MICRO: Model-Based Offline Reinforcement Learning with a Conservative Bellman Operator
IJCAI 2024(2024)
摘要
Offline reinforcement learning (RL) faces a significant challenge ofdistribution shift. Model-free offline RL penalizes the Q value forout-of-distribution (OOD) data or constrains the policy closed to the behaviorpolicy to tackle this problem, but this inhibits the exploration of the OODregion. Model-based offline RL, which uses the trained environment model togenerate more OOD data and performs conservative policy optimization withinthat model, has become an effective method for this problem. However, thecurrent model-based algorithms rarely consider agent robustness whenincorporating conservatism into policy. Therefore, the new model-based offlinealgorithm with a conservative Bellman operator (MICRO) is proposed. This methodtrades off performance and robustness via introducing the robust Bellmanoperator into the algorithm. Compared with previous model-based algorithms withrobust adversarial models, MICRO can significantly reduce the computation costby only choosing the minimal Q value in the state uncertainty set. Extensiveexperiments demonstrate that MICRO outperforms prior RL algorithms in offlineRL benchmark and is considerably robust to adversarial perturbations.
更多查看译文
关键词
Machine Learning -> ML: Reinforcement learning,Machine Learning -> ML: Model-based and model learning reinforcement learning,Machine Learning -> ML: Offline reinforcement learning,Machine Learning -> ML: Robustness
AI 理解论文
溯源树
样例
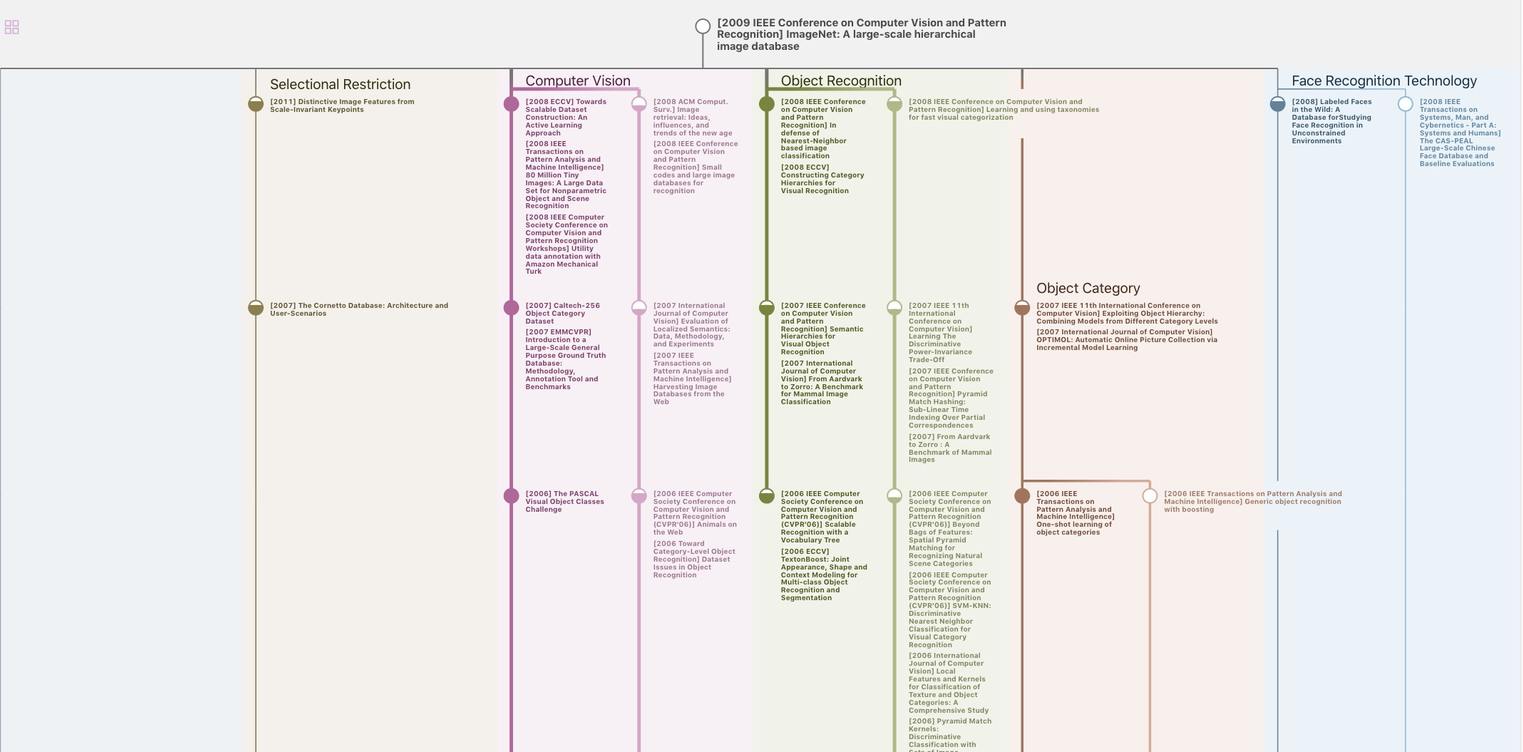
生成溯源树,研究论文发展脉络
Chat Paper
正在生成论文摘要