Guided Reconstruction with Conditioned Diffusion Models for Unsupervised Anomaly Detection in Brain MRIs
CoRR(2023)
摘要
Unsupervised anomaly detection in Brain MRIs aims to identify abnormalities
as outliers from a healthy training distribution. Reconstruction-based
approaches that use generative models to learn to reconstruct healthy brain
anatomy are commonly used for this task. Diffusion models are an emerging class
of deep generative models that show great potential regarding reconstruction
fidelity. However, they face challenges in preserving intensity characteristics
in the reconstructed images, limiting their performance in anomaly detection.
To address this challenge, we propose to condition the denoising mechanism of
diffusion models with additional information about the image to reconstruct
coming from a latent representation of the noise-free input image. This
conditioning enables high-fidelity reconstruction of healthy brain structures
while aligning local intensity characteristics of input-reconstruction pairs.
We evaluate our method's reconstruction quality, domain adaptation features and
finally segmentation performance on publicly available data sets with various
pathologies. Using our proposed conditioning mechanism we can reduce the
false-positive predictions and enable a more precise delineation of anomalies
which significantly enhances the anomaly detection performance compared to
established state-of-the-art approaches to unsupervised anomaly detection in
brain MRI. Furthermore, our approach shows promise in domain adaptation across
different MRI acquisitions and simulated contrasts, a crucial property of
general anomaly detection methods.
更多查看译文
AI 理解论文
溯源树
样例
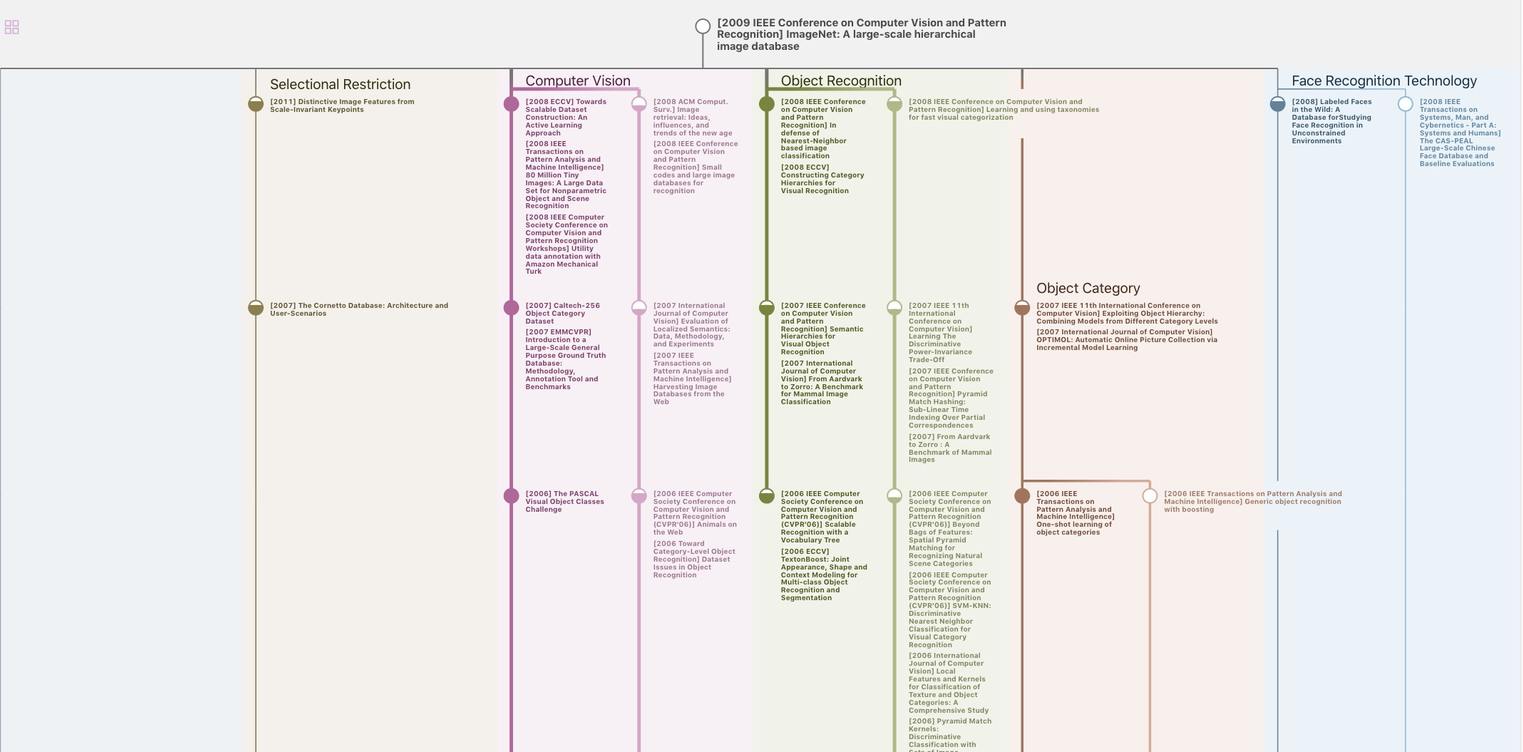
生成溯源树,研究论文发展脉络
Chat Paper
正在生成论文摘要