On Distributed Nonconvex Optimisation via Modified ADMM
IEEE CONTROL SYSTEMS LETTERS(2023)
摘要
This letter addresses the problem of nonconvex nonsmooth decentralised optimisation in multi-agent networks with undirected connected communication graphs. Our contribution lies in introducing an algorithmic framework designed for the distributed minimisation of the sum of a smooth (possibly nonconvex and non-separable) function and a convex (possibly nonsmooth and non-separable) regulariser. The proposed algorithm can be seen as a modified version of the ADMM algorithm where, at each step, an "inner loop" needs to be iterated for a number of iterations. The role of the inner loop is to aggregate and disseminate information across the network. We observe that a naive decentralised approach (one iteration of the inner loop) may not converge. We establish the asymptotic convergence of the proposed algorithm to the set of stationary points of the nonconvex problem where the number of iterations of the inner loop increases logarithmically with the step count of the ADMM algorithm. We present numerical results demonstrating the proposed method's correctness and performance.
更多查看译文
关键词
Optimization,Convex functions,Convergence,Approximation algorithms,Machine learning algorithms,Distributed algorithms,Australia,optimization algorithms,networked control systems
AI 理解论文
溯源树
样例
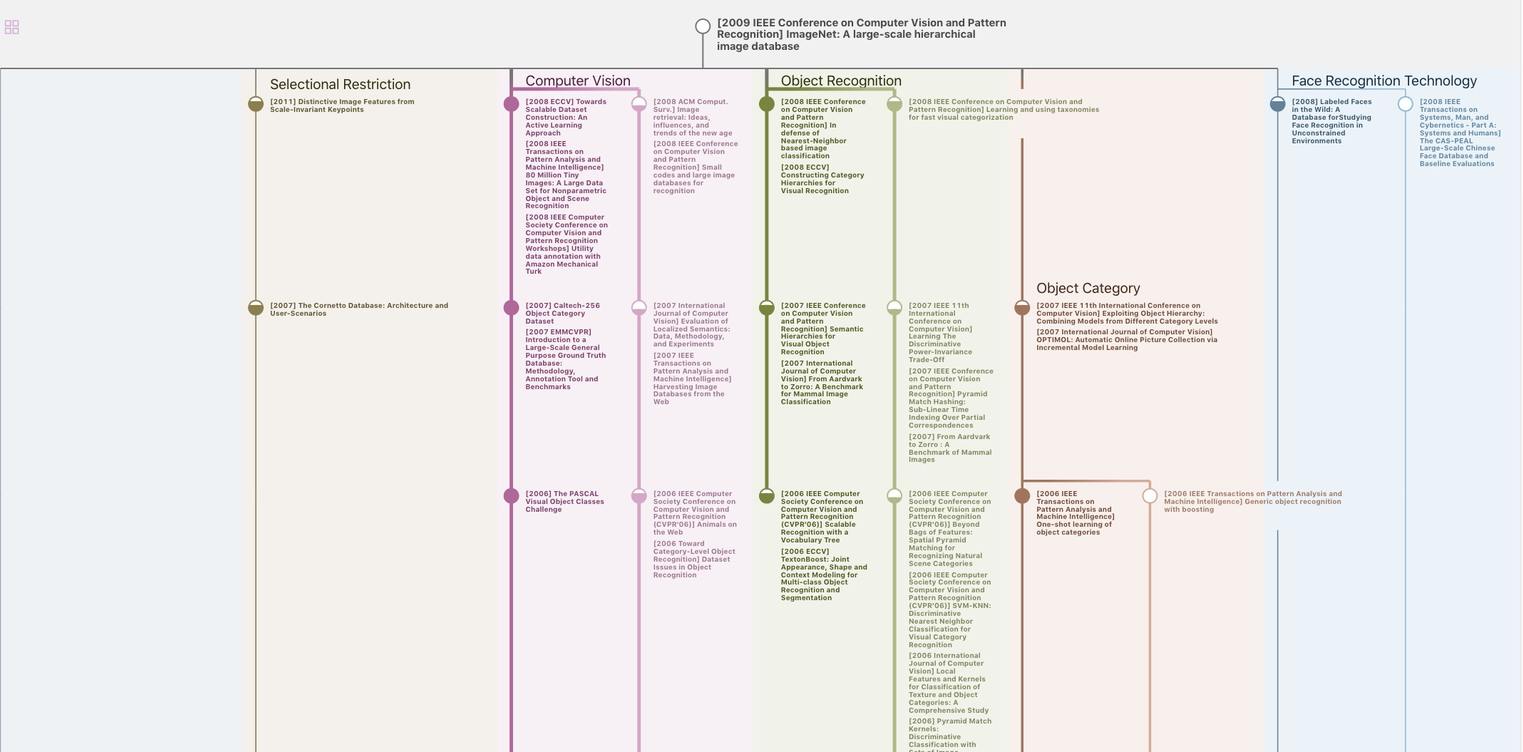
生成溯源树,研究论文发展脉络
Chat Paper
正在生成论文摘要