Close-Range Indoor Proximity Detection for COVID-19 Exposure Notifications Using Smartphone Magnetometer Traces.
2023 13th International Conference on Indoor Positioning and Indoor Navigation (IPIN)(2023)
摘要
Smartphone apps for exposure notification and contact tracing have been shown to be effective in controlling the COVID-19 pandemic. However, there is evidence that the Bluetooth Low Energy approach used for proximity detection by existing apps can be error-prone in areas with high numbers of metallic objects. In this paper, we present a new method for detecting whether or not two smartphones are 2 or fewer meters apart, intended to augment BLE-based proximity detection methods. We design a set of binary machine learning classifiers that take as input pairs of 10-second-long segments of magnetometer traces. These classifiers identify pairs of trace segments for which the two recording devices were 2 or fewer meters apart for at least 75% of the segment duration. We introduce a simple method of compensating for different magnetometer biases in heterogeneous devices. We confirm that our classifiers can generalize well to both new buildings and new devices whose traces are not present in their training data, and characterize their overall accuracy for heterogeneous-device proximity detection to be between 93.1% and 96.2%.
更多查看译文
关键词
proximity detection,contact tracing,magnetometer traces,machine learning,heterogeneous devices
AI 理解论文
溯源树
样例
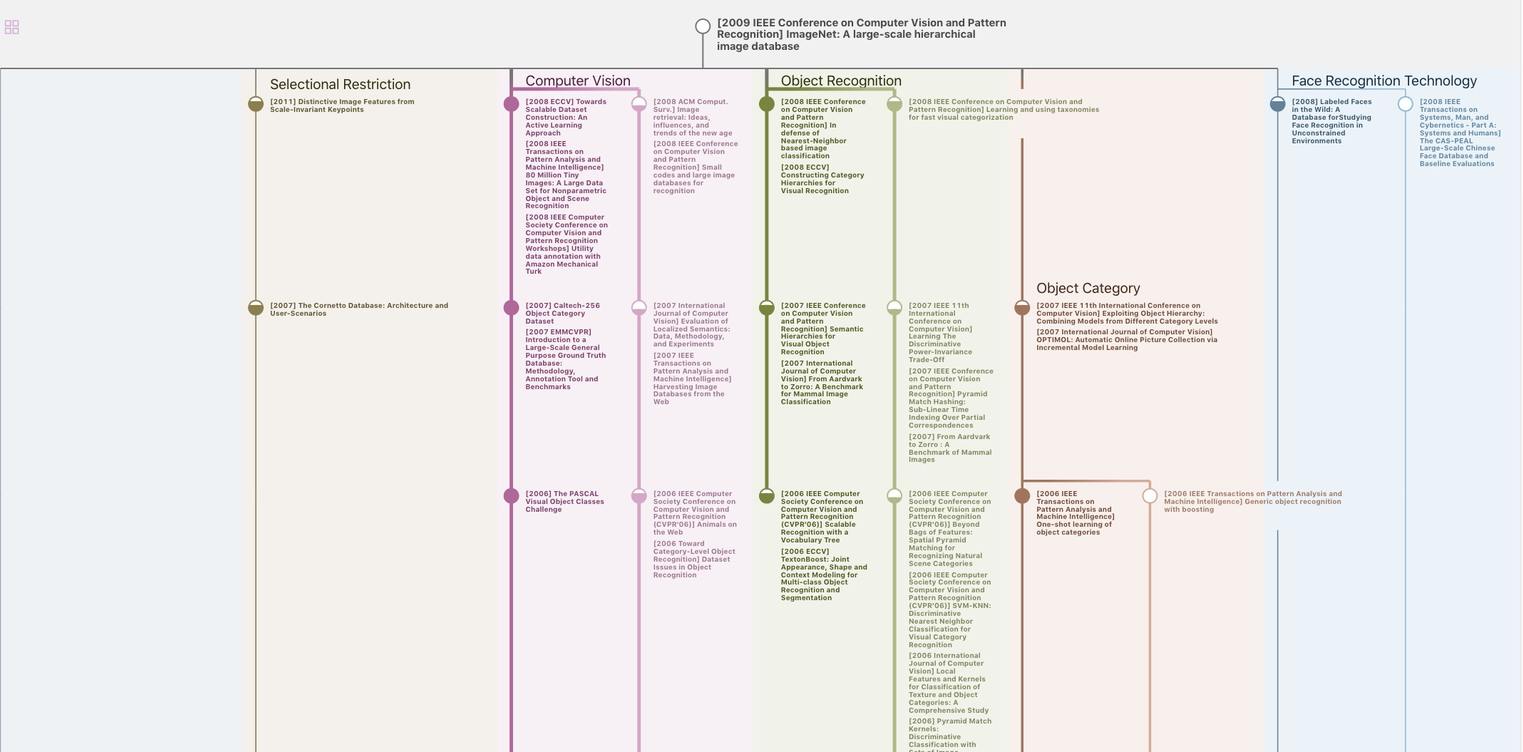
生成溯源树,研究论文发展脉络
Chat Paper
正在生成论文摘要