Combining CNN and Transformer for Noise-Robust Image Compressed Sensing
2023 IEEE 6th International Conference on Pattern Recognition and Artificial Intelligence (PRAI)(2023)
摘要
Compressed sensing (CS), as a signal acquisition and reconstruction method, benefits from deep learning technology and can quickly and accurately reconstruct signals from a small number of observations. Emerging Transformer networks bring significant gains to CS while also leaving unresolved issues. One obvious issue is that the reconstruction performance of CS methods drops sharply in the presence of noise. To achieve accurate CS reconstruction in noisy scenes, this paper proposes to improve the latest Transformer-based CS method with a CNN correction module, resulting in a joint scheme for noise-robust image CS recovery. The scheme adopts a combination of a deep learning reconstruction module and a denoising module. Specifically, it consists of a reconstruction module with a sampling subnet for a trainable perceptual matrix strategy, an initial reconstruction subnet, a hybrid reconstruction subnet, and a trainable denoising module. Extensive experiments show that the scheme can effectively improve the image quality and noise robustness of compressed sensing reconstruction.
更多查看译文
关键词
Compressed sensing,Deep learning,Transformer,Convolutional neural network (CNN),Noise-robust
AI 理解论文
溯源树
样例
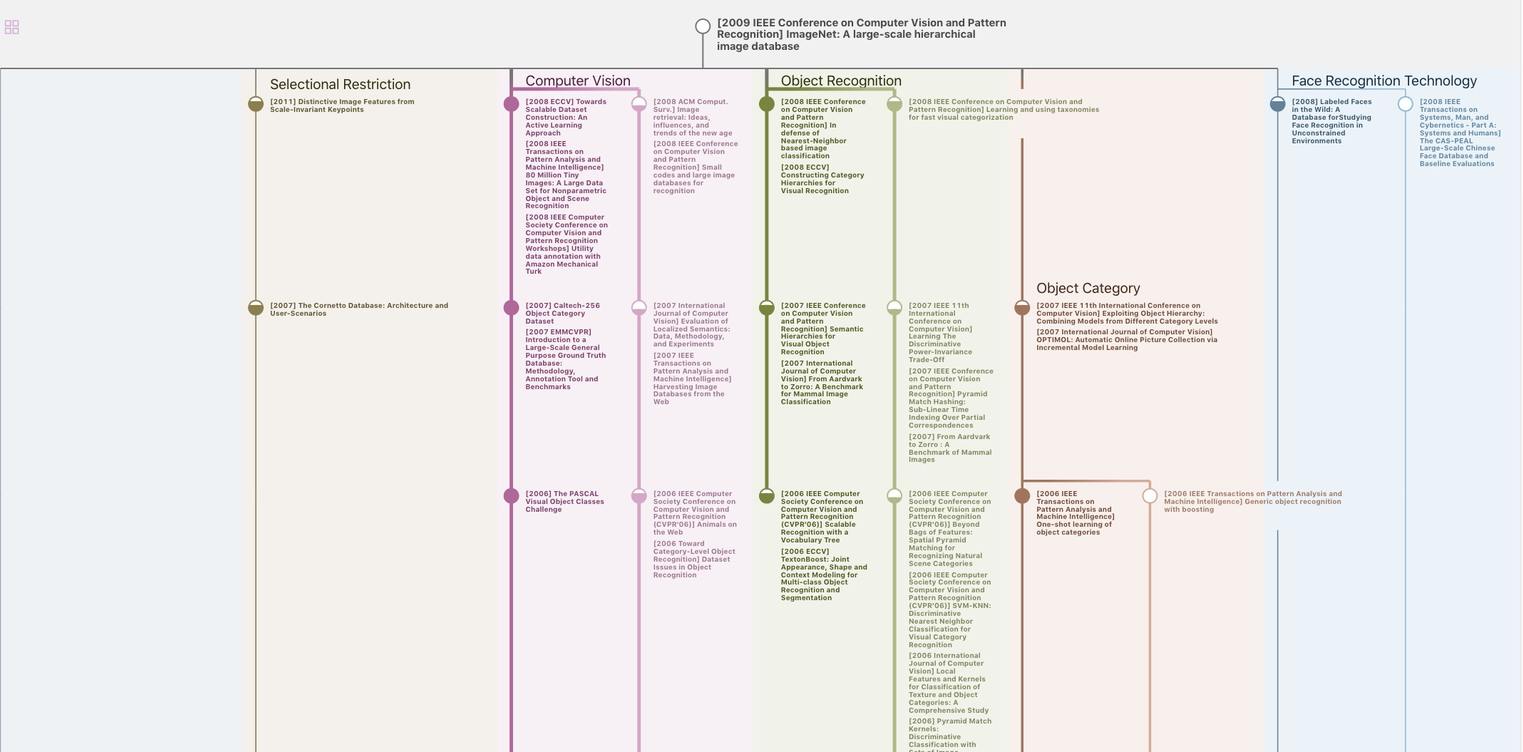
生成溯源树,研究论文发展脉络
Chat Paper
正在生成论文摘要