Normalization of RNA-Seq Data using Adaptive Trimmed Mean with Multi-reference
biorxiv(2023)
摘要
The normalization of RNA sequencing data is a primary step for downstream analysis. The most popular method used for the normalization is the trimmed mean of M values (TMM) and DESeq. The TMM tries to trim away extreme log fold changes of the data to normalize the raw read counts based on the remaining non-deferentially expressed genes. However, the major problem with the TMM is that the values of trimming factor M are heuristic. This paper tries to estimate the adaptive value of M in TMM based on Jaeckel Estimator, and each sample acts as a reference to find the scale factor of each sample. The presented approach is validated on SEQC, MAQC2, MAQC3, PICKRELL, and two simulated datasets with two groups and three groups conditions by varying the percentage of differential expression and the number of replicates. The performance of the present approach is compared, and it shows better in terms of area under the receiver operating characteristic curve (AUC) and differential expression. The implementation of the present approach is available on the GitHub platform: https://github.com/vikkyak/Normalization-of-Bulk-RNA-seq.
### Competing Interest Statement
The authors have declared no competing interest.
更多查看译文
AI 理解论文
溯源树
样例
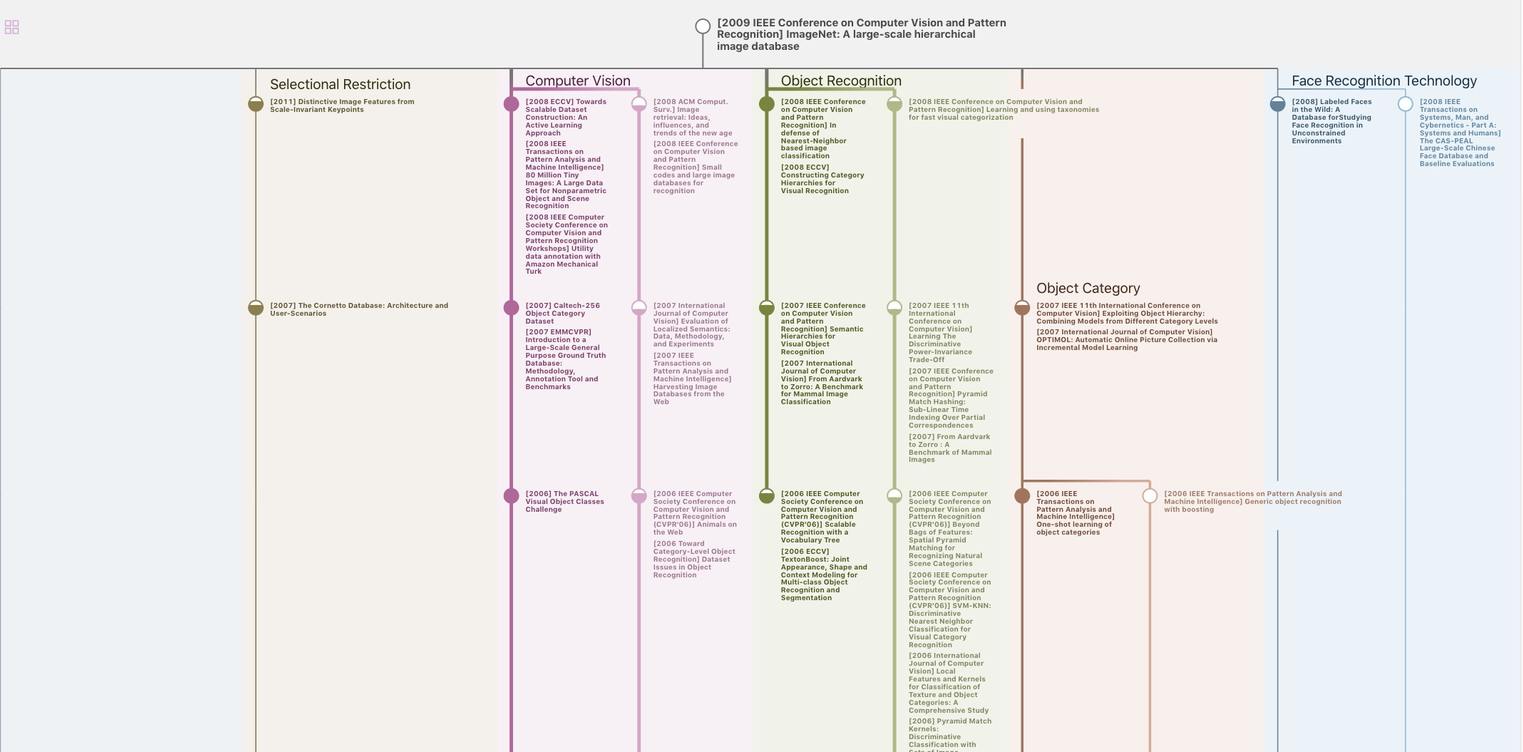
生成溯源树,研究论文发展脉络
Chat Paper
正在生成论文摘要