An Integrated Approach for Product Development using Latent Dirichlet Allocation and Gradient Boosting Decision Tree Methods.
2023 IEEE 6th International Conference on Knowledge Innovation and Invention (ICKII)(2023)
摘要
The COVID-19 pandemic brought immense strain on global manufacturing, highlighting the critical importance of the Customer-to-Manufacturer (C2M) model. To effectively manage customer needs and support decision-making in product development, there is a need for active research and the provision of additional tools. Thus, we collected and analyzed online sales data and user reviews to offer real-time insights into consumer needs. By using this data-driven recommendation system, product development directions can be identified to understand market demands, reduce the time required for research and development, and enhance overall product innovation efficiency. We also explored the effectiveness of employing the Latent Dirichlet Allocation (LDA) method and various machine learning technologies for predicting Amazon consumer ratings. Through the integration of structured and unstructured data, valuable industry insights were obtained, enabling businesses to understand consumer needs, discover market opportunities, and refine product specifications. Embracing the C2M model and incorporating consumer perspectives into decision-making processes were essential for sustainable business growth in the current economic landscape.
更多查看译文
关键词
C2M,Latent Dirichlet Allocation,machine learning,Product Development,Decision Support Systems
AI 理解论文
溯源树
样例
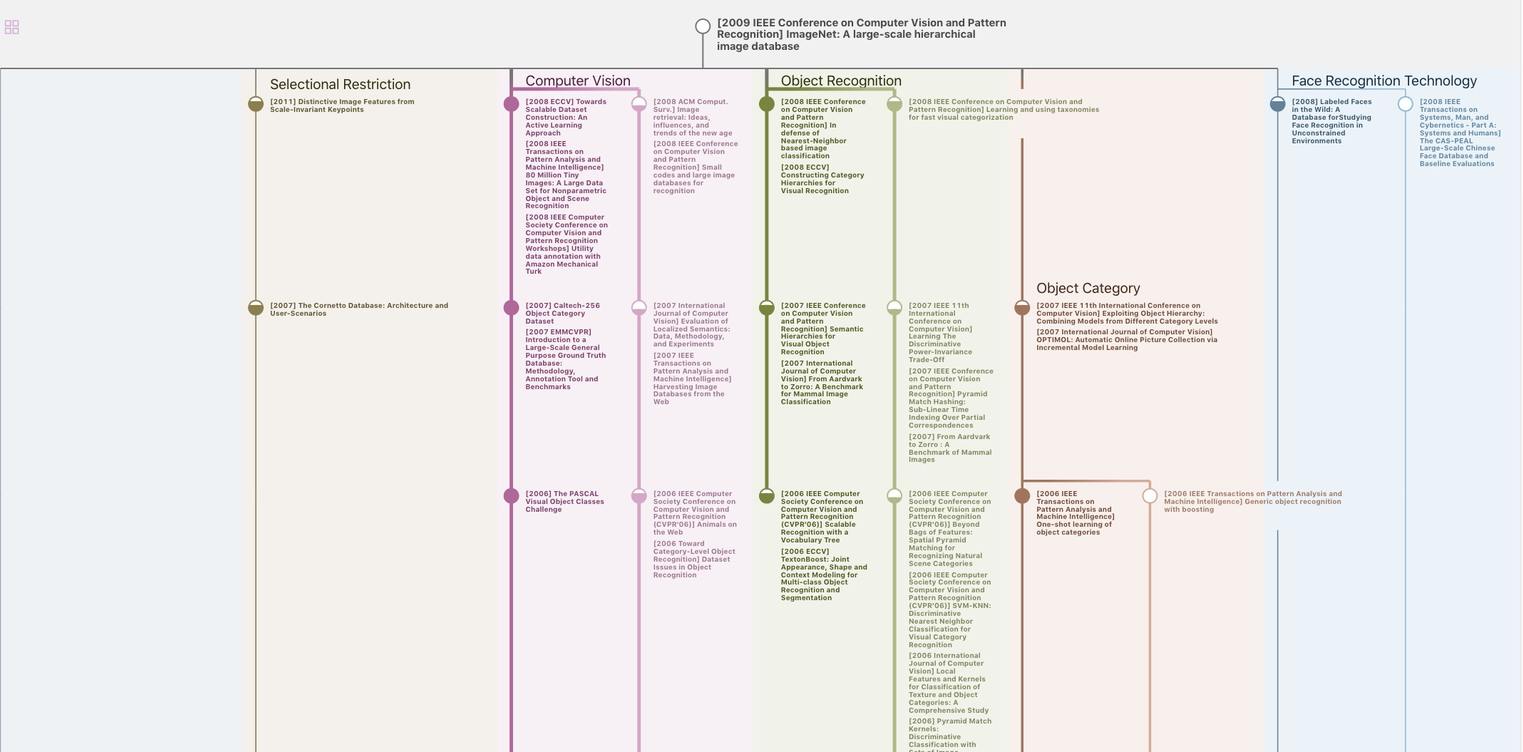
生成溯源树,研究论文发展脉络
Chat Paper
正在生成论文摘要