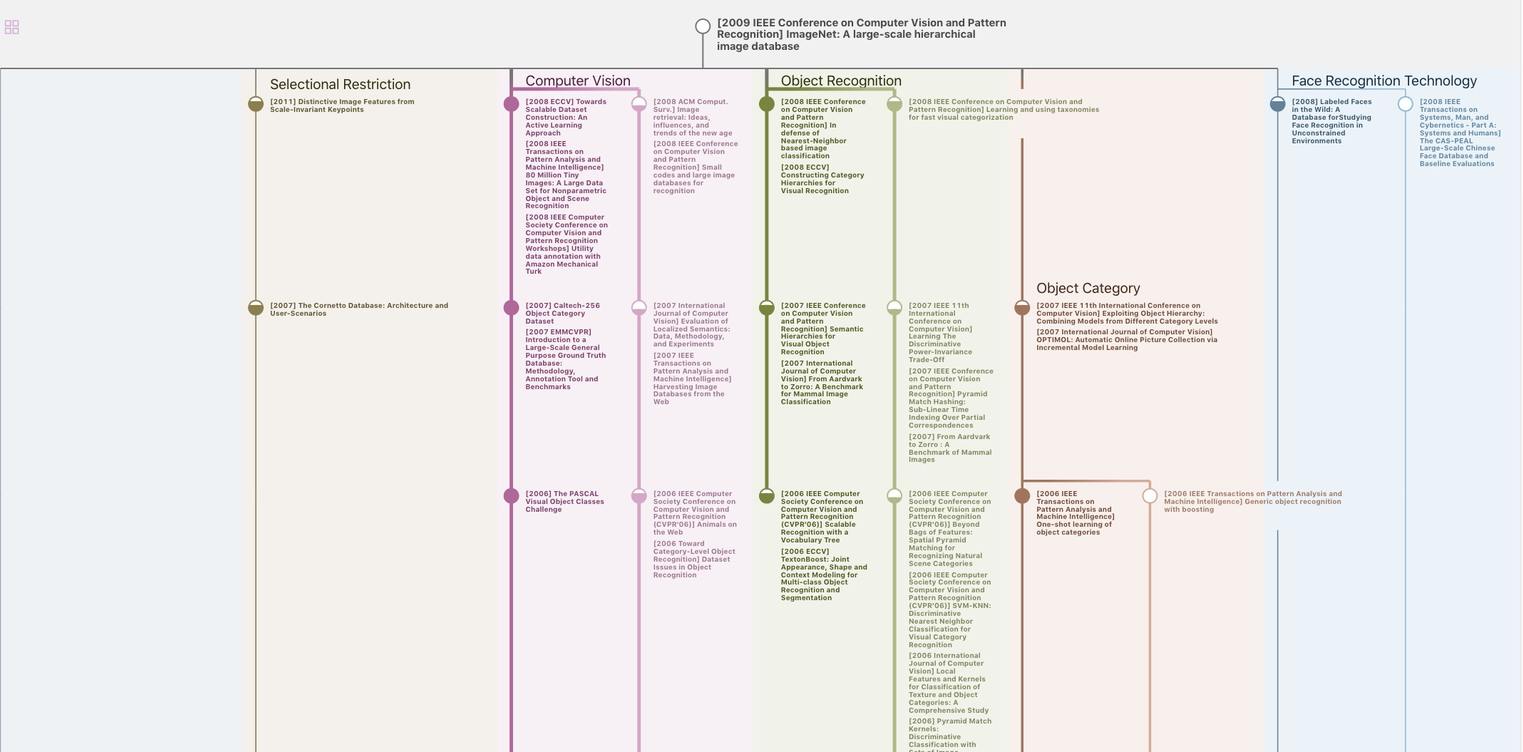
生成溯源树,研究论文发展脉络
Chat Paper
正在生成论文摘要